Fast and Effective Method for the Detection of Brain Tumor using Chebyshev Harmonic Fourier Moments
A. Prashar1 , R. Upneja2
Section:Research Paper, Product Type: Journal Paper
Volume-6 ,
Issue-10 , Page no. 312-316, Oct-2018
CrossRef-DOI: https://doi.org/10.26438/ijcse/v6i10.312316
Online published on Oct 31, 2018
Copyright © A. Prashar, R. Upneja . This is an open access article distributed under the Creative Commons Attribution License, which permits unrestricted use, distribution, and reproduction in any medium, provided the original work is properly cited.
View this paper at Google Scholar | DPI Digital Library
How to Cite this Paper
- IEEE Citation
- MLA Citation
- APA Citation
- BibTex Citation
- RIS Citation
IEEE Citation
IEEE Style Citation: A. Prashar, R. Upneja, “Fast and Effective Method for the Detection of Brain Tumor using Chebyshev Harmonic Fourier Moments,” International Journal of Computer Sciences and Engineering, Vol.6, Issue.10, pp.312-316, 2018.
MLA Citation
MLA Style Citation: A. Prashar, R. Upneja "Fast and Effective Method for the Detection of Brain Tumor using Chebyshev Harmonic Fourier Moments." International Journal of Computer Sciences and Engineering 6.10 (2018): 312-316.
APA Citation
APA Style Citation: A. Prashar, R. Upneja, (2018). Fast and Effective Method for the Detection of Brain Tumor using Chebyshev Harmonic Fourier Moments. International Journal of Computer Sciences and Engineering, 6(10), 312-316.
BibTex Citation
BibTex Style Citation:
@article{Prashar_2018,
author = {A. Prashar, R. Upneja},
title = {Fast and Effective Method for the Detection of Brain Tumor using Chebyshev Harmonic Fourier Moments},
journal = {International Journal of Computer Sciences and Engineering},
issue_date = {10 2018},
volume = {6},
Issue = {10},
month = {10},
year = {2018},
issn = {2347-2693},
pages = {312-316},
url = {https://www.ijcseonline.org/full_paper_view.php?paper_id=3023},
doi = {https://doi.org/10.26438/ijcse/v6i10.312316}
publisher = {IJCSE, Indore, INDIA},
}
RIS Citation
RIS Style Citation:
TY - JOUR
DO = {https://doi.org/10.26438/ijcse/v6i10.312316}
UR - https://www.ijcseonline.org/full_paper_view.php?paper_id=3023
TI - Fast and Effective Method for the Detection of Brain Tumor using Chebyshev Harmonic Fourier Moments
T2 - International Journal of Computer Sciences and Engineering
AU - A. Prashar, R. Upneja
PY - 2018
DA - 2018/10/31
PB - IJCSE, Indore, INDIA
SP - 312-316
IS - 10
VL - 6
SN - 2347-2693
ER -
![]() |
![]() |
![]() |
442 | 278 downloads | 268 downloads |
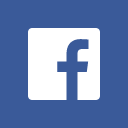
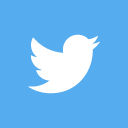
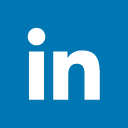
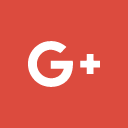
Abstract
Brain tumor is a serious type of disorder which is caused by the abnormal cells formation within the brain. The identification of brain tumor and further analysis from the Magnetic Resonance Imaging (MRI) is a vigorous process and in this paper fast and effective method is used for the tumor detection by using Chebyshev Harmonic Fourier Moments (CHFMs) on segmented magnetic resonance brain images. The proposed method is free from any overflow situations as it does not involve any factorial term and also free from underflow situations as no power terms are involved. Before the segmentation process, the feature set is extracted by using 2D Continuous Wavelet Transform (2D-CWT). Asymmetry in the MR brain image is analyzed by using CHFMs on each of the tissues segmented in the head. Once the presence of asymmetry is confirmed, it leads us to the diagnosis of the tumor. After the presence of tumor, the region of tumor is extracted by using Polar Harmonic Transforms (PHTs) as these transforms are found to be good descriptors in the field of image analysis and impose less computational complexity due to the absence of any factorial term in the calculation of radial kernels. The effectiveness of the proposed method is analyzed by doing experiments on 35 MR brain images with tumor and 65 normal MR brain images. It is observed that that the proposed method and technique is successful in 97% cases.
Key-Words / Index Term
Tumor detection, Chebyshev Harmonic Fourier Moments, Polar Harmonic Transforms, Segmentation
References
[1] M. R. Teague, “Image analysis via the general theory of moments,” Journal of the Optical Society of America, vol. 70, pp. 920-930, 1980.
[2] A. B. Bhatia and E. Wolf, “On the circle polynomials of Zernike and related orthogonal sets,” Proc. of the Cambridge Philosophical Society, vol. 50, pp. 40-48, 1954.
[3] Y. Sheng and L. Shen, “Orthogonal Fourier Mellin moments for invariant pattern recognition,” J. Opt. Soc. Am. A, vol. 11, Issue 6, pp. 1748-1757, 1994.
[4] R. Upneja and C. Singh, “Fast computation of Jacobi-Fourier moments for invariant image recognition,” Pattern Recognition, vol. 48, Issue 5, pp. 1836-1843, 2015.
[5] Y. Jiang, Z. Ping, and L. Gao, “A fast algorithm for computing Chebyshev-Fourier moments,” Proc. 2010 International Conference on Future Information Technology and Management Engineering (FITME), pp. 425 – 428, 9-10, 2010.
[6] Z. Dokur, and T. Olmez, “Classification of Magnetic Resonance Images using a novel neural network”, Proceedings of the IEEE-EMBS Asia Pacific conference on biomedical engineering, pp. 242-243, 2000.
[7] W. Qian, L. Li, and P. Clarke, “Image feature extraction for mass detection in digital mammography: Influence of wavelet analysis”, Medical Physics, vol. 26, Issue 3, pp. 402-408, 1999.
[8] E.J. Feleppa, A. Kalisz, J.B.S. Melgar, and F.L. Lizzi, “Typing of Prostate Tissue by Ultrasonic Spectrum Analysis”, IEEE Transactions on Ultrasonics, ferroelectrics, and frequency control, vol. 43, Issue 4, pp. 609-619, 1996.
[9] A. Jose, S. Ravi, and M. Sambath, “Brain Tumor Segmentation Using K-Means Clustering and Fuzzy C-Means Algorithms and Its Area Calculation”, International Journal of Innovative Research in Computer and Communication Engineering, vol. 2, pp. 3496-3501, 2014.
[10] E.A. Maksaud, M. Emlogy, R. Al-Awadi, “Brain Tumor Segmentation based on a Hybrid Clustering Technique”, Science Direct: Egyptian Informatics Journal, vol. 16, pp. 71-81, 2015.
[11] M. Kanimozhi, and C.H. Bindu, “Brain MR Image Segmentation Using Self Organizing Map”, International Journal of Advanced Research in Computer and Communication Engineering, vol. 2, pp. 3968-3973, 2013.
[12] Z. Ping, R. Wu, and Y. Sheng, “Image description with Chebyshev-Fourier moments,” J. Opt. Soc. Am. A, vol. 19, Issue 9, pp. 1748-1754, 2002.
[13] R. Upneja, C. Singh and A. Prashar, “Fast Computation of Chebyshev- Harmonic Fourier moments”, LNIT, vol. 3, Issue 2, pp. 60-64, 2015.
[14] C. Singh and E. Walia, “Algorithms for fast computation of Zernike moments and their numerical stability,” Image and Vision Computing, vol. 29, Issue 4, pp. 251-259, 2011.