A Survey on Cross - Domain Opinion Mining
V. Manimekalai1 , S. Gomathi @ Rohini2
Section:Survey Paper, Product Type: Journal Paper
Volume-6 ,
Issue-10 , Page no. 792-796, Oct-2018
CrossRef-DOI: https://doi.org/10.26438/ijcse/v6i10.792796
Online published on Oct 31, 2018
Copyright © V. Manimekalai, S. Gomathi @ Rohini . This is an open access article distributed under the Creative Commons Attribution License, which permits unrestricted use, distribution, and reproduction in any medium, provided the original work is properly cited.
View this paper at Google Scholar | DPI Digital Library
How to Cite this Paper
- IEEE Citation
- MLA Citation
- APA Citation
- BibTex Citation
- RIS Citation
IEEE Style Citation: V. Manimekalai, S. Gomathi @ Rohini, “A Survey on Cross - Domain Opinion Mining,” International Journal of Computer Sciences and Engineering, Vol.6, Issue.10, pp.792-796, 2018.
MLA Style Citation: V. Manimekalai, S. Gomathi @ Rohini "A Survey on Cross - Domain Opinion Mining." International Journal of Computer Sciences and Engineering 6.10 (2018): 792-796.
APA Style Citation: V. Manimekalai, S. Gomathi @ Rohini, (2018). A Survey on Cross - Domain Opinion Mining. International Journal of Computer Sciences and Engineering, 6(10), 792-796.
BibTex Style Citation:
@article{Manimekalai_2018,
author = {V. Manimekalai, S. Gomathi @ Rohini},
title = {A Survey on Cross - Domain Opinion Mining},
journal = {International Journal of Computer Sciences and Engineering},
issue_date = {10 2018},
volume = {6},
Issue = {10},
month = {10},
year = {2018},
issn = {2347-2693},
pages = {792-796},
url = {https://www.ijcseonline.org/full_paper_view.php?paper_id=3102},
doi = {https://doi.org/10.26438/ijcse/v6i10.792796}
publisher = {IJCSE, Indore, INDIA},
}
RIS Style Citation:
TY - JOUR
DO = {https://doi.org/10.26438/ijcse/v6i10.792796}
UR - https://www.ijcseonline.org/full_paper_view.php?paper_id=3102
TI - A Survey on Cross - Domain Opinion Mining
T2 - International Journal of Computer Sciences and Engineering
AU - V. Manimekalai, S. Gomathi @ Rohini
PY - 2018
DA - 2018/10/31
PB - IJCSE, Indore, INDIA
SP - 792-796
IS - 10
VL - 6
SN - 2347-2693
ER -
![]() |
![]() |
![]() |
419 | 293 downloads | 266 downloads |
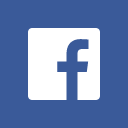
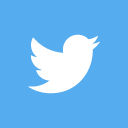
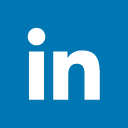
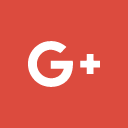
Abstract
The social network growth is increased and the interest of people in analyzing reviews and opinions for products before buy them. In this regarding research communities, academia, public and service industries are working rigorously on sentiment analysis, also known as, opinion mining, to extract and analyze public mood and views. Analyzing the sentiments in massive user-generated online data, such as product reviews and micro blogs, has become a hot research topic. Sentiment analysis is widely known as a domain dependent problem. In this paper presents a rigorous survey on cross domain sentiment analysis, challenges for social media, then identified problems in different domains usually have different sentiment expressions and a general sentiment classifier is not suitable for all domains. The main problem is the selection of sentiment from huge volume of opinionated data for different kinds of event which is available in the social networks, but there exist a huge difficulty in predicting the accurate outcome of the event at cross domain. A natural solution to this problem is to train a domain-specific sentiment classifier for each target domain. However, the labeled data in target domain is usually insufficient, and it is costly and time-consuming to annotate enough samples.
Key-Words / Index Term
Data Mining, Opinion Mining, Machine Learning, Cross - Domain Sentiment Analysis, SentiWordNet
References
[1] S. J. Pan, X. Ni, J.-T. Sun, Q. Yang, and Z. Chen, ‘‘Cross-domain sentiment classification via spectral feature alignment,’’ in Proc. 19th Int. Conf. World.Wide Web, vol. 10.2010, p. 751.
[2] C. Lin, Y. Lee, C. Yu, and H. Chen, ‘‘Exploring ensemble of models in taxonomy-based cross-domain sentiment classification,’’ in Proc. 23rd ACM Int. Conf. Conf. Inf. Knowl. Manage.-(CIKM), 2014, pp. 1279 –1288.
[3] J. Blitzer, R. McDonald, and F. Pereira, ‘‘Domain adaptation with structural correspondence learning,’’ in Proc. Conf. Empirical Methods Natural Lang. Process., 2006, pp. 120–128.
[4] J. Blitzer, M. Dredze, and F. Pereira, ‘‘Biographies, bollywood, boom-boxes and blenders: Domain adaptation for sentiment classification,’’ in Proc. ACL, vol. 7. 2007, pp. 440–447.
[5] J. Yu and J. Jiang, ‘‘Learning sentence embeddings with auxiliary tasks for cross-domain sentiment classification,’’ in Proc. Conf. Empirical Methods Natural Lang. Process., 2016, pp. 236–246.
[6] D. M. Blei and M. I. Jordan, ‘‘Modeling annotated data,’’ in Proc. 26th Annu. Int. ACM SIGIR Conf. Res. Develop. Inf. Retrieval, 2003, pp. 127–134.
[7] Pang, Bo, Lillian Lee, and ShivakumarVaithyanathan. "Thumbs up?: sentiment classification using machine learning techniques." Proceedings of the ACL-02 conference on Empirical methods in natural language processing-Volume 10.Association for Computational Linguistics, 2002.
[8] Turney, Peter D. "Thumbs up or thumbs down?: semantic orientation applied to unsupervised classification of reviews." Proceedings of the 40th annual meeting on association for computational linguistics.Association for Computational Linguistics, 2002.
[9] Yan Dang; Yulei Zhang; Hsinchun Chen, "A Lexicon-Enhanced Method for Sentiment Classification: An Experiment on Online Product Reviews, Intelligent Systems, IEEE , vol.25, no.4, pp.46,53, July-Aug.2010 doi: 10.1109/MIS.2009.105
[10] Hung, Chihli, and Hao-Kai Lin. "Using objective words in SentiWordNet to improve word-of-mouth sentiment classification." IEEE Intelligent Systems 28.2 (2013): 0047-54.
[11] Bhaskar, J.; Sruthi, K.; Nedungadi, P., "Enhanced sentiment analysis of informal textual communication in social media by considering objective words and intensifiers," Recent Advances and Innovations in Engineering (ICRAIE), 2014 , vol., no., pp.1,6, 9-11 May 2014 doi: 10.1109/ICRAIE.2014.6909220.
[12] Muhammad faheem Khan, Aurangzeb and khairullah khan efficient word sense disambigutionteqnique for sentence level sentiment classification of online review‖ Sci.Int(Lahore).25(4),2013.
[13] Bollegala, D.; Weir, D.; Carroll, J., "Cross-Domain Sentiment Classification Using a Sentiment Sensitive Thesaurus," Knowledge and Data Engineering, IEEE Transactions on , vol.25, no.8, pp.1719,1731, Aug. 2013 doi: 10.1109/TKDE.2012.103
[14] A. Esuli, F. Sebastiani, SENTIWORDNET: a publicly available lexical resource for opinion mining, in: Proceedings of the 5th Conference on Language Resources and Evaluation LREC-06, Genoa, Italy, 2006, pp. 417–422.
[15] S. Baccianella, A. Esuli, F. Sebastiani, SENTIWORDNET 3.0: an enhanced lexical resource for sentiment analysis and opinion mining, in: Proceedings of LREC10, Malta, 2010, pp. 2200–2204.
[16] A. Moreo, M. Romero, J.L. Castro, J.M. Zurita, Lexicon-based commentsoriented news sentiment analyzer system, Expert Syst. Appl. 39 (2012) 9166– 9180.
[17] H. Cho et al., Data-driven integration of multiple sentiment dictionaries for lexicon-based sentiment classification of product reviews, Knowl.-Based Syst. 71 (2014) 61–71.
[18] M. Hu, B. Liu, Mining and summarizing customer reviews, in: Proceedings of Tenth ACM SIGKDD International Conference on Knowledge Discovery and Data Mining, ACM, 2004, pp. 168–177.
[19] F.Å. Nielsen, A New ANEW: Evaluation of a Word List for Sentiment Analysis in Microblogs, 2011.
[20] M. Taboada, J. Brooke, M. Tofiloski, Lexicon-based methods for sentiment analysis, Comput.Linguist. 37 (2) (2011) 267–307.
[21] A. Weichselbraun, S. Gindl, A. Scharl, Extracting and grounding contextualized sentiment lexicons, IEEE Intell. Syst. 28 (2) (2013) 39–46.
[22] D. Bollegala, D. Weir, J. Carroll, Cross-domain sentiment classification using a sentiment sensitive thesaurus, IEEE Trans. Knowl. Data Eng. 25 (8) (2013).
[23] E. Riloff, J. Wiebe, Learning extraction patterns for subjective expressions, in: Proceedings of the 2003 Conference on Empirical Methods in Natural Language Processing (EMNLP 2003), 2003, pp. 105–112.
[24] P.J. Stone, E.B. Hunt, A computer approach to content analysis: studies using the general inquirer system, in: Proceedings of the Spring Joint Computer Conference (AFIPS 1963), 1963, pp. 241–256.
[25] E. Cambria, R. Speer, C. Havasi, A. Hussain, SenticNet: a publicly available semantic resource for opinion mining, in: AAAI Fall Symposium: Commonsense Knowledge, vol. 10, p. 02, 2010.
[26] J.C. de Albornoz, L. Plaza, P. Gervas, Sentisense: an easily scalable conceptbased affective lexicon for sentiment analysis, in: Proceedings of the 8th International Conference on Language Resources and Evaluation (LREC 2012), 2012, pp. 3562–3567.
[27] S. Cerini, V. Compagnoni, A. Demontis, M. Formentelli, C. Gandini, Micro- 862 WNOp: a gold standard for the evaluation of automatically compiled lexical resources for opinion mining, in: A. Sanso (Ed.), Language Resources and Linguistic Theory, Franco Angeli, 2007, pp. 200–210.
[28] S. Tan, X. Cheng, Y. Wang, H. Xu, Adapting naive bayes to domain adaptation for sentiment analysis, in: M. Boughanem et al. (Eds.), ECIR 2009, LNCS 5478, 2009, pp. 337–349.
[29] K. Bollacker, C. Evans, P. Paritosh, T. Sturge, J. Taylor, FreeBase: a collaboratively created graph database for structuring human knowledge, in: Proceedings of the 2008 ACM SIGMOD International Conference on Management of Data, ACM, 2008, pp. 1247–1250.
[30] C. Bizer, J. Lehmann, G. Kobilarov, S. Auer, C. Becker, R. Cyganiak, S. Hellmann, DBpedia-A crystallization point for the web of data, Web Semant.: Sci., Serv. Agents World Wide Web 7 (3) (2009) 154–165.
[31] J. Blitzer, M. Dredze, F. Pereira, Biographies, bollywood, boom-boxes and blenders: domain adaptation for sentiment classification, in: Proceedings of the 45th Annual Meeting of the Association for Computational Linguistics, ACL’07, vol. 7, 2007, pp. 187–205 (13, 29).
[32] B. Pang, L. Lee, A sentiment education: sentiment analysis using subjectivity summarization based on minimum cuts, in: Proceedings of the 42nd Annual Meeting on Association for Computational Linguistics, July 2004, p. 271.
[33] Z.Yan et al, EXPRS: an extended page rank method for product feature extraction from online consumer reviews, Inform Manage (2015), http://dx.doi.org/10.1016/j.imm.2015.02.002
[34] A.Hendari, Mhd. A.Tavakoli, N.Salim, Z.Hendari, Detection of Review Spam:aSurvvey, Expert Syst. Appl. 42 (7) (2015) 3634 – 3642.
[35] JyotiS.Deshmukh a, Amiya Kumar Tripathy, Entropy based Classifier for Cross – Domain Opinion Mining, Applied Computing and Informatics 144 (2017) 55-64.