Automatic Segmentation and Categorization of the Brain Tumors
B Nandan1 , Kunjam Nageswara Rao2
Section:Research Paper, Product Type: Journal Paper
Volume-6 ,
Issue-9 , Page no. 391-397, Sep-2018
CrossRef-DOI: https://doi.org/10.26438/ijcse/v6i9.391397
Online published on Sep 30, 2018
Copyright © B Nandan, Kunjam Nageswara Rao . This is an open access article distributed under the Creative Commons Attribution License, which permits unrestricted use, distribution, and reproduction in any medium, provided the original work is properly cited.
View this paper at Google Scholar | DPI Digital Library
How to Cite this Paper
- IEEE Citation
- MLA Citation
- APA Citation
- BibTex Citation
- RIS Citation
IEEE Citation
IEEE Style Citation: B Nandan, Kunjam Nageswara Rao, “Automatic Segmentation and Categorization of the Brain Tumors,” International Journal of Computer Sciences and Engineering, Vol.6, Issue.9, pp.391-397, 2018.
MLA Citation
MLA Style Citation: B Nandan, Kunjam Nageswara Rao "Automatic Segmentation and Categorization of the Brain Tumors." International Journal of Computer Sciences and Engineering 6.9 (2018): 391-397.
APA Citation
APA Style Citation: B Nandan, Kunjam Nageswara Rao, (2018). Automatic Segmentation and Categorization of the Brain Tumors. International Journal of Computer Sciences and Engineering, 6(9), 391-397.
BibTex Citation
BibTex Style Citation:
@article{Nandan_2018,
author = {B Nandan, Kunjam Nageswara Rao},
title = {Automatic Segmentation and Categorization of the Brain Tumors},
journal = {International Journal of Computer Sciences and Engineering},
issue_date = {9 2018},
volume = {6},
Issue = {9},
month = {9},
year = {2018},
issn = {2347-2693},
pages = {391-397},
url = {https://www.ijcseonline.org/full_paper_view.php?paper_id=2879},
doi = {https://doi.org/10.26438/ijcse/v6i9.391397}
publisher = {IJCSE, Indore, INDIA},
}
RIS Citation
RIS Style Citation:
TY - JOUR
DO = {https://doi.org/10.26438/ijcse/v6i9.391397}
UR - https://www.ijcseonline.org/full_paper_view.php?paper_id=2879
TI - Automatic Segmentation and Categorization of the Brain Tumors
T2 - International Journal of Computer Sciences and Engineering
AU - B Nandan, Kunjam Nageswara Rao
PY - 2018
DA - 2018/09/30
PB - IJCSE, Indore, INDIA
SP - 391-397
IS - 9
VL - 6
SN - 2347-2693
ER -
![]() |
![]() |
![]() |
595 | 409 downloads | 191 downloads |
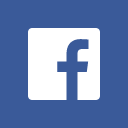
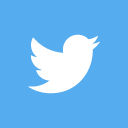
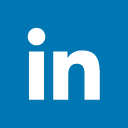
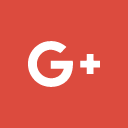
Abstract
Brain tumor detection and extraction within the time frame to offer better healthcare is vital and very important, but a time-consuming task performed by clinical supervisors or radiologists. Its accuracy for the brain tumor detection from modern imaging modalities also depends on their experience only. So the use of computer-aided methodology is very important to overcome these limitations. Generally, Cerebrum a tumor begins in the glial cells called Gliomas. Gliomas can be moderate developing (slow rate) or quickly developing (high rate). Doctors utilize the review of a mind tumor in light of gliomas to choose which treatment a patient needs. The state of the tumor is of indispensable significance for the treatment. In this paper, we propose a mechanized framework to separate between typical mind and strange cerebrum with tumor in the MRI pictures and furthermore additionally arrange the anomalous cerebrum tumors into High Rate or Low Rate tumors. The proposed framework utilizes KMFCM as the division strategy for grouping while Discrete Wavelet Transform (DWT) Principal Component Analysis (PCA) and Support Vector Machine (SVM)are the primary algorithms used. The calculated values of Cho/Cr and Cho/NAA of 15 different patients of different ages of both genders data is extracted from Brats-2017dataset are used classify into tumor grades.
Key-Words / Index Term
Tumors, Cho/Cr,Cho/NAA, DWT, PCA, High rate, Low rate, Gliomas
References
[1] E.-S. A. El-Dahshan, H. M. Mohsen, K. Revett, and A.-B. M. Salem, “Computer-aided diagnosis of human brain tumor through MRI: A survey and a new algorithm,” Expert Systems with Applications, vol. 41, no. 11, pp. 5526–5545, 2014.
[2] Wang, P., & Wang, H. L. (2008). A modified FCM algorithm for MRI brain image segmentation. In International seminar on Proceedings of future biomedical information engineering, 2008. FBIE’08 (pp. 26–29).
[3] Kim, JiHoon, et al. "Using a method based on a modified K-means clustering and mean shift segmentation to reduce file sizes and detect brain tumors from magnetic resonance (MRI) images." Wireless Personal Communications89.3 (2016): 993-1008.
[4] Beucher S., & Lantuejoul, C. (1979) Use of watershed in contour detection. In Proceedings of international workshop on image processing. CCETT/IRISA, Rennes, France.
[5] Friedman, J.H.: Greedy function approximation: a gradient boosting machine. Ann. stat. 1189–1232 (2001).
[6] P. Katti, V. R. Marathe, “Implementation of Classification System for Brain Tumor using Probabilistic Neural Network,” International Journal of Advanced Research in Computer and Communication Engineering, Vol. 4, no. 10, pp. 188-192, October 2015.
[7] C. L. Devasena and M. Hemalatha, “Efficient computer aided diagnosis of abnormal parts detection in magnetic resonance images using hybrid abnormality detection algorithm,”Central European Journal of Computer Science, vol. 3, no. 3, pp. 117–128, 2013.
[8] J. Cheng, X. Chen, H. Yang, and M. Leng, “An enhanced kmeans algorithm using agglomerative hierarchical clustering strategy,” International Conference on Automatic Control and Artificial Intelligence (ACAI 2012). pp. 407–410, 2012.
[9] E. Dandil, M. Cakiroglu, Z. Eksi, “Computer-aided diagnosis of malign and benign brain tumors on MR images,” ICT Innovations 2014, pp. 157–166, 2015.
[10] A. Batra, Dr. G. Kaushik, “SECTUBIM: Automatic Segmentation And Classification of Tumeric Brain MRI Images using FHS (FCM, HWT and SVM),” International Journal of Engineering Science and Computing, Vol. 7, no.6, pp. 13190-13194, June 2017.
[11] M. B. M. Amien, A. Abd-elrehman, and W. Ibrahim, “An intelligent model for automatic brain-tumor diagnosis basedon MRI images,” International Journal of Computer Applications, vol. 72, no. 23, pp. 21– 24, June 2013.
[12] Mohana Priya K, Kavitha S, bharathi B, “Brain Tumor Types and Grades Classification Based on Statistical Feature set using Support Vector Machine”, 10th International Conference on Intelligent Systems and Control (ISCO), Nov 2016.
[13] Ahmed Kharrat, Karim gasmi, Mohamed Ben Messaoud, Nacra Benamrane and Mohamed Abid, “A Hybrid Approach for Automatic Classification of brain MRI using Genetic Algorithm and Support Vector Machine”, Lenardo Journal of Sciences, vol.17,pp.71-82,2010.
[14] Sonali B Gaikwad, madhuri S Joshi, “Brain Tumor Classification Using Principal Component Analysis and Probabilistic Neural Network”, International Journal for Computer Applications, Vol-120, no:3,pp.5-9, June 2015
[15] K.B.Vaishnavee, K.Amshakala, “An automated MRI Brain Image Segmentation and Tumor Detection using SOM-Clustering and Proximal Support Vector Machine Classifier”, ICETECH, 20 March 2015.
[16] S. K. Shrivastava and S. S. Gharde, “Support vector machine for handwritten devanagari numeral recognition,” International Journal of Computer Applications, vol. 07, no. 11, pp. 09–14, October 2010