Probabilistic Support Vector Regression Classification Model for Credit Card Fraud Detection
Nikita Sawhney1 , B. Kaur2 , H. Kaur3
Section:Research Paper, Product Type: Journal Paper
Volume-6 ,
Issue-9 , Page no. 840-843, Sep-2018
CrossRef-DOI: https://doi.org/10.26438/ijcse/v6i9.840843
Online published on Sep 30, 2018
Copyright © Nikita Sawhney, B. Kaur, H. Kaur . This is an open access article distributed under the Creative Commons Attribution License, which permits unrestricted use, distribution, and reproduction in any medium, provided the original work is properly cited.
View this paper at Google Scholar | DPI Digital Library
How to Cite this Paper
- IEEE Citation
- MLA Citation
- APA Citation
- BibTex Citation
- RIS Citation
IEEE Style Citation: Nikita Sawhney, B. Kaur, H. Kaur, “Probabilistic Support Vector Regression Classification Model for Credit Card Fraud Detection,” International Journal of Computer Sciences and Engineering, Vol.6, Issue.9, pp.840-843, 2018.
MLA Style Citation: Nikita Sawhney, B. Kaur, H. Kaur "Probabilistic Support Vector Regression Classification Model for Credit Card Fraud Detection." International Journal of Computer Sciences and Engineering 6.9 (2018): 840-843.
APA Style Citation: Nikita Sawhney, B. Kaur, H. Kaur, (2018). Probabilistic Support Vector Regression Classification Model for Credit Card Fraud Detection. International Journal of Computer Sciences and Engineering, 6(9), 840-843.
BibTex Style Citation:
@article{Sawhney_2018,
author = {Nikita Sawhney, B. Kaur, H. Kaur},
title = {Probabilistic Support Vector Regression Classification Model for Credit Card Fraud Detection},
journal = {International Journal of Computer Sciences and Engineering},
issue_date = {9 2018},
volume = {6},
Issue = {9},
month = {9},
year = {2018},
issn = {2347-2693},
pages = {840-843},
url = {https://www.ijcseonline.org/full_paper_view.php?paper_id=2953},
doi = {https://doi.org/10.26438/ijcse/v6i9.840843}
publisher = {IJCSE, Indore, INDIA},
}
RIS Style Citation:
TY - JOUR
DO = {https://doi.org/10.26438/ijcse/v6i9.840843}
UR - https://www.ijcseonline.org/full_paper_view.php?paper_id=2953
TI - Probabilistic Support Vector Regression Classification Model for Credit Card Fraud Detection
T2 - International Journal of Computer Sciences and Engineering
AU - Nikita Sawhney, B. Kaur, H. Kaur
PY - 2018
DA - 2018/09/30
PB - IJCSE, Indore, INDIA
SP - 840-843
IS - 9
VL - 6
SN - 2347-2693
ER -
![]() |
![]() |
![]() |
381 | 372 downloads | 240 downloads |
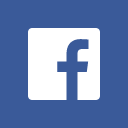
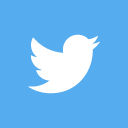
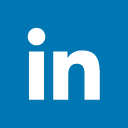
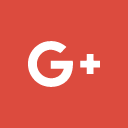
Abstract
The researchers have already worked with many supervised and unsupervised methods for the purpose of credit card fraud detection. The supervised models have been found more efficient for the purpose of credit card fraud detection. The major goal of the credit card fraud detection research is to improve the accuracy while decreasing the elapsed time. The proposed credit card fraud detection models purposes the use of feature extraction and selection of the credit card data with linear regression algorithm for the credit card fraud detection. The feature engineering and analysis would be performed over the given trans-actional data and then final classification of the anomalies or outliers is done using linear regression classifier. The proposed model has been tested under the various experiments from the various groups of test cases. The test case groups have been ob-tained after applying the various levels of the feature elimination and feature selection over the collection of credit card transac-tion data. The proposed credit card fraud classification model is based upon two different models, which includes the Naïve Bayes and Support Vector Regression. The main aim of the research is to achieve the higher credit card fraud recognition accuracy, with the minimum classification complexity.
Key-Words / Index Term
Credit card fraud detection, Early Fraud Detection, Regressive analysis, Linear regression models
References
[1]. Li, Jinyan, Simon Fong, Yan Zhuang, and Richard Khoury. "Hierarchical classification in pattern discovery for outlier analysis of credit card database." Soft Computing (2015): 1-10.
[2]. Prollochs, Nicolas, Stefan Feuerriegel, and Dirk Neumann. "Enhancing Outlier analysis of Financial News by Detecting Negation Scopes." InSystem Sciences (HICSS), 2015 48th Hawaii International Conference on, pp. 959-968. IEEE, 2015.
[3]. Cui, Limeng, Fan Meng, Yong Shi, Minqiang Li, and An Liu. "A Hierarchy Method Based on LDA and SVM for Credit card fraud detection." In Data Mining Workshop (ICDMW), 2014 IEEE International Conference on, pp. 60-64. IEEE, 2014.
[4]. Ouyang, Yuanxin, Yao Huangfu, Hao Sheng, and Zhang Xiong. " target on the problem of news title classification which is an essential and typical member in short text fami-ly." In Neural Information Processing, pp. 581-588. Springer International Publishing, 2014.
[5]. Krishnlal G, S Babu Rengarajan, K G Srinivasagan, “ A new pattern discovery approach based on HMM-SVM for web credit card fraud detection” International Journal of Computer Applications (0975-8887) Volumn 1- No.19,2010.
[6]. Vandana Korde, C namrata Mahender, “Text classification and classifier a survey,” International Journal of Artificial Intelligence and Application (IJAIA), vol.3, No.2, March2012.
[7]. Mita K. Dalal, Mukesh A.Zaveri,” Automatic text Classifica-tion,” International Journal of Computer Applications (0975-8887) Volumn 28- No.2, August 2011.
[8]. Denial I.Morariu, Lucian N. Vintan, and Volker Tresp, “Meta- Clalssification using SVM classifiers for text documents “, “World Academy of science engineering and technology” 21,2006.
[9]. Hyeran Byun 1 and Seong-Whan Lee2, “ Application of Sup-port Vector machines for pattern recognition: A Survey,” SVM 2002, LNCS 2388,pp.213-236,2002.
[10]. D. Morariu, R. Cre,Tulescu and L.,Vin,tan, “ improving the SVM Meta Clssifier for text document by using Naïve bayes,”Int. J. of Computers, communication and control, ISSN 1841-9844.
[11]. Lie Lu, Stan Z. Li and Hong –Jiang Zhang, “ Content based Audion Segmentation using Support vector machine.”
[12]. CREŢULESCU, Radu George, and N. VINŢAN Lucian. "Contributions to Document Classification System Design." Vol. 1, Issue 1, SIBIU, 2011.
[13]. Hyeran Byun 1 and Seong-Whan Lee2, “ Application of Sup-port Vector machines for pattern recognition: A Survey,” SVM 2002, LNCS 2388,pp.213-236,2002.
[14]. Krishnlal G, S Babu Rengarajan, K G Srinivasagan, “ A new pattern discovery approach based on HMM-SVM for web credit card fraud detection” International Journal of Computer Applications (0975-8887) Volumn 1- No.19,2010.
[15]. Zhang, Yulei, Yan Dang, Hsinchun Chen, Mark Thurmond, and Cathy Larson. "Automatic credit card database monitoring and classification for syndromic surveillance." Decision Support Systems 47, no. 4 (2009): 508-517.