Performance Comparison of Forecasting on Solar Plant Generation Data
Sukhpal Kaur1 , Madhuchanda Rakshit2
Section:Research Paper, Product Type: Journal Paper
Volume-6 ,
Issue-10 , Page no. 827-834, Oct-2018
CrossRef-DOI: https://doi.org/10.26438/ijcse/v6i10.827834
Online published on Oct 31, 2018
Copyright © Sukhpal Kaur, Madhuchanda Rakshit . This is an open access article distributed under the Creative Commons Attribution License, which permits unrestricted use, distribution, and reproduction in any medium, provided the original work is properly cited.
View this paper at Google Scholar | DPI Digital Library
How to Cite this Paper
- IEEE Citation
- MLA Citation
- APA Citation
- BibTex Citation
- RIS Citation
IEEE Style Citation: Sukhpal Kaur, Madhuchanda Rakshit, “Performance Comparison of Forecasting on Solar Plant Generation Data,” International Journal of Computer Sciences and Engineering, Vol.6, Issue.10, pp.827-834, 2018.
MLA Style Citation: Sukhpal Kaur, Madhuchanda Rakshit "Performance Comparison of Forecasting on Solar Plant Generation Data." International Journal of Computer Sciences and Engineering 6.10 (2018): 827-834.
APA Style Citation: Sukhpal Kaur, Madhuchanda Rakshit, (2018). Performance Comparison of Forecasting on Solar Plant Generation Data. International Journal of Computer Sciences and Engineering, 6(10), 827-834.
BibTex Style Citation:
@article{Kaur_2018,
author = {Sukhpal Kaur, Madhuchanda Rakshit},
title = {Performance Comparison of Forecasting on Solar Plant Generation Data},
journal = {International Journal of Computer Sciences and Engineering},
issue_date = {10 2018},
volume = {6},
Issue = {10},
month = {10},
year = {2018},
issn = {2347-2693},
pages = {827-834},
url = {https://www.ijcseonline.org/full_paper_view.php?paper_id=3107},
doi = {https://doi.org/10.26438/ijcse/v6i10.827834}
publisher = {IJCSE, Indore, INDIA},
}
RIS Style Citation:
TY - JOUR
DO = {https://doi.org/10.26438/ijcse/v6i10.827834}
UR - https://www.ijcseonline.org/full_paper_view.php?paper_id=3107
TI - Performance Comparison of Forecasting on Solar Plant Generation Data
T2 - International Journal of Computer Sciences and Engineering
AU - Sukhpal Kaur, Madhuchanda Rakshit
PY - 2018
DA - 2018/10/31
PB - IJCSE, Indore, INDIA
SP - 827-834
IS - 10
VL - 6
SN - 2347-2693
ER -
![]() |
![]() |
![]() |
537 | 252 downloads | 255 downloads |
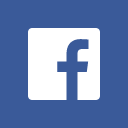
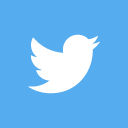
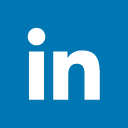
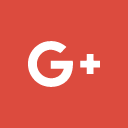
Abstract
The purpose of this paper is to compare the forecasting performances of daily generation of a solar plant by utilizing the autoregressive time series models. As the demand for energy is increasing frequently all over the world, the proper integration of solar energy and its accurate predictions become necessary for our society for better planning and distribution of energy. In this study, we compare our solar energy time series data with Autoregressive Integrated Moving Average with Explanatory Variable (ARIMAX) and Vector Autoregressive (VAR) time series models for analyzing our solar plant data separately and at last conclusion is made on the better performance of these two methods. Moreover for VAR model effects of various variables are tested for maximum production of solar power. For evaluating the accuracy performance of our forecasted data, we use Mean Absolute Error (MAE), Mean Absolute Scaled Error (MASE) and Root Mean Square Error (RMSE) measurements.
Key-Words / Index Term
ARIMAX, MAE, MASE, RMSE, Solar Plant Generation, VAR
References
[1] A. Jalalkamali, M. Moradi and N. Moradi, “Application of several artificial intelligence models and ARIMAX model for forecasting drought using Standardized Precipitation Index”, Int. J. Environ. Sci. Technology, Vol. 12, pp. 1201-1210, 2015.
[2] Billy M. Williams, “Multivariate vehicular traffic flow prediction, Evaluation of ARIMAX modelling”, Journal of the transportation research board ,pp. 194-200, January 2001
[3] B. Yogarajah, C. elankumaran and R. Vigneswaran, “Application of ARIMAX Model for forecasting Paddy Production in Trincomalee District in Sri Lanka”, Proceeding of third international Symposium, Vol. 24, pp. 2-3, July 2013
[4] Christopher Bennett, Rodney A. Stewart and Junwei Lu, “ Autoregressive with Exogenous Variables and Neural Network Short- Term Load Forecast Models for Residential Low Voltage Distribution Networks”, Energies, Vol. 7, pp. 2938-2960, 2014
[5] Ding N, Besanger Y, “ Time series method for short – term load forecastingusing smart metering in distribution systems, “ International Proceeding of the IEEE Trondheim Power Tech, pp. 1-6, 2011
[6] D.K.Chaturvedi and Isha, “ Solar Power Forecasting: A Review”,International Journal of Computer Applications”, Vol.145, Issue. 6, July 2016
[7] El Mostafa Bentour, “ A ranking of VAR and structural models in forecasting”,http://mpra.ub.uni-muenchen.de/61502/, January 2015
[8] Herui Cui and Xu Peng , “Short –Term City Electric Load Forecasting with Considering Temprature Effects: An Improved ARIMAX Model”, Hindawi Pubishing Corporation, Mathematical Problem in Engineering , Volume 2015
[9] Gulam Ali “Cointegration VAR and VECM and ARIMAX Econometric Approaches for water quality Variaties” Journal of Statistical and Econometric Methods, Vol. 4, Issue. 1, pp.1-38, 2015
[10] Harendra Kumar Yadav, Vinay Kumar Yadav and Vijay Kumar, “ Potential of solar energy in India: A Review” International Advanced Research Journal of Science, Engineering and Technology, Vol. 2, Issue 1, May 2015
[11] Jabar H. Yousif and Hussein A. Kazem, “ Modeling of Daily Solar Energy System Prediction using Soft Cmputing Methods for Oman”, Research Journal of Applied Sciences, Engineering and Technology 13(3): 237-244, 2016
[12] Jai Singh Arya, Aadesh Kumar Arya and Sanjeev Aggarwal, “ Recent Trends in Solar Energy Generation”, Association of Computer Electronics and Electrical Engineers, 2013
[13] Javed Iqbal, “Forecasting Methods: A Comparative Analysis”, Proc. Eight Stat. Sem. 2001, K.U, MPRA Paper No.23856, posted29. Auguest 2013
[14] Jing Fan, Rui Shan and Xiaoqin Cao & Peiliang Li, “The Analysis to Tertiary- industry with ARIMAX Model”, Journal of Mathematics Research, Vol.1, No.2, September 2009
[15] Mohamed Abuella and Badrul Chowdhary, “Solar power Forecasting Using Support Vector Regression” in proceeding of the American Society for Engineering Management 2016
[16] Paul S. P. Cowperwait and Andrew V. Metcalfe,“Introductory Time Series with R” ,Springer, 2008
[17] Phillips, P. C. B.; Ouliaris, S. (1990). "Asymptotic Properties of Residual Based Tests for Cointegration".Econometrica. Vol.58, Issue.1, pp. 165–193.
[18] Renny Elfira Wulansari, Setiawan and Suhartono, “A Comparison of Forecasting Performance of Seasonal ARIMAX and Hybrid Seasonal ARIMAX –ANN of Surabaya’s Currency Circulation Data”, International Journal of Management and Applied Science , Vol.2, Issue-10,Oct-2016
[19] Robert H. Shumway and David S. Stoffer, “Time Series Analysis and Its Applications With R Examples”,Second Edition, Springer text in Statistics, 2005
[20] S.M. Husnain Bokhari and Mete Feridun, “Forecasting inflation through Econometric models : An Empirical Study on Pakistani Data”, Vol. 7, Issue. 1, pp.39-47, 2006
[21] Stijin de Waele and Piet M.T. Broerson, “ Order Selection for Vector Autoregressive Model” ,IEEE transactions on signal processing, Vol. 51, Issue. 2, February, 2003
[22] Uyodhu Amekauma Victor- Edema and Prof Isaac Didi Essi , “Autoregressive Integrated Moving Average with Exogenous Variable(ARIMAX) Model for Nigerian Non Oil Export”, European Journal of Business and Management, Vol. 8, Issue. 36, 2016
[23] Wiwik Anggraeni, Kuntoro Boga Andri, Sumaryanto and Faizal Mahananto, “The performance of ARIMAX Model and Vector Autoregressive (VAR) Modelforecasting strategic commodity price in Indonesia”, Procedia Computer Science, pp.189-196, November 2017
[24] Wiwik Anggraeni, Retno Aulia Vinarti and Yuni Dwi Kurniawati, “Performance Comparisions Between Arima and arimax Method in Moslem Kids Clothes Demand Forecasting: Case Study”, Procedia Computer Science, Vol.72, pp.630-637, 2015