Efficient Learning on Imbalanced Image Set
Shivani Guldas1
Section:Research Paper, Product Type: Journal Paper
Volume-6 ,
Issue-10 , Page no. 121-126, Oct-2018
CrossRef-DOI: https://doi.org/10.26438/ijcse/v6i10.121126
Online published on Oct 31, 2018
Copyright © Shivani Guldas . This is an open access article distributed under the Creative Commons Attribution License, which permits unrestricted use, distribution, and reproduction in any medium, provided the original work is properly cited.
View this paper at Google Scholar | DPI Digital Library
How to Cite this Paper
- IEEE Citation
- MLA Citation
- APA Citation
- BibTex Citation
- RIS Citation
IEEE Style Citation: Shivani Guldas, “Efficient Learning on Imbalanced Image Set,” International Journal of Computer Sciences and Engineering, Vol.6, Issue.10, pp.121-126, 2018.
MLA Style Citation: Shivani Guldas "Efficient Learning on Imbalanced Image Set." International Journal of Computer Sciences and Engineering 6.10 (2018): 121-126.
APA Style Citation: Shivani Guldas, (2018). Efficient Learning on Imbalanced Image Set. International Journal of Computer Sciences and Engineering, 6(10), 121-126.
BibTex Style Citation:
@article{Guldas_2018,
author = {Shivani Guldas},
title = {Efficient Learning on Imbalanced Image Set},
journal = {International Journal of Computer Sciences and Engineering},
issue_date = {10 2018},
volume = {6},
Issue = {10},
month = {10},
year = {2018},
issn = {2347-2693},
pages = {121-126},
url = {https://www.ijcseonline.org/full_paper_view.php?paper_id=2991},
doi = {https://doi.org/10.26438/ijcse/v6i10.121126}
publisher = {IJCSE, Indore, INDIA},
}
RIS Style Citation:
TY - JOUR
DO = {https://doi.org/10.26438/ijcse/v6i10.121126}
UR - https://www.ijcseonline.org/full_paper_view.php?paper_id=2991
TI - Efficient Learning on Imbalanced Image Set
T2 - International Journal of Computer Sciences and Engineering
AU - Shivani Guldas
PY - 2018
DA - 2018/10/31
PB - IJCSE, Indore, INDIA
SP - 121-126
IS - 10
VL - 6
SN - 2347-2693
ER -
![]() |
![]() |
![]() |
448 | 234 downloads | 243 downloads |
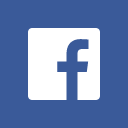
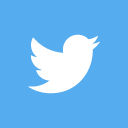
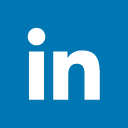
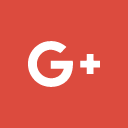
Abstract
Handling imbalanced image sets is a challenging issue being faced by the conventional categorizer. Imbalance problem occur with real world data due to various reasons, to which the ordinary classifiers gets influenced towards major class data. In this paper, we aim to balance bi-class absolute image set by creating synthetic samples of minority class images. Tests on three image sets using five synthetic image generation methods, four image features and three evaluation measures is carried out. KNN classification is performed on all three image set which are pretty imbalanced and the results indicate that synthetic creation of minor class images progresses the performance measures.
Key-Words / Index Term
Imbalanced image set, k-nn categorization, Synthetic image generation, performance measures improvement
References
[1] Dr. Durga Prasad, Dr. K Nageswar Rao, “An Imprved approach on class imbalance data using within class minority oversampling technique” International Journal of latest trends in engineering and technology, VOL.7, 2017l.
[2] Yingying Qin, Wenjie Chen, Jie Chen, “Generating images for imbalanced dataset problem” Institute of Electrical and Electronics Engineer (IEEE), 2016.
[3] Bartosz Krawezyk, “Learning from Imbalanced data: open challenges and future data”, 2016, Springerlink.com.
[4] Jiaojiao Li, Qian Du, Wei Li, Yunsng Li “Representation based yper spectral Image Classification with Imbalanced data”, Institute of Electrical and Electronics Engineers (IEEE), 2016.
[5] Gregory Luppescu, Raj Shah,“Personalized Image Enhancement” Institute of Electrical and Electronics Engineers(IEEE) , 2016.
[6] Anu Namdeo, Sandeep Singh Bhadriya, “A Review on Image enhancement techniques with its advantages and disadvantages” IJSART, 2016.
[7] Hebatallah Mostafa Anwer, Mohamed Farouk, Ayman Abdel-HamAida Ali, Siti Mariyam Shamssuddin, Anca L Ralescu, “Classification with class imbalance problem: A review” Intertiol Jourl Of Advances In Soft Computing And Its Applications, 7 (3). pp. 176-204, 2015.
[8] Vaishnavi L Kaundanya, Anita Patil, Ashish Panat “Classfication of Emotions from EGG using KNN classifier” 2015, IJSET.
[9] Apurva Sonak, R A Patankar “A survey on methods to handle Imbalance dataset” 2015 International Conference on Computing, Networking an d Communications(IJCSMC).
[10] Tdd Perry, Mhommed Bader-El-Den, Steven Cooper, “Imbalanced classification using Genetically Optimized Cost sensitive classifiers” Institute of Electrical and Electronics Engineers (IEEE),2015.
[11] M Srinivas, R Bharath, P Rajalakshmi, C Krishna Mohan, “Multi-level categorization method for medical methods”, 2015, 17th International conference n E-Health Networking, Application & Services (HealthCom).
[12] Dr. D Ramyachitra, P Manikandan,“ Imbalanced dataset classification and solutions: A Review” July 4 2014, International Journal of Computing and Business Research (IJCBR).
[13] M Akkil Jabbar, B L Deekshatulu, Priti Chandra “Classification of Heart disease using KNN and Genetic algorithm” International Conference on Cmputational Intelligence: Modelling Techniques and Applications(CIMTA,)2013.
[14] Mr. Rushi Lngadge, Ms. Snehalata S Dngre, Dr. Latesh Malik, “Class Imbalance problem in data mining: A Review”, International Journal of Computer Science and Network (IJCSN), Feb 2013 .
[15] Ruchika Mishra, Utkarsh Sharma, “Review of Image Enhancement Technique”, 7th International Journal of Engineering Research and Technology (IJERT), August 8 2013.
[16] Nour Moustafa, Jill Slay “Improving classification performance for the Minority class in highly imbalanced dataset using Boosting”, 2012 Third International Conference on Computing, Communication and Networking Technologies (ICCCNT`12).
[17] Arun Kumar M N, H S Sheshadri, “On the class of Imbalanced datasets”, International Journal of Computer Applications (IJCA), April, 2012.
[18] Tarek M Bittibssi, Gouda I Salama, Yehia Z Mehaseb and Adel E Henawy, “Image Enhancement Algorithms using FPGA”, 2012 8th International Computer Engineering Conference (ICENCO).
[19] Pradeep N, Girisha H, Sreepathi B and Karibasappa K, “Feature extraction of Mammograms”, International journal of -l of Bioinformatics research, Vol 4, 2012.
[20] G N Srinivasa, Shoba G, “Statistical Texture analysis”, Proceedings of world academy of Science and Technology-vol 36, dec 2008.
[21] Wacharasak Siriseriwan and Krung Sinapiromsaran, “ The Effective Redistribution of Imbalance Dataset: Relocating safe-level SMOTEwith Minority outcast handling”, Department of Mathematics and Computer Science, Faculty of Science, Chulalongkorn University, Bangkok 10300, Thailand, 12 Nov 2014.