ECG Signal Classification Based On Deep Learning Classifier
R. Kavitha1 , T.Christopher 2
Section:Review Paper, Product Type: Journal Paper
Volume-6 ,
Issue-9 , Page no. 438-441, Sep-2018
CrossRef-DOI: https://doi.org/10.26438/ijcse/v6i9.438441
Online published on Sep 30, 2018
Copyright © R. Kavitha, T.Christopher . This is an open access article distributed under the Creative Commons Attribution License, which permits unrestricted use, distribution, and reproduction in any medium, provided the original work is properly cited.
View this paper at Google Scholar | DPI Digital Library
How to Cite this Paper
- IEEE Citation
- MLA Citation
- APA Citation
- BibTex Citation
- RIS Citation
IEEE Style Citation: R. Kavitha, T.Christopher, “ECG Signal Classification Based On Deep Learning Classifier,” International Journal of Computer Sciences and Engineering, Vol.6, Issue.9, pp.438-441, 2018.
MLA Style Citation: R. Kavitha, T.Christopher "ECG Signal Classification Based On Deep Learning Classifier." International Journal of Computer Sciences and Engineering 6.9 (2018): 438-441.
APA Style Citation: R. Kavitha, T.Christopher, (2018). ECG Signal Classification Based On Deep Learning Classifier. International Journal of Computer Sciences and Engineering, 6(9), 438-441.
BibTex Style Citation:
@article{Kavitha_2018,
author = {R. Kavitha, T.Christopher},
title = {ECG Signal Classification Based On Deep Learning Classifier},
journal = {International Journal of Computer Sciences and Engineering},
issue_date = {9 2018},
volume = {6},
Issue = {9},
month = {9},
year = {2018},
issn = {2347-2693},
pages = {438-441},
url = {https://www.ijcseonline.org/full_paper_view.php?paper_id=2887},
doi = {https://doi.org/10.26438/ijcse/v6i9.438441}
publisher = {IJCSE, Indore, INDIA},
}
RIS Style Citation:
TY - JOUR
DO = {https://doi.org/10.26438/ijcse/v6i9.438441}
UR - https://www.ijcseonline.org/full_paper_view.php?paper_id=2887
TI - ECG Signal Classification Based On Deep Learning Classifier
T2 - International Journal of Computer Sciences and Engineering
AU - R. Kavitha, T.Christopher
PY - 2018
DA - 2018/09/30
PB - IJCSE, Indore, INDIA
SP - 438-441
IS - 9
VL - 6
SN - 2347-2693
ER -
![]() |
![]() |
![]() |
347 | 203 downloads | 186 downloads |
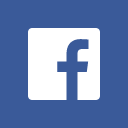
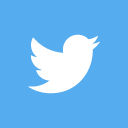
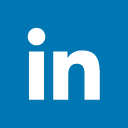
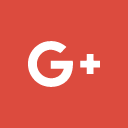
Abstract
ECG (Electrocardiogram), non-stationary biomedical signal measures the electrical activity of the human heart. This ECG signal helps the professional as a diagnostic tool to predict the cardiac disorder and the function of the human heart. According to the report of WHO (World Health Organization), most of the humans suffer from the cardiac disorder and passed due to the cardiac illness.ECG signal analysis is an important factor in this prediction and this work proposes an automatic classification of the signal which identifies the normal and abnormal signal. The ECG signals are taken from the MIT BIH database. The ECG signal is identified as normal and abnormal with the deep learning classifier CNN. The CNN is a convolution neural network which requires minimal pre-processing compared with traditional machine learning classifier. This work focus on the prediction of the cardiac disorder with automatic classification.
Key-Words / Index Term
ECG, Arrhythmia, MIT-BIH, CNN
References
[1] Latifah Aljafar et., al., "Classification of ECG signals of normal and abnormal subjects using common spatial pattern”, IEEE 5th International Conference, 2016.
[2] G.Kaur, G Singh and V Kumar, "A review on biometric recognition", International Journal of Bio-Science and Biotechnology, Vol. 6, no:4, 2014.
[3] z.Deng, M. Zhar, et.al., "Deep Structured models for group activity recognization", British Machine Vision Conference 2015.
[4] R. Collobert and J. Weston, “A unified architecture for natural language processing: Deep neural networks with multitask learning,” in Proceedings of the 25th International Conference on Machine Learning. ACM, Pg No: 160–167, 2008.
[5] K. Fukushima, “Neocognitron: A self-organizing neural network model for a mechanism of pattern recognition unaffected by shift in position,” Biological Cybernetics, vol. 36, no. 4, pp. 193–202, 1980.
[6] M. Abo-Zahhad, S. M. Ahmed, and S. N. Abbas, "Biometric authentication based on PCG and ECG signals: present status and future directions," Signal, Image and Video Processing, vol. 8, no. 4, pp. 739– 751, 2014.
[7] R.Kavitha, Dr.T.Christopher " Predicting Accuracy in ECG signal classification: A comparative method for feature selection", Journal of advanced research and dynamic control systems, vol.10 pp:273-281,2018.