Feature Extraction Using Principal Component Analysis and Discrete Wavelet Transform for Image Classification
B. Kumar1
Section:Research Paper, Product Type: Journal Paper
Volume-6 ,
Issue-8 , Page no. 582-586, Aug-2018
CrossRef-DOI: https://doi.org/10.26438/ijcse/v6i8.582586
Online published on Aug 31, 2018
Copyright © B. Kumar . This is an open access article distributed under the Creative Commons Attribution License, which permits unrestricted use, distribution, and reproduction in any medium, provided the original work is properly cited.
View this paper at Google Scholar | DPI Digital Library
How to Cite this Paper
- IEEE Citation
- MLA Citation
- APA Citation
- BibTex Citation
- RIS Citation
IEEE Citation
IEEE Style Citation: B. Kumar, “Feature Extraction Using Principal Component Analysis and Discrete Wavelet Transform for Image Classification,” International Journal of Computer Sciences and Engineering, Vol.6, Issue.8, pp.582-586, 2018.
MLA Citation
MLA Style Citation: B. Kumar "Feature Extraction Using Principal Component Analysis and Discrete Wavelet Transform for Image Classification." International Journal of Computer Sciences and Engineering 6.8 (2018): 582-586.
APA Citation
APA Style Citation: B. Kumar, (2018). Feature Extraction Using Principal Component Analysis and Discrete Wavelet Transform for Image Classification. International Journal of Computer Sciences and Engineering, 6(8), 582-586.
BibTex Citation
BibTex Style Citation:
@article{Kumar_2018,
author = {B. Kumar},
title = {Feature Extraction Using Principal Component Analysis and Discrete Wavelet Transform for Image Classification},
journal = {International Journal of Computer Sciences and Engineering},
issue_date = {8 2018},
volume = {6},
Issue = {8},
month = {8},
year = {2018},
issn = {2347-2693},
pages = {582-586},
url = {https://www.ijcseonline.org/full_paper_view.php?paper_id=2737},
doi = {https://doi.org/10.26438/ijcse/v6i8.582586}
publisher = {IJCSE, Indore, INDIA},
}
RIS Citation
RIS Style Citation:
TY - JOUR
DO = {https://doi.org/10.26438/ijcse/v6i8.582586}
UR - https://www.ijcseonline.org/full_paper_view.php?paper_id=2737
TI - Feature Extraction Using Principal Component Analysis and Discrete Wavelet Transform for Image Classification
T2 - International Journal of Computer Sciences and Engineering
AU - B. Kumar
PY - 2018
DA - 2018/08/31
PB - IJCSE, Indore, INDIA
SP - 582-586
IS - 8
VL - 6
SN - 2347-2693
ER -
![]() |
![]() |
![]() |
368 | 292 downloads | 158 downloads |
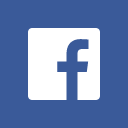
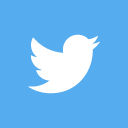
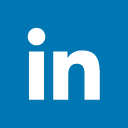
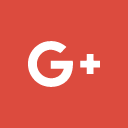
Abstract
Feature extraction is an important part of any image classification scheme. It provides more informative and compact values derived from the original data. In this paper two conventional and widely used techniques known as principal component analysis (PCA) and discrete wavelet transform (DWT) are used for feature extraction. Both techniques are based on entirely different approaches. The results for the two techniques are analyzed and compared. The classification is performed with a benchmark classifier support vector machine. The experiments are carried out on a publically available datasets. The results have shown that DWT has performed better than PCA under the tested scenario.
Key-Words / Index Term
Classification, Feature extraction, Discrete Wavelet Transform (DWT), Principal Component Analysis (PCA)
References
[1] G. Mountrakis, J. Im, and C. Ogole, “Support vector machines in remote sensing: A review,” ISPRS Journal of Photogrammetry and Remote Sensing, vol. 66, pp. 247–259, 2011.
[2] L.M. Bruce, C.H. Koger, and J. Li, “Dimensionality reduction of hyperspectral data using discrete wavelet transform feature extraction,” IEEE Transactions on Geoscience and Remote Sensing, vol. 40, no. 10, pp. 2331-2338, 2002.
[3] Joliffe, I., “Principal Component Analysis,” Springer-Verlag, New York, 2002.
[4] M. Khalil, H. Ayad, and A. Adib, “Performance evaluation of feature extraction techniques in MR-Brain image classification system,” Procedia Computer Science, vol. 127, pp. 218-225, 2018.
[5] Ş. Öztürk and B. Akdemir, “Application of feature extraction and classification methods for histopathological image using GLCM, LBP, LBGLCM, GLRLM and SFTA,” Procedia Computer Science, vol. 132, pp. 40-46, 2018.
[6] J. Yue, Z. Li, L. Liu, and Z. Fu, “Content-based image retrieval using color and texture fused features,” Mathematical and Computer Modelling, vol. 54, pp. 1121-1127, 2011.
[7] R. Das, S. Thepade, S. Bhattacharya, and S. Ghosh, “Retrieval architecture with classified query for content based image recognition,” Applied Computional Intelligence and Soft Computing,, vol. 2016, pp. 1-9, 2016.
[8] A. Boukharouba and A. Bennia, “Novel feature extraction technique for the recognition of handwritten digits,” Applied Computing and Informatics, vol. 13, pp. 19-26, 2015.
[9] A. Bala and T. Kaur, “Local texton XOR patterns: A new feature descriptor for content based image retrieval,” Engineering Science and Technology, An International Journal, vol. 19, pp. 101-112, 2016.
[10] L. Guo, M. Dai, and M. Zhu, “Quaternion moment and its invriants for color object classification,” Information Science, vol. 273, pp. 132-143, 2014.
[11] Y. Qian, M. Ye, and J. Zhou, “Hyperspectral image classification based on structured sparse logistic regression and three-dimensional wavelet texture features,” IEEE Transactions On Geoscience And Remote Sensing, vol. 51, no. 4, pp. 2276-2291, 2012.
[12] J. Li, “Wavelet-based feature extraction for improved endmember abundance estimation in linear unmixing of hyperspectral signals,” IEEE Transactions On Geoscience And Remote Sensing, vol. 42, no. 3, pp. 644-649, 2004.
[13] L. M. Bruce, C. H. Koger, and J. Li, “Dimensionality reduction of hyperspectral data using discrete wavelet transform feature extraction,” IEEE Transactions nn Geoscience And Remote Sensing, vol. 40, no. 10, pp. 2331-2338, 2002.
[14] Chang, C.-C., and Lin, C.-J., “LIBSVM: a library for support vector machines,” ACM Transactions on Intelligent Systems and Technology, vol. 2, no. 3, pp. 27:1-27:27, 2011.