A Brief Survey Ondynamic Topic Model for Unsupervised Object Discovery and Localization
Mereena Johny1 , L. Haldurai2
Section:Survey Paper, Product Type: Journal Paper
Volume-6 ,
Issue-9 , Page no. 567-571, Sep-2018
CrossRef-DOI: https://doi.org/10.26438/ijcse/v6i9.567571
Online published on Sep 30, 2018
Copyright © Mereena Johny, L. Haldurai . This is an open access article distributed under the Creative Commons Attribution License, which permits unrestricted use, distribution, and reproduction in any medium, provided the original work is properly cited.
View this paper at Google Scholar | DPI Digital Library
How to Cite this Paper
- IEEE Citation
- MLA Citation
- APA Citation
- BibTex Citation
- RIS Citation
IEEE Style Citation: Mereena Johny, L. Haldurai, “A Brief Survey Ondynamic Topic Model for Unsupervised Object Discovery and Localization,” International Journal of Computer Sciences and Engineering, Vol.6, Issue.9, pp.567-571, 2018.
MLA Style Citation: Mereena Johny, L. Haldurai "A Brief Survey Ondynamic Topic Model for Unsupervised Object Discovery and Localization." International Journal of Computer Sciences and Engineering 6.9 (2018): 567-571.
APA Style Citation: Mereena Johny, L. Haldurai, (2018). A Brief Survey Ondynamic Topic Model for Unsupervised Object Discovery and Localization. International Journal of Computer Sciences and Engineering, 6(9), 567-571.
BibTex Style Citation:
@article{Johny_2018,
author = {Mereena Johny, L. Haldurai},
title = {A Brief Survey Ondynamic Topic Model for Unsupervised Object Discovery and Localization},
journal = {International Journal of Computer Sciences and Engineering},
issue_date = {9 2018},
volume = {6},
Issue = {9},
month = {9},
year = {2018},
issn = {2347-2693},
pages = {567-571},
url = {https://www.ijcseonline.org/full_paper_view.php?paper_id=2909},
doi = {https://doi.org/10.26438/ijcse/v6i9.567571}
publisher = {IJCSE, Indore, INDIA},
}
RIS Style Citation:
TY - JOUR
DO = {https://doi.org/10.26438/ijcse/v6i9.567571}
UR - https://www.ijcseonline.org/full_paper_view.php?paper_id=2909
TI - A Brief Survey Ondynamic Topic Model for Unsupervised Object Discovery and Localization
T2 - International Journal of Computer Sciences and Engineering
AU - Mereena Johny, L. Haldurai
PY - 2018
DA - 2018/09/30
PB - IJCSE, Indore, INDIA
SP - 567-571
IS - 9
VL - 6
SN - 2347-2693
ER -
![]() |
![]() |
![]() |
289 | 242 downloads | 277 downloads |
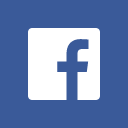
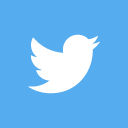
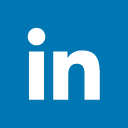
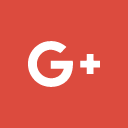
Abstract
With the explosion of the number of images in personal and on-line collections, efficient techniques for navigating, indexing, labelling and searching images become more and more important. In several studies, the representation of images by topic models in its various aspects and extend the current models. This paper aims to present a brief survey on knowledge based topic model for Unsupervised Object Discovery and Localization techniques in which the goal is to maximize the amount of work needed to re-optimize the solution when the object changes. Number of relative studies namely Latent Dirichlet allocation (LDA) with Multi-Domain Knowledge (MDK), Collaborative randomized search algorithm, Conditional random field and LDA with mixture of Dirichlet trees algorithms are discussed and evaluate the accuracy performance on the several datasets. Comparing to these algorithms the LDA with mixture of tree technique methods having better performance than other methods.
Key-Words / Index Term
Object discovery, object localization, topic model, and latentDirichlet allocation
References
[1] J. Deng, W. Dong, R. Socher, L.-J. Li, K. Li, and L. Fei-Fei. ImageNet: A large-scale hierarchical image database. In CVPR, 2009.
[2] M. Everingham, L. Van Gool, C. K. I.Williams, J.Winn, and A. Zisserman. The PASCAL Visual Object Classes Challenge 2007 (VOC2007)
[3] T. Deselaers, B. Alexe, and V. Ferrari. “Localizing objects while learning their appearance”. In ECCV, 2010.
[4] R. G. Cinbis, J. Verbeek, and C. Schmid. “Multi-fold MIL training for weakly supervised object localization”. In CVPR, 2014.
[5] A. Joulin, F. Bach, and J. Ponce. Discriminative clustering for image co-segmentation. In CVPR, 2010.
[6] A. Joulin, K. Tang, and L. Fei-Fei. “Efficient image and video co-localization with frank-wolfe algorithm”. In ECCV, 2014.
[7] M. H. Nguyen, L. Torresani, F. de la Torre, and C. Rother. Weakly supervised discriminative localization and classification: a joint learning process. In ICCV, 2009.
[8] A. W. M. Smeulders, M. Worring, S. Santini, A. Gupta, and R. Jain. Content-based image retrieval at the end of the early years. IEEE Trans. Pattern Anal. Mach. Intell., 22(12):1349–1380, 2000.
[9] A. Dong and B. Bhanu. Active concept learning for image retrieval in dynamic databases. In ICCV ’03: Proceedings of the Ninth IEEE International Conference on Computer Vision, page 90, 2003.
[10] T. Gevers and A. W. M. Smeulders. Content-based image retrieval: an overview. In G. Medioni and S. B. Kang, editors, Emerging Topics in Computer Vision, pages 333 –384. Prentice Hall, 2004.
[11] Y. Rui, T. S. Huang, and S.-F. Chang. Image retrieval: Current techniques, promising directions, and open issues. Journal of Visual Communication and Image Representation, 10(1):39–62, 1999.
[12] C. Schmid and R. Mohr. Local greyvalue invariants for image retrieval. IEEE Transactions on Pattern Analysis and Machine Intelligence, 19:530–535, 1997.
[13] R. Lienhart and A. Hartmann. Classifying images on the web automatically. Journal of Electronic Imaging, 11(4):445–454, 2002.
[14] D. Mimno, H. M. Wallach, E. Talley, M. Leenders, and A. McCallum, “Optimizing semantic coherence in topic models,” in Proc. EMNLP, 2011, pp. 262–272.
[15] T. Deselaers, B. Alexe, and V. Ferrari, “Weakly supervised localization and learning with generic knowledge,” Int. J. Comput. Vis., vol. 100, no. 3, pp. 275–293, Dec. 2012.
[16] Z. Chen, A. Mukherjee, B. Liu, M. Hsu, M. Castellanos, and R. Ghosh, “Leveraging multi-domain prior knowledge in topic models,” in Proc. IJCAI, 2013, pp. 2071–2077.
[17] M. Rubinstein, J. Kopf, C. Liu, and A. Joulin, “Unsupervised joint object discovery and segmentation in Internet images,” in Proc. CVPR, Jun. 2013, pp. 1939–1946.
[18] A. Faktor and M. Irani, “Clustering by composition’—Unsupervised discovery of image categories,” IEEE Trans. Pattern Anal. Mach. Intell., vol. 36, no. 6, pp. 1092–1106, Jun. 2014.
[19] A. Joulin, K. Tang, and L. Fei-Fei, “Efficient image and video co-localization with Frank–Wolfe algorithm,” in Proc. ECCV, 2014, pp. 253–268.
[20] Z. Niu, G. Hua, X. Gao, and Q. Tian, “Semi-supervised relational topic model for weakly annotated image recognition in social media,” in Proc. CVPR, Jun. 2014, pp. 4233–4240.
[21] C. Wang, K. Huang, W. Ren, J. Zhang, and S. Maybank, “Largescale weakly supervised object localization via latent category learning,” IEEE Trans. Image Process., vol. 24, no. 4, pp. 1371–1385, Apr. 2015.
[22] M. Cho, S. Kwak, C. Schmid, and J. Ponce, “Unsupervised object discovery and localization in the wild: Part-based matching with bottomup region proposals,” in Proc. CVPR, Jun. 2015, pp. 1201–1210.
[23] Zhenzhen Wang ; Junsong Yuan, “Simultaneously Discovering and Localizing Common Objects in Wild Images”, IEEE Transactions on Image Processing ( Volume: 27, Issue: 9, Sept. 2018 )
[24] Zhenxing Niu, Gang Hua, Le Wang, Member, and Xinbo Gao, “Knowledge-Based Topic Model for Unsupervised Object Discovery and Localization”, IEEE TRANSACTIONS on image processing, vol. 27, no. 1, january 2018