Evaluation and Performance Analysis of Brain MRI Segmentation Methods
Naresh Ghorpade1 , H. R. Bhapkar2
Section:Research Paper, Product Type: Journal Paper
Volume-6 ,
Issue-8 , Page no. 687-696, Aug-2018
CrossRef-DOI: https://doi.org/10.26438/ijcse/v6i8.687696
Online published on Aug 31, 2018
Copyright © Naresh Ghorpade, H. R. Bhapkar . This is an open access article distributed under the Creative Commons Attribution License, which permits unrestricted use, distribution, and reproduction in any medium, provided the original work is properly cited.
View this paper at Google Scholar | DPI Digital Library
How to Cite this Paper
- IEEE Citation
- MLA Citation
- APA Citation
- BibTex Citation
- RIS Citation
IEEE Citation
IEEE Style Citation: Naresh Ghorpade, H. R. Bhapkar, “Evaluation and Performance Analysis of Brain MRI Segmentation Methods,” International Journal of Computer Sciences and Engineering, Vol.6, Issue.8, pp.687-696, 2018.
MLA Citation
MLA Style Citation: Naresh Ghorpade, H. R. Bhapkar "Evaluation and Performance Analysis of Brain MRI Segmentation Methods." International Journal of Computer Sciences and Engineering 6.8 (2018): 687-696.
APA Citation
APA Style Citation: Naresh Ghorpade, H. R. Bhapkar, (2018). Evaluation and Performance Analysis of Brain MRI Segmentation Methods. International Journal of Computer Sciences and Engineering, 6(8), 687-696.
BibTex Citation
BibTex Style Citation:
@article{Ghorpade_2018,
author = {Naresh Ghorpade, H. R. Bhapkar},
title = {Evaluation and Performance Analysis of Brain MRI Segmentation Methods},
journal = {International Journal of Computer Sciences and Engineering},
issue_date = {8 2018},
volume = {6},
Issue = {8},
month = {8},
year = {2018},
issn = {2347-2693},
pages = {687-696},
url = {https://www.ijcseonline.org/full_paper_view.php?paper_id=2756},
doi = {https://doi.org/10.26438/ijcse/v6i8.687696}
publisher = {IJCSE, Indore, INDIA},
}
RIS Citation
RIS Style Citation:
TY - JOUR
DO = {https://doi.org/10.26438/ijcse/v6i8.687696}
UR - https://www.ijcseonline.org/full_paper_view.php?paper_id=2756
TI - Evaluation and Performance Analysis of Brain MRI Segmentation Methods
T2 - International Journal of Computer Sciences and Engineering
AU - Naresh Ghorpade, H. R. Bhapkar
PY - 2018
DA - 2018/08/31
PB - IJCSE, Indore, INDIA
SP - 687-696
IS - 8
VL - 6
SN - 2347-2693
ER -
![]() |
![]() |
![]() |
565 | 316 downloads | 165 downloads |
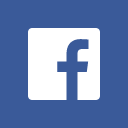
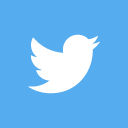
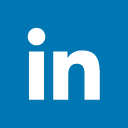
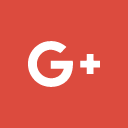
Abstract
Image segmentation is very important in computer vision for image recovery, visual summary, image base modeling, and for many other purposes. Despite many years of research and substantial contributions, image segmentation is still a very challenging task to suit for range of applications. Brain Magnetic Resonance Image (MRI) segmentation is one of the most challenging and time consuming task in the field of medical imaging. But by nature medical images are complex and noisy. This leads to the necessity of processes that reduces difficulties in analysis and improves quality of output. Even though several methods and encouraging results are obtained in medical imaging area, reproducible segmentation and grouping of abnormalities are still a thought provoking task due to the different shapes, locations and image intensities of different types of tumors. This paper critically reviews recent brain MRI segmentation methods along with their detailed analysis, and evaluation on the basis of various parameters. The study and evaluation is useful in improving the performance of existing methods as well as helpful in the development of new methods.
Key-Words / Index Term
Image Segmentation, Brain MRI, Graph Cuts
References
[1]. Acton, S.T., Mukherjee, “Scale space classification using area morphology,” IEEE Trans Image Process 9(4):623–635, 2000.
[2]. Ahmed, M.N., Yamany, S.M., Mohamed, N. “A modified fuzzy c-means algorithm for bias field estimation and segmentation of MRI data,” IEEE transaction medical imaging, Vol.21, No.3, pp.193-199, 2002.
[3]. Abdullah, N., Ngah, U.K., Aziz, “Image Classification of brain MRI using Support Vector Machine,” Imaging systems and Techniques (IST), IEEE International conference on 17-18 May 2011.
[4]. J. Tou and R. Gonzalez, Pattern Recognition Principles. Addison-Wesley, 1974.
[5]. C. C. Hung, “Competitive learning networks for unsupervised training,” Int. J. of Remote Sensing, vol. 14, no. 12, pp. 2411-2415, 1993.
[6]. S. Nagabhushana, “Computer Vision and Image Processing,” New Age International Publishers, 2005.
[7]. W. Hongzhi and D. Ying, “An Improved Image Segmentation Algorithm Based on Otsu Method,” International Symposium on Photo electronic Detection and Imaging 2007: Related Technologies and Applications, Vol. 6625, 2008.
[8]. Nobuyuki Otsu, “A Threshold Selection Method From Gray-Level Histograms,” IEEE Transactions on Systems, Man, And Cybernetics, Vol. 9, No. 1, 1979.
[9]. Tranos Zuva, Oludayo O. Olugbara, Sunday O. Ojo and Seleman M. Ngwira, “Image Segmentation, Available Techniques, Developments and Open Issues,” Canadian Journal on Image Processing and Computer Vision, Vol. 2, No. 3, 2011.
[10]. Bhattacharyya, D., Tai-hoon Kim, "Brain Tumor Detection Using MRI Image Analysis", Communications in Computer and Information Science, Vol: 151, pp. 307-314, 2011.
[11]. Bryan S. Morse, Lecture 18: Segmentation (Region Based), Brigham Young University, 1998.
[12]. H.S.Prasantha, H. Shashidhara, and Madhavi Lata, “MEDICAL IMAGE SEGMENTATION”, (IJCSE) International Journal on Computer Science and Engineering Vol. 02, No. 04, 2010.
[13]. Dzung L. Pham, Chenyang Xu, and Jerry L. Prince, “Current Methods in Medical Image Segmentation,” Annu. Rev. Biomed. Eng., Vol. 02 pp. 315–37. 23, 2000.
[14]. Rolf Adams and Leanne Bischof, “Seeded Region Growing,” IEEE Transactions on Pattern Analysis and Machine Intelligence, Vol.16, No. 6, 1994.
[15]. Bo Peng, Lei Zhang, and David Zhang, “A Survey of Graph Theoretical Approaches to Image Segmentation,” IEEE Transactions on Image Processing, Vol. 7, No. 12, December 2001.
[16]. Koley, S., Majumder, "Brain MRI segmentation for tumor detection using cohesion based self-merging algorithm", Proceedings of the IEEE 3rd International Conference on Communication Software and Networks (ICCSN), Xi`an, pp: 781 – 785, 2017.
[17]. Ravi S and A M Khan, “Operators Used in Edge Detection: A Case Study,” International Journal of Applied Engineering Research, Vol. 7 No. 11, 2012.
[18]. N. Senthil kumaran and R. Rajesh, “Edge Detection Techniques for Image Segmentation – A Survey of Soft Computing Approaches,” International Journal of Recent Trends in Engineering, Vol. 1, No. 2, 2009.
[19]. http://www.cs.uu.nl/docs/vakken/ibv/reader/chapter10.pdf.
[20]. K.Duraiswamy and V. Valli Mayil “Similarity Matrix Based Session Clustering by Sequence Alignment Using Dynamic Programming,” Computer and Information Science journal, Vol. 1, No.3, 2008.
[21]. Qurat-ul Ain., Irfan Mehmood., Naqi, M. Syed., Arfan Jaffar, M "Bayesian Classification Using DCT Features for Brain Tumor Detection", Lecture Notes in Computer Science, Vol:6276, pp. 340-349, 2016.
[22]. Rogowska, J (2009): “Overview and Fundamentals of medical image segmentation,” Handbook of medical imaging: Processing and Analysis Management, I.Bankman, Ed.pp.69-85, 2009.
[23]. F. Benezit, T. Cour, and J. Shi, “Spectral segmentation with multi-scale graph decomposition,” in Proc. IEEE Computer Society Conference on Computer Vision and Pattern Recognition, vol.2, pp.1124-1131, 2005.
[24]. S. D. Kapade, S. M. Khairnar, B. S. Chaudhari, “Enhanced Graph based Normalized cut Methods for Image Segmentation,” ICTACT Journal on Image and Video Processing, vol. 5, no. 2, pp. 907 - 911, November 2014.
[25]. G. Csurka, C. Bray, and L. Fan, “Visual categorization with bags of key points,” Conf. on Statistical Learning in Computer Vision, 2004.
[26]. R. Fergus, P. Perona, and A. Zisserman, “Object class recognition by unsupervised scale - invariant learning,” in Proc. of the IEEE Conf on Computer Vision and Pattern Recognition, 2003
[27]. S. Yu and J. Shi, “Object-specific figure-ground segregation,” in Proc. IEEE Computer Society Conf on Computer Vision and Pattern Recognition, vol.2, pp. 39-45, 2003.
[28]. Shi and J. Malik, “Normalized Cuts and Image Segmentation,” IEEE Trans. on Pattern Analysis and Machine Intelligence, vol.22, no.8, pp. 888-905, 2000.
[29]. I. J. Cox, S.B. Rao, and Y. Zhong, “Ratio Regions: a technique for image segmentation,” in Proc. of the Int. Conf. on Pattern Recognition, pp. 557-564, 1996.
[30]. A.X. Falcao, J.K. Udupa, and F.K. Miyazawa, “An ultra-fast user-steered Image segmentation paradigm: live wire on the fly,” IEEE Trans. on Medical Imaging, vol.19, no.1, pp. 55-62, 2000.
[31]. X. Bai and G. Sapiro, “A geodesic framework for fast interactive image and video segmentation and matting,” in Proc. of Int. Conf. on Computer Vision, pp.1-8, 2007.
[32]. L. Grady, “Minimal surfaces extend shortest path segmentation methods to 3D,” IEEE Trans. on Pattern Analysis and Machine Intelligence, pp. 321-334, vol.32, no.2, 2010.
[33]. V. Ramos V. and F. Almeida, “Artificial ant colonies in digital image habitats - a mass behaviours effect study on pattern recognition,” in Proc. Second Int. Workshop on Ant Algorithms, pp. 113-116, 2000
[34]. H. Nezamabadi, S. Saryazdi, and E. Rashedi, “Edge detection using ant algorithms,” Soft Computing, vol.10, pp. 623-628, 2006.
[35]. Aydın, “A modified ant-based approach to edge detection,” ICCCI – LNAI, vol. 5796, pp. 620 – 628, 2009.
[36]. Badran, E.F., Mahmoud, E.G., Hamdy, "An algorithm for detecting brain tumors in MRI images", Proceedings of the International Conference on Computer Engineering and Systems (ICCES), Cairo, pp: 368 – 373, 2010.
[37]. Chandra, S., Bhat, R., Singh, "A PSO based method for detection of brain tumors from MRI", Proceedings of the World Congress on Nature & Biologically Inspired Computing, Coimbatore, pp: 666 – 671, 2009.
[38]. S. D. Kapade, S. M. Khairnar, B. S. Chaudhari, “Image Segmentation using min- cut and swarm intelligence,” International Journal of mathematical Sciences and Engineering Applications, vol. 9, no. I, pp. 165 - 177, March 2015.