Feedback Rate Based User Order Predication (FR-UOP) Model for Sentiment Analysis in Data Mining
A. Suriya1 , M. Prabakaran2
Section:Research Paper, Product Type: Journal Paper
Volume-6 ,
Issue-9 , Page no. 793-797, Sep-2018
CrossRef-DOI: https://doi.org/10.26438/ijcse/v6i9.793797
Online published on Sep 30, 2018
Copyright © A. Suriya, M. Prabakaran . This is an open access article distributed under the Creative Commons Attribution License, which permits unrestricted use, distribution, and reproduction in any medium, provided the original work is properly cited.
View this paper at Google Scholar | DPI Digital Library
How to Cite this Paper
- IEEE Citation
- MLA Citation
- APA Citation
- BibTex Citation
- RIS Citation
IEEE Style Citation: A. Suriya, M. Prabakaran, “Feedback Rate Based User Order Predication (FR-UOP) Model for Sentiment Analysis in Data Mining,” International Journal of Computer Sciences and Engineering, Vol.6, Issue.9, pp.793-797, 2018.
MLA Style Citation: A. Suriya, M. Prabakaran "Feedback Rate Based User Order Predication (FR-UOP) Model for Sentiment Analysis in Data Mining." International Journal of Computer Sciences and Engineering 6.9 (2018): 793-797.
APA Style Citation: A. Suriya, M. Prabakaran, (2018). Feedback Rate Based User Order Predication (FR-UOP) Model for Sentiment Analysis in Data Mining. International Journal of Computer Sciences and Engineering, 6(9), 793-797.
BibTex Style Citation:
@article{Suriya_2018,
author = {A. Suriya, M. Prabakaran},
title = {Feedback Rate Based User Order Predication (FR-UOP) Model for Sentiment Analysis in Data Mining},
journal = {International Journal of Computer Sciences and Engineering},
issue_date = {9 2018},
volume = {6},
Issue = {9},
month = {9},
year = {2018},
issn = {2347-2693},
pages = {793-797},
url = {https://www.ijcseonline.org/full_paper_view.php?paper_id=2946},
doi = {https://doi.org/10.26438/ijcse/v6i9.793797}
publisher = {IJCSE, Indore, INDIA},
}
RIS Style Citation:
TY - JOUR
DO = {https://doi.org/10.26438/ijcse/v6i9.793797}
UR - https://www.ijcseonline.org/full_paper_view.php?paper_id=2946
TI - Feedback Rate Based User Order Predication (FR-UOP) Model for Sentiment Analysis in Data Mining
T2 - International Journal of Computer Sciences and Engineering
AU - A. Suriya, M. Prabakaran
PY - 2018
DA - 2018/09/30
PB - IJCSE, Indore, INDIA
SP - 793-797
IS - 9
VL - 6
SN - 2347-2693
ER -
![]() |
![]() |
![]() |
463 | 264 downloads | 231 downloads |
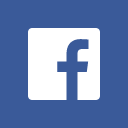
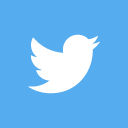
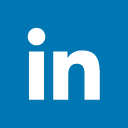
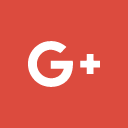
Abstract
Sentiment analysis is an examination philosophy for estimating the behavior of users through the investigation of past Opinion information. Behavioral financial aspects and quantitative examination utilize a large number of similar instruments of specialized study, which being a part of dynamic management. The capability of both dedicated and principal investigation is examined by the useful opinion mining which expresses that securities exchange logs are feedback. Sentiment analysis entirely relies on user enthusiasm and also user relationship about the feedback. Opinion mining is a way to deal with services connection with present and potential users. It utilizes information investigation about user history with a gathering to enhance services relationships with users, mainly concentrating on user maintenance and at last driving deals development. The issue of user premium expectation has been examined in the other circumstance, and there are a few strategies has been explored before. The effect of sentiment analysis in opinion mining could be adjusted for different issues like user seek, item inspiration, etc. To enhance the execution of user logs in opinion mining, a novel Feedback Rate based User Order Predication (FR-UOP) model for sentiment analysis scheme has been examined in this paper. The FR-UOP calculation first preprocesses the user log information to part them into the time-space. At that point, the strategy differentiates the rundown of user curiosity of the feedback rate in particular concern of the user and enhances the execution of user relationship in data mining.
Key-Words / Index Term
Sentiment Analysis, opinion model, user log, feedback rate, data mining
References
[1]. Song, H., Chen, C., & Yu, Q. “Research on Kano model based on online comment data mining”. 2018 IEEE 3rd International Conference on Big Data Analysis (ICBDA), 2018.
[2]. Angelo, T. N., de Faissol Attux, R. R., & Pagan, C. J. B. “DEMOS Project: a Proposal for Construction of the Deliberative Democracy from the Knowledge of Public Opinion”. 2018 International Conference on eDemocracy & eGovernment (ICEDEG), 2017.
[3]. Zvarevashe, Olugbara, “A framework for sentiment analysis with opinion mining of hotel reviews”. ICTAS, 2018.
[4]. Cambria,Schuller, “New Avenues in Opinion Mining and Sentiment Analysis”. IEEE Intelligent Systems, 28(2) 2013.
[5]. Balazs, Velásquez, “Opinion Mining and Information Fusion: A survey. Information Fusion”, 27, 95–110, 2016.
[6]. Dhanalakshmi, Saravanan, “Opinion mining from student feedback data using supervised learning algorithms”. ICBDSC, 2016.
[7]. Morency, Mihalcea, “Towards multimodal sentiment analysis”. ICMI 11, 2011.
[8]. Fanelli, Torsello, “Mining usage profiles from access data using fuzzy clustering”, WSEAS International Conference on Simulation, 2006.
[9]. Cambria, E., Song, Y., Wang, H., & Howard, N. “Semantic Multidimensional Scaling for Open-Domain Sentiment Analysis”. IEEE Intelligent Systems, 29(2), 44–51, 2014.
[10]. Tempelaar, Rienties, Giesbers, “In the search for the most informative data for feedback generation: Learning analytics in a data-rich context”. Computers in Human Behavior, 47, 157–167, 2015.
[11]. Jaehong Park, Ravi Sandhu, Yuan Cheng, “User-Activity-Centric Framework for Access Control in Online Social Networks”, IEEE Internet Computing, 28 Feb. 2011.
[12]. Doina Caragea, Rohit Parimi, , “Predicting Friendship Links in Social Networks Using a Topic Modeling Approach”, Springer, Volume 6635, 2011, pp 75-86
[13]. H. Liu, Tang, “Scalable Learning of Collective Behavior Based on Sparse Social Dimensions”, CIKM , pp. 1107-1116, 2009.
[14]. Nesar Ahmad, Sufyan Beg, “Web search enhancement by mining user actions”, Journal of Information Sciences Vol-177 PP-5203-5218, 2007.
[15]. Lucchese, Orlando, “Detecting Task-based Query Sessions Using Collaborative Knowledge”, IEEE/WIC/ACM International Conference on Web Intelligence and Intelligent Agent Technology 2010.