A Survey of Machine Learning Appliacations In Decision Making To Improve Farming
Ankita Bissa1 , Meena Kushwaha2 , Mayank Patel3
Section:Survey Paper, Product Type: Journal Paper
Volume-6 ,
Issue-9 , Page no. 669-683, Sep-2018
CrossRef-DOI: https://doi.org/10.26438/ijcse/v6i9.669683
Online published on Sep 30, 2018
Copyright © Ankita Bissa, Meena Kushwaha, Mayank Patel . This is an open access article distributed under the Creative Commons Attribution License, which permits unrestricted use, distribution, and reproduction in any medium, provided the original work is properly cited.
View this paper at Google Scholar | DPI Digital Library
How to Cite this Paper
- IEEE Citation
- MLA Citation
- APA Citation
- BibTex Citation
- RIS Citation
IEEE Citation
IEEE Style Citation: Ankita Bissa, Meena Kushwaha, Mayank Patel, “A Survey of Machine Learning Appliacations In Decision Making To Improve Farming,” International Journal of Computer Sciences and Engineering, Vol.6, Issue.9, pp.669-683, 2018.
MLA Citation
MLA Style Citation: Ankita Bissa, Meena Kushwaha, Mayank Patel "A Survey of Machine Learning Appliacations In Decision Making To Improve Farming." International Journal of Computer Sciences and Engineering 6.9 (2018): 669-683.
APA Citation
APA Style Citation: Ankita Bissa, Meena Kushwaha, Mayank Patel, (2018). A Survey of Machine Learning Appliacations In Decision Making To Improve Farming. International Journal of Computer Sciences and Engineering, 6(9), 669-683.
BibTex Citation
BibTex Style Citation:
@article{Bissa_2018,
author = {Ankita Bissa, Meena Kushwaha, Mayank Patel},
title = {A Survey of Machine Learning Appliacations In Decision Making To Improve Farming},
journal = {International Journal of Computer Sciences and Engineering},
issue_date = {9 2018},
volume = {6},
Issue = {9},
month = {9},
year = {2018},
issn = {2347-2693},
pages = {669-683},
url = {https://www.ijcseonline.org/full_paper_view.php?paper_id=2926},
doi = {https://doi.org/10.26438/ijcse/v6i9.669683}
publisher = {IJCSE, Indore, INDIA},
}
RIS Citation
RIS Style Citation:
TY - JOUR
DO = {https://doi.org/10.26438/ijcse/v6i9.669683}
UR - https://www.ijcseonline.org/full_paper_view.php?paper_id=2926
TI - A Survey of Machine Learning Appliacations In Decision Making To Improve Farming
T2 - International Journal of Computer Sciences and Engineering
AU - Ankita Bissa, Meena Kushwaha, Mayank Patel
PY - 2018
DA - 2018/09/30
PB - IJCSE, Indore, INDIA
SP - 669-683
IS - 9
VL - 6
SN - 2347-2693
ER -
![]() |
![]() |
![]() |
904 | 557 downloads | 285 downloads |
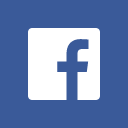
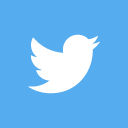
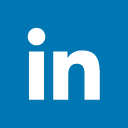
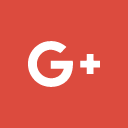
Abstract
Machine learning has developed with huge information innovations and best outline to make new opportunity for data science in the multi-disciplinary agriculture domain. In this paper, we present a far reaching review of research devoted to applications of machine learning in agrarian generation frameworks. The works broke down were classified in (a)crop management, including applications on yield expectation, infection identification, weed discovery, crop quality, and species acknowledgment; (b) domesticated animals management, including applications on creature welfare and animals generation; (c) water management; and (d) soil management. The review reports the application of machine learning techniques with artificial neural networks, Bayesian networks, support vector machines and k-means. The filtering and classification of the reviewed articles show how farming will benefit from machine learning advancements. By applying machine learning to sensor information, cultivate administration frameworks are advancing into ongoing artificial insight empowered projects that give rich proposals also, bits of knowledge for agriculturist choice help and activity.
Key-Words / Index Term
Machine learning, Artificial Intelligence, Big data, Artificial neural networks, Bayesian networks, Support vector machines and K-means
References
[1] Meena Kushwaha and D. V. R. Raghuveer, "Survey of Impact of Technology on Effective Implementation of Precision Farming in India," International Journal on Recent and Innovation Trends in Computing and Communication, vol. 5, no. 6, p. 11, 2017.
[2] T. Ranjeet and L. Armstrong, “An artificial neural network for predicting crops yield in Nepal”, Proceedings of the 9th Conference of the Asian Federation for Information Technology in Agriculture “ICT’s for future Economic and Sustainable Agricultural Systems”, Perth, Australia, pp.376-386, 2014.
[3] S. Jabjone and S. Wannasang, "Decision Support System Using Artificial Neural Network to Predict Rice Production in Phimai District, Thailand," International Journal of Computer and Electrical Engineering, vol. 6, no. 2, pp. 162-166, 2014.
[4] N. Gandhi, L. J. Armstrong, and O. Petkar, “PredictingRice crop yield using Bayesian networks,” 2016 International Conference on Advances in Computing, Communications and Informatics (ICACCI), pp. 795–799, 2016.
[5] S. Dahikar and S. Rode, "Agricultural Crop Yield Prediction Using Artificial Neural Network Approach", International Journal of Innovative Research in Electrical, Electronics, Instrumentation, and Control Engineering, vol. 2, no. 1, 2014.
[6] D. Jiang, X. Yang, N. Clinton, and N. Wang, "An artificial neural network model for estimating crop yields using remotely sensed information," International Journal of Remote Sensing, vol. 25, no. 9, pp. 1723-1732, 2004.
[7] M. Kaul, R. L. Hill, and C. Walthall, "Artificial neural networks for corn and soybean yield prediction," Agricultural Systems, vol. 85, no. 1, pp. 1-18, 2005.
[8] K. Movagharnejad and M. Nikzad, "Modeling of tomato drying using the artificial neural network," Computers and Electronics in Agriculture, vol. 59, no. 1-2, pp. 78-85, 2007.
[9] M. R. O`Neal, B. A. Engel, D. R. Ess, and J. R. Frankenberger, "AE—Automation and Emerging Technologies," Biosystems Engineering, vol. 83, no. 1, pp. 31-45, 2002.
[10] K. Ostad-Ali-Askari, M. Shayannejad, and H. Ghorbanizadeh-Kharazi, "Artificial neural network for modeling nitrate pollution of groundwater in the marginal area of Zayandeh-Rood River, Isfahan, Iran," KSCE Journal of Civil Engineering, vol. 21, no. 1, pp. 134-140, 2016.
[11] J. Y. Shin, M. Ajmal, J. Yoo, and T.-W. Kim, "A Bayesian Network-Based Probabilistic Framework for Drought Forecasting and Outlook," Advances in Meteorology, vol. 2016, pp. 1-10, 2016.
[12] Aalders, “Modeling land-use decision behavior with Bayesian belief networks”, Ecology and Society, vol.13, no. 1, pp. 16, 2008.
[13] G. C. Chunguang Bi "Bayesian Networks Modeling for Crop Diseases," IFIP International Federation for Information Processing 2011, p. 8, 2011.
[14] M. A. E. Forio et al., "Bayesian belief network models to analyze and predict ecological water quality in rivers," Ecological Modelling, vol. 312, pp. 222-238, 2015.
[15] N. Gandhi, L. Armstrong, and O. Petkar, "Predicting Rice Crop Yield using Bayesian Networks", In Proceedings of IEEE 5th International Advances in Computing, Communications, and Informatics, E-ISBN: 978-1-5090-2029-4, 2016.
[16] S. Madadgar and H. Moradkhani, "Spatio-temporal drought forecasting within Bayesian networks," Journal of Hydrology, vol. 512, pp. 134-146, 2014.
[17] S. Maiti and R. K. Tiwari, "A comparative study of artificial neural networks, Bayesian neural networks and adaptive neuro-fuzzy inference system in groundwater level prediction," Environmental Earth Sciences, vol. 71, no. 7, pp. 3147-3160, 2013.
[18] G. S. Nearing, H. V. Gupta, and W. T. Crow, "Information loss in approximately Bayesian estimation techniques: A comparison of generative and discriminative approaches to estimating agricultural productivity," Journal of Hydrology, vol. 507, pp. 163-173, 2013.
[19] C. Perez-Ariza, A. Nicholson and M. Flores, "Prediction of coffee rust disease using Bayesian networks", In Proceedings of the Sixth European Workshop on Probabilistic Graphical Models, pp. 259-266, 2012.
[20] J. M. Quinn, R. M. Monaghan, V. J. Bidwell, and S. R. Harris, "A Bayesian Belief Network approach to evaluating complex effects of irrigation-driven agricultural intensification scenarios on future aquatic environmental and economic values in a New Zealand catchment," Marine and Freshwater Research, vol. 64, no. 5, 2013.
[21] A. Sharma and M. Goyal, "Bayesian network model for monthly rainfall forecast", 2015 IEEE International Conference on Research in Computational Intelligence and Communication Networks (ICRCICN), pp. 241-246, 2015.
[22] C.-J. Du and D.-W. Sun, "Pizza sauce spread classification using color vision and support vector machines," Journal of Food Engineering, vol. 66, no. 2, pp. 137-145, 2005.
[23] V. Anandhi and R. Chezian, “Support Vector Regression in forecasting”, International Journal of Advanced Research in Computer and Communication Engineering, vol. 2, no. 10, pp. 4148-4151, 2013.
[24] S. Brdar, D. Culibrk, B. Marinkovic, J. Crnobarac and V. Cmojevic, “Support Vector Machines with Features Contribution Analysis for Agricultural Yield Prediction”, 2011
[25] K. Brudzewski, "Classification of milk by means of an electronic nose and SVM neural network," Sensors and Actuators B: Chemical, vol. 98, no. 2-3, pp. 291-298, 2004.
[26] S. Fagerlund, "Bird Species Recognition Using Support Vector Machines," EURASIP Journal on Advances in Signal Processing, vol. 2007, no. 1, 2007.
[27] A. Bharadwaj, S. Dahiya and R. Jain, "Discretization based Support Vector Machine (D-SVM) for Classification of Agricultural Datasets", International Journal of Computer Applications, vol. 40, no. 1, pp. 8-12,2012.
[28] N. Gandhi, L. Armstrong, O. Petkar and A. Tripathy, "Rice Crop YieldPrediction in India using Support Vector Machines", In Proceedings of IEEE The 13th International Joint Conference on Computer Science and Software Engineering (JCSSE), 2016.
[29] B. Huang, C. Xie, and R. Tay, "Support vector machines for urban growth modeling," GeoInformatica, vol. 14, no. 1, pp. 83-99, 2009.
[30] Y. Karimi, S. O. Prasher, R. M. Patel, and S. H. Kim, "Application of support vector machine technology for weed and nitrogen stress detection in corn," Computers and Electronics in Agriculture, vol. 51, no. 1-2, pp. 99-109, 2006.
[31] G. Camps-Valls, L. Gomez-Chova, J. Calpe-Maravilla, E. Soria-Olivas, JD. Martin-Guerrero and J. Moreno, “Support vector machines for crop classification using hyperspectral data”, Lect Notes CompSci, 2652, pp. 134–141, 2003.
[32] B. S. Shedthi, S. Shetty, and M. Siddappa, “Implementation and comparison of K-means and fuzzy C-means algorithms for agricultural data,” 2017 International Conference on Inventive Communication and Computational Technologies (ICICCT), 2017.
[33] Stewart, J., Stewart, R., & Kennedy, S. (2017). Dynamic IoT management system using K-means machine learning for precision agriculture applications. Proceedings of the Second International Conference on Internet of things and Cloud Computing - ICC `17.
[34] P. K. Dr. SUJATHA S, "Smart Farming using K-means Clustering and SVM Classifier in Image Processing," International Journal of Science, Engineering and Technology Research, vol. 6, no. 11, p. 5, 2017.
[35] G. Chen, Y. Yang, H. Guo, X. Sun, H. Chen, and L. Cai, "Analysis and Research of K-means Algorithm in Soil Fertility Based on Hadoop Platform," Computer and Computing Technologies in Agriculture VIII IFIP Advances in Information and Communication Technology, pp. 304–312, 2015.
[36] J. Baghel and P. Jain, “Disease Detection in Soya Bean using K-Means Clustering Segmentation Technique,” International Journal of Computer Applications, vol. 145, no. 9, pp. 15–18, 2016.
[37] A. Khatra, "Yellow Rust Extraction in Wheat Crop-based on Color Segmentation Techniques," IOSR Journal of Engineering, vol. 3, no. 12, pp. 56–58, 2013.
[38] A. Tellaeche, X.-P. Burgosartizzu, G. Pajares, and A. Ribeiro, “A Vision-Based Hybrid Classifier for Weeds Detection in Precision Agriculture Through the Bayesian and Fuzzy k-Means Paradigms,” Advances in Soft Computing Innovations in Hybrid Intelligent Systems, pp. 72–79, 2007.
[39] T Williams and Dr. P. ShanthiBala, "To Recognize the Crop Growth Rate in Agricultural Land By Using K-Means Clustering Algorithm and Contrast Enhancement Algorithm," International Journal of Scientific Research in Computer Science, Engineering and Information Technology, vol. 3, no. 5, pp. 41–47, 2018.
[40] J. Tang, D. Wang, Z. Zhang, L. He, J. Xin, and Y. Xu, "Weed identification based on K-means feature learning combined with convolutional neural network," Computers and Electronics in Agriculture, vol. 135, pp. 63-70, 2017.
[41] R. K N, C. N, O. S N, M. B. Meenavathi, and R. V, "Detection of Rows in Agricultural Crop Images Acquired by Remote Sensing from a UAV," International Journal of Image, Graphics and Signal Processing, vol. 8, no. 11, pp. 25-31, 2016.