An Effective Feature Extraction through Fourgram Scheme for Long Payloads in Network Intrusion Detection Systems
Abdul Rustum Ali1 , K N Brahmaji Rao2
Section:Research Paper, Product Type: Journal Paper
Volume-6 ,
Issue-10 , Page no. 296-303, Oct-2018
CrossRef-DOI: https://doi.org/10.26438/ijcse/v6i10.296303
Online published on Oct 31, 2018
Copyright © Abdul Rustum Ali, K N Brahmaji Rao . This is an open access article distributed under the Creative Commons Attribution License, which permits unrestricted use, distribution, and reproduction in any medium, provided the original work is properly cited.
View this paper at Google Scholar | DPI Digital Library
How to Cite this Paper
- IEEE Citation
- MLA Citation
- APA Citation
- BibTex Citation
- RIS Citation
IEEE Citation
IEEE Style Citation: Abdul Rustum Ali, K N Brahmaji Rao, “An Effective Feature Extraction through Fourgram Scheme for Long Payloads in Network Intrusion Detection Systems,” International Journal of Computer Sciences and Engineering, Vol.6, Issue.10, pp.296-303, 2018.
MLA Citation
MLA Style Citation: Abdul Rustum Ali, K N Brahmaji Rao "An Effective Feature Extraction through Fourgram Scheme for Long Payloads in Network Intrusion Detection Systems." International Journal of Computer Sciences and Engineering 6.10 (2018): 296-303.
APA Citation
APA Style Citation: Abdul Rustum Ali, K N Brahmaji Rao, (2018). An Effective Feature Extraction through Fourgram Scheme for Long Payloads in Network Intrusion Detection Systems. International Journal of Computer Sciences and Engineering, 6(10), 296-303.
BibTex Citation
BibTex Style Citation:
@article{Ali_2018,
author = {Abdul Rustum Ali, K N Brahmaji Rao},
title = {An Effective Feature Extraction through Fourgram Scheme for Long Payloads in Network Intrusion Detection Systems},
journal = {International Journal of Computer Sciences and Engineering},
issue_date = {10 2018},
volume = {6},
Issue = {10},
month = {10},
year = {2018},
issn = {2347-2693},
pages = {296-303},
url = {https://www.ijcseonline.org/full_paper_view.php?paper_id=3021},
doi = {https://doi.org/10.26438/ijcse/v6i10.296303}
publisher = {IJCSE, Indore, INDIA},
}
RIS Citation
RIS Style Citation:
TY - JOUR
DO = {https://doi.org/10.26438/ijcse/v6i10.296303}
UR - https://www.ijcseonline.org/full_paper_view.php?paper_id=3021
TI - An Effective Feature Extraction through Fourgram Scheme for Long Payloads in Network Intrusion Detection Systems
T2 - International Journal of Computer Sciences and Engineering
AU - Abdul Rustum Ali, K N Brahmaji Rao
PY - 2018
DA - 2018/10/31
PB - IJCSE, Indore, INDIA
SP - 296-303
IS - 10
VL - 6
SN - 2347-2693
ER -
![]() |
![]() |
![]() |
526 | 320 downloads | 248 downloads |
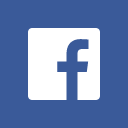
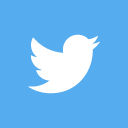
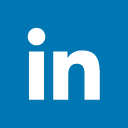
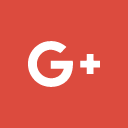
Abstract
Now a day’s security is very global issue in Network based systems. The rate of cyber terrorism has increased day by day and it put national security under risk. In addition, network attacks have caused several damages to different sectors (i.e., individuals, economy, enterprises, organizations, and governments). Network Intrusion Detection Systems are giving the solutions against these attacks. NIDS always need to improve their performance in terms of increasing the accuracy and decreasing false alarm rates. Feature selection gives the ranking to the data set attributes and it can help for selecting the most important features from the entire set of data. In the previous researches feature selection selects the irrelevant and redundant features. These are causes of increasing the processing speed and time. An efficient feature selection method eliminates dimension of data and decrease redundancy and ambiguity. In the present network intrusion detection systems working with the long payload features are not easy tasks because many machine learning algorithms can’t handle these long payload features. Some of the Network Intrusion Detection Systems are not process these long payload features. To solve this problem, a new methodology called feature extraction through Fourgram technique has been proposed. The long payload features are processed these proposed technique and prepared to be implemented in machine learning algorithms and the results were carried out on ISCX 2012 data set. The designed feature selection system has shown a very good improvement on the performance using different metrics like Accuracy, F- Measure etc.
Key-Words / Index Term
NIDS,Feature Extraction,Fourgram Scheme, Long Payload Features, Dataset, Dictionary Building
References
[1] TarfaHamed, Rozita Dara, Stefan C. Kremer, Network intrusion detection system based on recursive feature addition and bigram technique, International journal of computers & security, Vol. 73, pp.137–155, 2018.
[2] Shiravi A, Shiravi H, Tavallaee M, Ghorbani AA, “Toward developing a systematic approach to generate benchmark datasets for intrusion detection”, International journal of Computer Security, Vol.3,No.31,pp.357-374,2012.
[3] Garcia LP, de Carvalho AC, Lorena AC, “Effect of label noise in the complexity of classification problems”,International journal of Neurocomputing”, Vol.19,No.16,pp.108-119,2015.
[4] Beigi EB, Jazi HH, Stakhanova N, Ghorbani AA,“Towards effective feature selection in machine learningbased bonnet detection approaches”, In the proceedings of the 2014 IEEE conference on Communications and Network Security,pp.247–255, 2014.
[5] Bolon-Canedo V, Snchez-Maroo N, Alonso-Betanzos A, Bentez J, Herrera F, “A review of microarray datasets and applied feature selection methods”, International journal of Information Sciences”,Vol.5,No.42,pp.111-135,2014.
[6] Beniwal S, Arora J, “Classification and feature selection techniques in data mining”, International journal of engineering and Research and technology, Vol.1, No.6, pp.1-6, 2012.
[7] Fahad A, Tari Z, Khalil I, Habib I, Alnuweiri H, “Toward an efficient and scalable feature selection approach for internet traffic classification”, International journal of Computer Networks”,Vol.9.No.57,pp.2040-2057,2013.
[8] Aghdam MH, Kabiri P, “Feature selection for intrusion detection system using ant colony optimization”, International journal of network security, Vol.3, No.18, pp.420-432, 2016.
[9] Bolón-Canedo V, Sánchez-Maroño N, Alonso-Betanzos, “A review of feature selection methods on synthetic data”, International journal of information systems, Vol.3, No. 34, pp.483-519, 2013.
[10] Sahu SK, Sarangi S, Jena SK, “A detail analysis on intrusion detection datasets”In the proceedings of the 2014 IEEE International conference on Advance Computing Conference (IACC), pp.1348-1353, 2014.
[11] Mancini LV, Di Pietro R, “Intrusion Detection Systems”, International journal of Springer, Vol.5, No.9,pp.513-524, 2008.
[12] Ambusaidi MA, He X, Nanda P, Tan Z, “Building an intrusion detection system using a filter-based feature selection algorithm”, International journal of Computer science and applications, Vol.10, No.65, pp.2986-2998, 2016.
[13] Abou El Kalam A., Gad El Rab M., and Deswarte Y, “A model-driven approach for experimental evaluation of intrusion detection systems, International journal of Security Communication Networks, Vol.7, No.14, pp.1955–1973, 2014.
[14] Mell P, Hu V, Lipmann R, Haines J, Zissman M, “An overview of issues in testing intrusion detection systems”, Technical Report, NIST IR 7007, National Institute of Standard and Technology, USA, 2003.
[15] NiccolòCascarano, Luigi Ciminiera, FulvioRisso, “Improving cost and accuracy of DPI traffic classifiers”, In Proceedings of the ACM Symposium on Applied Computing (SAC `10) ACM, New York, NY, USA, pp.641-646, 2010.
[16] Laurent Bernaille , Renata Teixeira , Ismael Akodkenou,Augustin Soule , KaveSalamatian, “Traffic classification on the fly”, Journal of ACM SIGCOMM Computer Communications, Vol.36, No.2, pp.145-158, 2006.
[17] Zhang M, Wang L, Jajodia S, Singhal A, Albanese M, “Networkdiversity: a security metric for evaluating the resilience ofnetworks against zero-day attacks”, International journal of transformation of information science Vol.5, No.11, pp.1071–1086, 2016.
[18] Chang C-C, Lin C-J. LIB, “SVM: a library for support vector machines”, International journal of Intelligent System Technologies, Vol.3, No.2, pp.215-226, 2011.