WILD ANIMAL DETECTION USING MULTI-CLUSTER FEATURE SELECTION
S. Keerthana1 , E. Mary Shyla2
Section:Research Paper, Product Type: Journal Paper
Volume-6 ,
Issue-10 , Page no. 628-632, Oct-2018
CrossRef-DOI: https://doi.org/10.26438/ijcse/v6i10.628632
Online published on Oct 31, 2018
Copyright © S. Keerthana, E. Mary Shyla . This is an open access article distributed under the Creative Commons Attribution License, which permits unrestricted use, distribution, and reproduction in any medium, provided the original work is properly cited.
View this paper at Google Scholar | DPI Digital Library
How to Cite this Paper
- IEEE Citation
- MLA Citation
- APA Citation
- BibTex Citation
- RIS Citation
IEEE Style Citation: S. Keerthana, E. Mary Shyla, “WILD ANIMAL DETECTION USING MULTI-CLUSTER FEATURE SELECTION,” International Journal of Computer Sciences and Engineering, Vol.6, Issue.10, pp.628-632, 2018.
MLA Style Citation: S. Keerthana, E. Mary Shyla "WILD ANIMAL DETECTION USING MULTI-CLUSTER FEATURE SELECTION." International Journal of Computer Sciences and Engineering 6.10 (2018): 628-632.
APA Style Citation: S. Keerthana, E. Mary Shyla, (2018). WILD ANIMAL DETECTION USING MULTI-CLUSTER FEATURE SELECTION. International Journal of Computer Sciences and Engineering, 6(10), 628-632.
BibTex Style Citation:
@article{Keerthana_2018,
author = {S. Keerthana, E. Mary Shyla},
title = {WILD ANIMAL DETECTION USING MULTI-CLUSTER FEATURE SELECTION},
journal = {International Journal of Computer Sciences and Engineering},
issue_date = {10 2018},
volume = {6},
Issue = {10},
month = {10},
year = {2018},
issn = {2347-2693},
pages = {628-632},
url = {https://www.ijcseonline.org/full_paper_view.php?paper_id=3073},
doi = {https://doi.org/10.26438/ijcse/v6i10.628632}
publisher = {IJCSE, Indore, INDIA},
}
RIS Style Citation:
TY - JOUR
DO = {https://doi.org/10.26438/ijcse/v6i10.628632}
UR - https://www.ijcseonline.org/full_paper_view.php?paper_id=3073
TI - WILD ANIMAL DETECTION USING MULTI-CLUSTER FEATURE SELECTION
T2 - International Journal of Computer Sciences and Engineering
AU - S. Keerthana, E. Mary Shyla
PY - 2018
DA - 2018/10/31
PB - IJCSE, Indore, INDIA
SP - 628-632
IS - 10
VL - 6
SN - 2347-2693
ER -
![]() |
![]() |
![]() |
473 | 405 downloads | 215 downloads |
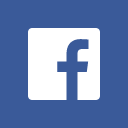
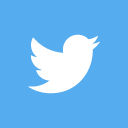
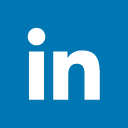
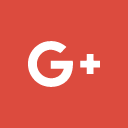
Abstract
Wild animal detection helps wildlife researchers to analyze and study wild animal habitat and behavior. Discriminative Feature-oriented Dictionary Learning (DFDL) was utilized for learning discriminative features of positive images that have animals present in positive class, in addition of negative images that do not have animals present in that class. But, this approach has low performance for detection of visual wild animals. Hence, in this paper, Multi-Cluster Feature Selection (MCFS) is proposed for unsupervised feature selection and wild animal detection. Those features are chosen, which the multi-cluster structure of the data is well preserved. Based on spectral analysis approaches, the proposed method suggests a principled manner for calculating the correlations among various features without label information. Thus, the proposed technique handles the data with multiple cluster structure. The experimental results show that the proposed approach provides the better results.
Key-Words / Index Term
Dictionary Learning, Multi-Cluster Feature Selection, Wild animal detection, Spectral analysis
References
[1] Neff, M. G., Cheng, S. N., & Johnson, T. L. (2011). U.S. Patent No. 7,999,849. Washington, DC: U.S. Patent and Trademark Office.
[2] KaewTraKulPong, P., & Bowden, R. (2002). “An improved adaptive background mixture model for real-time tracking with shadow detection”. In Video-based surveillance systems (pp. 135-144). Springer, Boston, MA.
[3] Bhat, K. S., Saptharishi, M., & Khosla, P. K. (2000). “Motion detection and segmentation using image mosaics”. InMultimedia and Expo, 2000. ICME 2000. 2000 IEEE International Conference on (Vol. 3, pp. 1577-1580). IEEE.
[4] Reilly, V., Idrees, H., & Shah, M. (2010, September). “Detection and tracking of large number of targets in wide area surveillance”. In European conference on computer vision (pp. 186-199). Springer, Berlin, Heidelberg.
[5] Wang, Y., Zhang, Z., & Wang, Y. (2012, December). “Moving object detection in aerial video”. In Machine Learning and Applications (ICMLA), 2012 11th International Conference on(Vol. 2, pp. 446-450). IEEE.
[6] Gupta, P., & Verma, G. K. “Wild Animal Detection using Discriminative Feature-oriented Dictionary Learning”.
[7] Fang, Y., Du, S., Abdoola, R., Djouani, K., & Richards, C. (2016). “Motion based animal detection in aerial videos.”Procedia Computer Science, 92, 13-17.
[8] Nguyen, H., Maclagan, S. J., Nguyen, T. D., Nguyen, T., Flemons, P., Andrews, K., ... & Phung, D. (2017, October). “Animal recognition and identification with deep convolutional neural networks for automated wildlife monitoring”. In Data Science and Advanced Analytics (DSAA), 2017 IEEE International Conference on (pp. 40-49). IEEE.
[9] Parham, J., Stewart, C., Crall, J., Rubenstein, D., Holmberg, J., & Berger-Wolf, T. (2018, March). “An Animal Detection Pipeline for Identification”. In 2018 IEEE Winter Conference on Applications of Computer Vision (WACV) (pp. 1075-1083). IEEE.
[10] Zhu, C., Li, T. H., & Li, G. (2017, October). “Towards automatic wild animal detection in low quality camera-trap images using two-channeled perceiving residual pyramid networks”. InComputer Vision Workshop (ICCVW), 2017 IEEE International Conference on (pp. 2860-2864). IEEE.
[11] Zhang, T., Wiliem, A., Hemsony, G., & Lovell, B. C. (2015, April). “Detecting kangaroos in the wild: the first step towards automated animal surveillance”. In ICASSP (pp. 1961-1965).
[12] Jaskó, G., Giosan, I., & Nedevschi, S. (2017, September). “Animal detection from traffic scenarios based on monocular color vision”. In Intelligent Computer Communication and Processing (ICCP), 2017 13th IEEE International Conference on (pp. 363-368). IEEE.