An Efficient Tweeter Sentiment Analysis Sfcetr: Selective Feature Based Case Content Extraction Using Maximum Entropy Classifier To Rank The Tweets
P. Kavitha1 , M. Prabakaran2
Section:Research Paper, Product Type: Journal Paper
Volume-6 ,
Issue-9 , Page no. 289-299, Sep-2018
CrossRef-DOI: https://doi.org/10.26438/ijcse/v6i9.289299
Online published on Sep 30, 2018
Copyright © P. Kavitha, M. Prabakaran . This is an open access article distributed under the Creative Commons Attribution License, which permits unrestricted use, distribution, and reproduction in any medium, provided the original work is properly cited.
View this paper at Google Scholar | DPI Digital Library
How to Cite this Paper
- IEEE Citation
- MLA Citation
- APA Citation
- BibTex Citation
- RIS Citation
IEEE Citation
IEEE Style Citation: P. Kavitha, M. Prabakaran, “An Efficient Tweeter Sentiment Analysis Sfcetr: Selective Feature Based Case Content Extraction Using Maximum Entropy Classifier To Rank The Tweets,” International Journal of Computer Sciences and Engineering, Vol.6, Issue.9, pp.289-299, 2018.
MLA Citation
MLA Style Citation: P. Kavitha, M. Prabakaran "An Efficient Tweeter Sentiment Analysis Sfcetr: Selective Feature Based Case Content Extraction Using Maximum Entropy Classifier To Rank The Tweets." International Journal of Computer Sciences and Engineering 6.9 (2018): 289-299.
APA Citation
APA Style Citation: P. Kavitha, M. Prabakaran, (2018). An Efficient Tweeter Sentiment Analysis Sfcetr: Selective Feature Based Case Content Extraction Using Maximum Entropy Classifier To Rank The Tweets. International Journal of Computer Sciences and Engineering, 6(9), 289-299.
BibTex Citation
BibTex Style Citation:
@article{Kavitha_2018,
author = {P. Kavitha, M. Prabakaran},
title = {An Efficient Tweeter Sentiment Analysis Sfcetr: Selective Feature Based Case Content Extraction Using Maximum Entropy Classifier To Rank The Tweets},
journal = {International Journal of Computer Sciences and Engineering},
issue_date = {9 2018},
volume = {6},
Issue = {9},
month = {9},
year = {2018},
issn = {2347-2693},
pages = {289-299},
url = {https://www.ijcseonline.org/full_paper_view.php?paper_id=2862},
doi = {https://doi.org/10.26438/ijcse/v6i9.289299}
publisher = {IJCSE, Indore, INDIA},
}
RIS Citation
RIS Style Citation:
TY - JOUR
DO = {https://doi.org/10.26438/ijcse/v6i9.289299}
UR - https://www.ijcseonline.org/full_paper_view.php?paper_id=2862
TI - An Efficient Tweeter Sentiment Analysis Sfcetr: Selective Feature Based Case Content Extraction Using Maximum Entropy Classifier To Rank The Tweets
T2 - International Journal of Computer Sciences and Engineering
AU - P. Kavitha, M. Prabakaran
PY - 2018
DA - 2018/09/30
PB - IJCSE, Indore, INDIA
SP - 289-299
IS - 9
VL - 6
SN - 2347-2693
ER -
![]() |
![]() |
![]() |
636 | 399 downloads | 242 downloads |
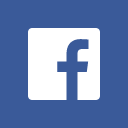
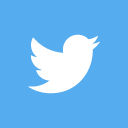
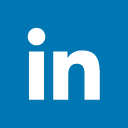
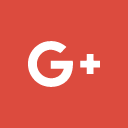
Abstract
Real world analysis the data based on the realistic approach to deal any objectives in online environment. Specifically social remedies have various approach of projective comments to share the information about products, innovative technologies etc. in this thing tweeter is a main platform to provide communication mammon the sharable users. In this provision most used by the people have the onions to make sentimental key terms to notify the originality. The sentiments about specific data be pointed by the comments in content format with sort text opinions. The opinions are extracted from the comments statement to analyst the tweeter data. By the fact of analyzing tweets have the hidden sentimental approach the problem arise due to right choice of sentimental extraction to classification is difficult. To overcome the problematic issue to propose a selective feature based case content extraction using maximum entropy classifier (SFCETR) to rank the tweets. This initially preprocess the tweets data the content reason from the comments statement. to aim the case reasons of relational features observed from the tweet contents are key term as contents .the comment case sentimental relation keyword terms are extracted synonmically to classify the data base on the reference key variable . Finally the rank case resultant categorize the sentimental case reasoning onion s about the predicative approach are classifies as class. This improves the tweets case opinions extraction are carried with the high performance sentimental research.
Key-Words / Index Term
Opinion mining, rank analysis, tweeter analysis, sentiment classification, features election
References
[1]. Popescu, A. M., Etzioni, O, Extracting Product Features and Opinions from Reviews, In Proc. Conf. Human Language Technology and Empirical Methods in Natural Language Processing, Vancouver, British Columbia, 2005.
[2]. A.H.Huang, D.C. Yen, & X. Zhang, “Exploring the effects of emoticons,” Information & Management, 45(7), 466–473, 2008.
[3]. J. Spencer and G. Uchyigit, “Sentiment or: Sentiment Analysis of Twitter Data,” Second Joint Conference on Lexicon and Computational Semantics. Brighton:University of Brighton, 2008.
[4]. M. Annett, and G. Kondrak, “A Comparison of Sentiment Analysis Techniques: Polarizing Movie Blogs,” Conference on web search and web data mining (WSDM). University of Alberia: Department of Computing Science, 2009
[5]. Sang-Hyun Cho and Hang-Bong Kang, “Text Sentiment Classification for SNS-based Marketing Using Domain Sentiment Dictionary”, IEEE International Conference on Conference on consumer Electronics (ICCE), p.717-718, 2012.
[6]. Z. Kechaou, B. M. Ammar and A. M. Alimi, “Improving e-learning with sentiment analysis of users` opinions”, in Global Engineering Education Conference (EDUCON), pp. 1032-1038.2011.
[7]. A. Celikyilmaz, D. Hakkani-Tur and Junlan Feng, “Probabilistic modelbased sentiment analysis of twitter messages”, IEEE Spoken Language Technology Workshop (SLT), pp. 79-84.2010
[8]. A. Pak,and P. Paroubek, “Twitter as a Corpus for Sentiment Analysis and Opinion Mining,” Special Issue of International Journal of Computer Application, France:Universitede Paris-Sud, 2010.
[9]. S.Lohmann, M. Burch, H. Schmauder and D. Weiskopf, “Visual Analysis of Microblog Content Using Time-Varying Co-occurrence Highlighting in Tag Clouds,” Annual conference of VISVISUS. Germany: University of Stuttgart, 2012.
[10]. R. Batool, A. M. Khattak, J. Maqbool and S. Lee, “Precise tweet classification and sentiment analysis”, in 12th Int. Conf. on Computer and Information Science (ICIS),pp. 461-466.2013.
[11]. G.Vinodini and RM.Chandrashekaran, “Sentiment Analysis and Opinion Mining: A Survey”, International Journal of Advanced Research in Computer Science and Software Engineering, 283-294, Volume 2, Issue 6, June 2012.
[12]. A.Pak and P. Paroubek. „Twitter as a Corpus for Sentiment Analysis and Opinion Mining". In Proceedings of the Seventh Conference on International Language Resources and Evaluation, pp.1320-1326.1010
[13]. Po-Wei Liang, Bi-Ru Dai, “Opinion Mining on Social MediaData", IEEE 14th International Conference on Mobile Data Management,Milan, Italy, June 3- 6, pp 91-96, ISBN: 978-1- 494673-6068-5, 2013.
[14]. A. Sarlan, C. Nadam and S. Basri, “Twitter Sentiment Analysis”, in Int. Conf. on Information Technology and Multimedia,, pp. 213-216,2014
[15]. Pablo Gamallo and Marcos Garcia “A Naive-Bayes Strategy for Sentiment Analysis on English Tweets” Proceedings of the 8th International Workshop on Semantic Evaluation (SemEval 2014), pages 171–175, Dublin, Ireland, Aug 23-24 2014
[16]. Varsha Sahayak, Vijaya Shete and Apashabi Pathan, “Sentiment Analysis on Twitter Data”, (IJIRAE) ISSN: 2349-2163, January 2015.
[17]. Aliza Sarlan, Chayanit Nadam and Shuib Basri, “Twitter Sentiment Analysis”, 2014 International Conference on Information Technology and Multimedia (ICIMU), Putrajaya, Malaysia November 18 – 20, 2014.
[18]. Shi Yuan, Junjie Wu, Lihong Wang and Qing Wang, “A Hybrid Method for Multi-class Sentiment Analysis of Micro-blogs”, ISBN- 978-1-5090-2842-9, 2016.
[19]. Halima Banu S and S Chitrakala, “Trending Topic Analysis Using Novel Sub Topic Detection Model”, (IEEE) ISBN- 978-1-4673-9745-2, 2017.
[20]. David Zimbra, M. Ghiassi and Sean Lee, “Brand-Related Twitter Sentiment Analysis using Feature Engineering and the Dynamic Architecture for Artificial Neural Networks”, IEEE 1530-1605, 2016.