Face Recognition using Symbolic Data with Texture Features
Yogish Naik G.R.1 , Arun Kumar H.D.2 , Prabhakar C.J.3
Section:Research Paper, Product Type: Journal Paper
Volume-6 ,
Issue-11 , Page no. 354-358, Nov-2018
CrossRef-DOI: https://doi.org/10.26438/ijcse/v6i11.354358
Online published on Nov 30, 2018
Copyright © Yogish Naik G.R., Arun Kumar H.D., Prabhakar C.J. . This is an open access article distributed under the Creative Commons Attribution License, which permits unrestricted use, distribution, and reproduction in any medium, provided the original work is properly cited.
View this paper at Google Scholar | DPI Digital Library
How to Cite this Paper
- IEEE Citation
- MLA Citation
- APA Citation
- BibTex Citation
- RIS Citation
IEEE Style Citation: Yogish Naik G.R., Arun Kumar H.D., Prabhakar C.J., “Face Recognition using Symbolic Data with Texture Features,” International Journal of Computer Sciences and Engineering, Vol.6, Issue.11, pp.354-358, 2018.
MLA Style Citation: Yogish Naik G.R., Arun Kumar H.D., Prabhakar C.J. "Face Recognition using Symbolic Data with Texture Features." International Journal of Computer Sciences and Engineering 6.11 (2018): 354-358.
APA Style Citation: Yogish Naik G.R., Arun Kumar H.D., Prabhakar C.J., (2018). Face Recognition using Symbolic Data with Texture Features. International Journal of Computer Sciences and Engineering, 6(11), 354-358.
BibTex Style Citation:
@article{G.R._2018,
author = {Yogish Naik G.R., Arun Kumar H.D., Prabhakar C.J.},
title = {Face Recognition using Symbolic Data with Texture Features},
journal = {International Journal of Computer Sciences and Engineering},
issue_date = {11 2018},
volume = {6},
Issue = {11},
month = {11},
year = {2018},
issn = {2347-2693},
pages = {354-358},
url = {https://www.ijcseonline.org/full_paper_view.php?paper_id=3168},
doi = {https://doi.org/10.26438/ijcse/v6i11.354358}
publisher = {IJCSE, Indore, INDIA},
}
RIS Style Citation:
TY - JOUR
DO = {https://doi.org/10.26438/ijcse/v6i11.354358}
UR - https://www.ijcseonline.org/full_paper_view.php?paper_id=3168
TI - Face Recognition using Symbolic Data with Texture Features
T2 - International Journal of Computer Sciences and Engineering
AU - Yogish Naik G.R., Arun Kumar H.D., Prabhakar C.J.
PY - 2018
DA - 2018/11/30
PB - IJCSE, Indore, INDIA
SP - 354-358
IS - 11
VL - 6
SN - 2347-2693
ER -
![]() |
![]() |
![]() |
678 | 360 downloads | 305 downloads |
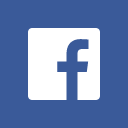
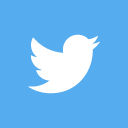
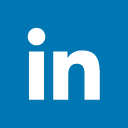
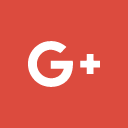
Abstract
Face recognition is a type of biometric identification method, it is challenging and one of the active research area in the field of Computer Vision. Variations in face image is due to changes in expression, presence of occlusion, geographical variations and illumination along with aging are some of the challenges to face recognition technique. In this paper, we attempt to solve illumination challenge for face recognition. Here, we propose a novel symbolic face recognition technique using Logarithm Gradient Histogram (LGH). Experimental results are carried out on standard benchmark databases like Extended YaleB, ORL. The performance of the proposed face recognition technique turns out to be 94.35 to 100% for the mentioned databases.
Key-Words / Index Term
Face recognition, Illumination invariant feature, Logarithm Gradient Histogram (LGH)
References
[1] Hiremath, P. S., and C. J. Prabhakar. "Face recognition technique using symbolic PCA method." International Conference on Pattern Recognition and Machine Intelligence. Springer, Berlin, Heidelberg, 2005.
[2] Hiremath, P. S., and C. J. Prabhakar. "Acquiring non linear subspace for face recognition using symbolic kernel PCA method." Journal of Symbolic Data Analysis 4.1 (2006): 15-26.
[3] Hiremath, P. S., and Manjunatha Hiremath. "Depth and intensity Gabor features based 3D face recognition using symbolic LDA and AdaBoost." International Journal of Image, Graphics and Signal Processing (IJIGSP) ISSN (2014): 2074-9074.
[4] Jolliffe, Ian. "Principal component analysis." International encyclopedia of statistical science. Springer, Berlin, Heidelberg, 2011. 1094-1096.
[5] Roweis, Sam T., and Lawrence K. Saul. "Nonlinear dimensionality reduction by locally linear embedding." science290.5500 (2000): 2323-2326.
[6] Chien, Jen-Tzung, and Chia-Chen Wu. "Linear Discriminant Analysis (LDA)." (2005).
[7] Delorme, Arnaud, and Scott Makeig. "EEGLAB: an open source toolbox for analysis of single-trial EEG dynamics including independent component analysis." Journal of neuroscience methods 134.1 (2004): 9-21.
[8] He, Xiaofei, and Partha Niyogi. "Locality preserving projections." Advances in neural information processing systems. 2004.
[9] Gais, Steffen, et al. "Sleep transforms the cerebral trace of declarative memories." Proceedings of the National Academy of Sciences 104.47 (2007): 18778-18783.
[10] Krogh, Anders, et al. "Predicting transmembrane protein topology with a hidden Markov model: application to complete genomes." Journal of molecular biology 305.3 (2001): 567-580.
[11] T. Ojala, M. Pietik¨ainen, D. Harwood, A comparative study of texture 420 measures with classification based on featured distributions, Pattern recognition 29 (1) (1996) 51–59.
[12] T. Ojala, M. Pietikainen, T. Maenpaa, Multiresolution gray-scale and rotation invariant texture classification with local binary patterns, IEEE Transactions on pattern analysis and machine intelligence 24 (7) (2002) 971–987.
[13] Z. Guo, L. Zhang, D. Zhang, A completed modeling of local binary pattern operator for texture classification, IEEE Transactions on Image Processing 19 (6) (2010) 1657–1663.
[14] T. Ahonen, E. Rahtu, V. Ojansivu, J. Heikkila, Recognition of blurred faces using local phase quantization, in: Pattern Recognition, 2008. ICPR 2008. 430 19th International Conference on, IEEE, 2008, pp. 1–4.
[15] S. Liao, X. Zhu, Z. Lei, L. Zhang, S. Li, Learning multi-scale block local binary patterns for face recognition, Advances in Biometrics (2007) 828– 837
[16] Zhu, Jun-Yong, et al. "Illumination invariant single face image recognition under heterogeneous lighting condition." Pattern Recognition 66 (2017): 313-327.