Denoising of skull stripped brain tumor MR images
Akshath M J1 , H S Sheshadri2
Section:Research Paper, Product Type: Journal Paper
Volume-6 ,
Issue-11 , Page no. 323-329, Nov-2018
CrossRef-DOI: https://doi.org/10.26438/ijcse/v6i11.323329
Online published on Nov 30, 2018
Copyright © Akshath M J, H S Sheshadri . This is an open access article distributed under the Creative Commons Attribution License, which permits unrestricted use, distribution, and reproduction in any medium, provided the original work is properly cited.
View this paper at Google Scholar | DPI Digital Library
How to Cite this Paper
- IEEE Citation
- MLA Citation
- APA Citation
- BibTex Citation
- RIS Citation
IEEE Citation
IEEE Style Citation: Akshath M J, H S Sheshadri, “Denoising of skull stripped brain tumor MR images,” International Journal of Computer Sciences and Engineering, Vol.6, Issue.11, pp.323-329, 2018.
MLA Citation
MLA Style Citation: Akshath M J, H S Sheshadri "Denoising of skull stripped brain tumor MR images." International Journal of Computer Sciences and Engineering 6.11 (2018): 323-329.
APA Citation
APA Style Citation: Akshath M J, H S Sheshadri, (2018). Denoising of skull stripped brain tumor MR images. International Journal of Computer Sciences and Engineering, 6(11), 323-329.
BibTex Citation
BibTex Style Citation:
@article{J_2018,
author = {Akshath M J, H S Sheshadri},
title = {Denoising of skull stripped brain tumor MR images},
journal = {International Journal of Computer Sciences and Engineering},
issue_date = {11 2018},
volume = {6},
Issue = {11},
month = {11},
year = {2018},
issn = {2347-2693},
pages = {323-329},
url = {https://www.ijcseonline.org/full_paper_view.php?paper_id=3162},
doi = {https://doi.org/10.26438/ijcse/v6i11.323329}
publisher = {IJCSE, Indore, INDIA},
}
RIS Citation
RIS Style Citation:
TY - JOUR
DO = {https://doi.org/10.26438/ijcse/v6i11.323329}
UR - https://www.ijcseonline.org/full_paper_view.php?paper_id=3162
TI - Denoising of skull stripped brain tumor MR images
T2 - International Journal of Computer Sciences and Engineering
AU - Akshath M J, H S Sheshadri
PY - 2018
DA - 2018/11/30
PB - IJCSE, Indore, INDIA
SP - 323-329
IS - 11
VL - 6
SN - 2347-2693
ER -
![]() |
![]() |
![]() |
581 | 443 downloads | 307 downloads |
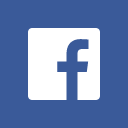
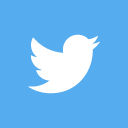
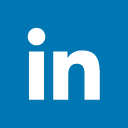
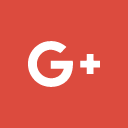
Abstract
To improve the accuracy in segmenting the brain tumor from Magnetic resonance images (MR), pre-processing of raw MR images plays significant importance, is required for proper visualization and detection of the tumor part, to increase the quality of the image affected by the noise. The paper is mainly focused on skull removal and denoising techniques. Inclusion of skull may lead to misclassification of tumour tissues and might increase the time complexity. In this study T1, T1contrast, T2, Flair MR images is analysed in axial, sagittal and coronal planes. Mathematical morphology operation with histogram thresholding is performed to remove the skull region. Denoising the images with various filters and evaluation of filters in terms of mean square error (MSE), signal to noise ratio (SNR) and peak signal to noise ratio (PSNR) are considered for the study. The Algorithm developed provides better results for skull stripping and high PSNR is obtained for wiener filter reducing the Gaussian noise by preserving the edges.
Key-Words / Index Term
Denoising, MR Image, Morphology, , MSE, PSNR, Skull removal
References
[1] P.Narendran, V. K. Narendra Kumar and K.Somasundaram, “3D Brain Tumors and Internal Brain Structures Segmentation in MR Images”, International Journal of Image, Graphics and Signal Processing, 2012,1, 35-43.
[2] S.cha, “Update on Brain tumor imaging: From anatomy to physiology, American journal of neuroradiology”, March 2006, 27(3), 475-487.
[3] Ayse Demirhan, Mustafa Toru, and Inan Guler, “Segmentation of Tumor and Edema along with healthy tiassues of brain using wavelets and Neural Networks”, IEEE Journal of biomedical and health informatics, vol. 19, no. 4, July 2015.
[4] Horst K. Hahn and Heinz-Otto Peitgen, “The Skull Stripping Problem in MRI Solved by a Single 3D Watershed Transform”, Proc. MICCAI, LNCS, 1935, 2000, 134-143, Springer.
[5] El Sayed A, El dashan, Heba M Mohsen, kenett revett, Abdel-Badeeh M salem, “Computer aided diagnosis of human brain tumor- A survey”, Elsevier-2014.
[6] F. Segonne, A.M. Dale, E. Busa, M. Glessner, D. Salat, H.K. Hahn, and B. Fischl, “ A hybrid approach to the skull stripping problem in MRI”, NeuroImage, 22, 2004, 1060– 1075.
[7] Andre G.R. Balan, AgmaJ.M.Traina, Marcela X. Ribeiro, Paulo M. A. Marques and Caetano Traina Jr., “Smart histogram analysis applied to the skull-stripping problem in T1-weighted MRI, Computers in Biology and Medicine”, 42, 2012, 509-522.
[8] Juan Eugenio Iglesias, Cheng-Yi Liu, Paul Thompson and Zhuowen Tu, “Robust Brain Extraction across Datasets and Comparison with Publicly Available Methods”, IEEE Transactions on Medical Imaging, 2011.
[9] Francisco J. Galdames, Fabrice Jaillet and Claudio A. Perez, “An Accurate Skull Stripping Method Based on Simplex Meshes and Histogram Analysis in Magnetic Resonance Images”, Elsevier, Journal of Neuroscience Methods, 206, 2012, 103–119.
[10] Audrey H. Zhuang, Daniel J. Valentino and Arthur W. Toga, “Skull-stripping Magnetic Resonance Brain Images Using a Model-based Level Set”, Neuro Image, 32, 2006, 79-92.
[11] Orazio Gambino, Enrico Daidone, Matteo Sciortino, Roberto Pirrone and Edoardo Ardizzone, “Automatic Skull Stripping in MRI based on Morphological Filters and Fuzzy C-means Segmentation”, 33rd Annual International Conference of the IEEE EMBS Boston, Massachusetts USA, 2011.
[12] Dwarikanath Mahapatra, “Skull Stripping of Neonatal Brain MRI: Using Prior Shape Information with Graph Cuts”, Springer, J Digit Imaging, 25, 2012, 802–814.
[13] Rosniza Roslan, Nursuriati Jamil and Rozi Mahmud, “Skull Stripping of MRI Brain Images using Mathematical Morphology”, 2010 IEEE EMBS Conference on Biomedical Engineering & Sciences (IECBES 2010), 2010.
[14] John Chivertona, Kevin Wells, Emma Lewis, Chao Chen, Barbara Podda and Declan Johnson, “Statistical Morphological Skull Stripping of Adult and Infant MRI Data”, Computers in Biology and Medicine, 37, 2007, 342-357.
[15] Selvaraj. D and Dhanasekaran. R, “MRI Brain Tumor Detection by Histogram and Segmentation by Modified GVF Model”, International Journal of Electronics and Communication Engineering & Technology (IJECET), 4(1), 2013, 55-68.
[16] Sajjad Mohsin, Sadaf Sajjad, Zeeshan Malik, and Abdul Hanan Abdullah, “Efficient Way of Skull Stripping in MRI to Detect Brain Tumor by Applying Morphological Operations, after Detection of False Background”, International Journal of Information and Education Technology, 2(4), 2012.
[17] Saritha Saladi, N Amrutha Prabha, “Analysis of denoising filters on MRI brain images”, volume 27, 2017.
[18] Anchal A, Budhiraja S, Goyal B, Dogra A, Agarwal S, “An efficient image denoising scheme for higher noise levels using spatial domain filters”, Biomedical and pharmacology journal,11(2), June 2018, 625-634.
[19] Iza Sazanita Isa, Siti Noraini Sulaiman, Muzaimi Mustapha, Sailudin Darus, “Evaluating Denoising performances of Fundamental filters for T2-Weighted MRI Images”, Elsevier, International conference on Knowledge based and intelligent information and engineering systems, 60(2015),760-768.
[20] Ahmed faisal, Sharmin parveen, Shahriar badsha, “An Improved image denoising and segmentation approach for detecting tumor from 2-D MRI Brain images”, Advanced computer science applictions and technologies, 2012.
[21] R. Balachander, R. Rajkumar, “Efficient and robust denoising of magnetic resonance brain images based on wavelet based nonlocal means algorithm”, International journal of engineering and science, 84-89, 2015.
[22] P. Perona, J. Malik, “Scale space and edge detection using anisotropic diffusion”, IEEE Transactions on Image Processing 12 (8) (1990) 629–639.
[23] Jian Yang, Jingfan Fan, Yongtian Wang, “Brain MR image denoising for rician noise using pre-smooth non-local means filter”, Biomedical Engineering online, 2015.
[24] Virendra Kumar, Sonia Saini, Sanjeev Dhiman, “Quality Improvement on MRI Corrupted with Rician Noise using Wave Atom Transform”, International Journal of Computer Applications, Vol. 37, No. 8, Jan. 2012.
[25] Pei-Eng Ng and Kai-Kuang Ma, “A Switching Median Filter with BDND for Extremely Corrupted Images”, IEEE Trans Image Processing, Vol. 15, No. 6, pp. 1506-1516, June 2006.
[26] A.C. Motagi and V.S. Malemath, “Detection of brain tumor using Expectation maximization and watershed”, International journal of scientific research in computer science and engineering, Vol. 6, Issue. 3, pp. 76-80, June 2018.
[27] S.D. Kasute, M Kohlekar, “ROI based Medical image compression”, International journal of scientific research in network security and communication, vol.5, Issue.1, pp-6-11, January 2011.