Application of Multilayer Perceptron for Forecasting of Selected IIPs of India – An Empirical Analysis
Dipankar Das1 , Awanish Kumar Tripathi2 , Ayushi Shah3 , Samarth Mehta4
Section:Research Paper, Product Type: Journal Paper
Volume-6 ,
Issue-11 , Page no. 400-406, Nov-2018
CrossRef-DOI: https://doi.org/10.26438/ijcse/v6i11.400406
Online published on Nov 30, 2018
Copyright © Dipankar Das, Awanish Kumar Tripathi, Ayushi Shah, Samarth Mehta . This is an open access article distributed under the Creative Commons Attribution License, which permits unrestricted use, distribution, and reproduction in any medium, provided the original work is properly cited.
View this paper at Google Scholar | DPI Digital Library
How to Cite this Paper
- IEEE Citation
- MLA Citation
- APA Citation
- BibTex Citation
- RIS Citation
IEEE Citation
IEEE Style Citation: Dipankar Das, Awanish Kumar Tripathi, Ayushi Shah, Samarth Mehta, “Application of Multilayer Perceptron for Forecasting of Selected IIPs of India – An Empirical Analysis,” International Journal of Computer Sciences and Engineering, Vol.6, Issue.11, pp.400-406, 2018.
MLA Citation
MLA Style Citation: Dipankar Das, Awanish Kumar Tripathi, Ayushi Shah, Samarth Mehta "Application of Multilayer Perceptron for Forecasting of Selected IIPs of India – An Empirical Analysis." International Journal of Computer Sciences and Engineering 6.11 (2018): 400-406.
APA Citation
APA Style Citation: Dipankar Das, Awanish Kumar Tripathi, Ayushi Shah, Samarth Mehta, (2018). Application of Multilayer Perceptron for Forecasting of Selected IIPs of India – An Empirical Analysis. International Journal of Computer Sciences and Engineering, 6(11), 400-406.
BibTex Citation
BibTex Style Citation:
@article{Das_2018,
author = {Dipankar Das, Awanish Kumar Tripathi, Ayushi Shah, Samarth Mehta},
title = {Application of Multilayer Perceptron for Forecasting of Selected IIPs of India – An Empirical Analysis},
journal = {International Journal of Computer Sciences and Engineering},
issue_date = {11 2018},
volume = {6},
Issue = {11},
month = {11},
year = {2018},
issn = {2347-2693},
pages = {400-406},
url = {https://www.ijcseonline.org/full_paper_view.php?paper_id=3177},
doi = {https://doi.org/10.26438/ijcse/v6i11.400406}
publisher = {IJCSE, Indore, INDIA},
}
RIS Citation
RIS Style Citation:
TY - JOUR
DO = {https://doi.org/10.26438/ijcse/v6i11.400406}
UR - https://www.ijcseonline.org/full_paper_view.php?paper_id=3177
TI - Application of Multilayer Perceptron for Forecasting of Selected IIPs of India – An Empirical Analysis
T2 - International Journal of Computer Sciences and Engineering
AU - Dipankar Das, Awanish Kumar Tripathi, Ayushi Shah, Samarth Mehta
PY - 2018
DA - 2018/11/30
PB - IJCSE, Indore, INDIA
SP - 400-406
IS - 11
VL - 6
SN - 2347-2693
ER -
![]() |
![]() |
![]() |
735 | 543 downloads | 265 downloads |
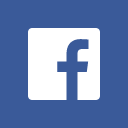
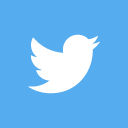
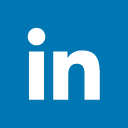
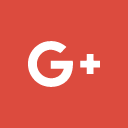
Abstract
The Index of Industrial Production (IIP) is an important indicator and a univariate time series data in nature. In the present study, the authors endeavored to develop forecasting models for twenty three (23) selected IIPs of India. The models were developed using Multilayer Perceptron. The study focused at (i) development of forecasting models, (ii) visualization of them, and (iii) analyzing the accuracies of the developed models. The study showed a mixed result with approximately twenty two percent (22%) i.e. five (5) out of twenty three (23) of the IIPs under study gave very good forecasting accuracy in terms of Mean Absolute Percentage Error (MAPE less than five), approximately twenty six percent (26%) i.e. six (6) out of twenty three (23) of the IIPs under study gave good forecasting accuracy (MAPE greater than or equal to five and MAPE less than ten) and approximately thirteen percent (13%) i.e. three (3) out of twenty three (23) of the IIPs under study gave moderate forecasting accuracy (MAPE greater than or equal to ten & MAPE less than twelve).
Key-Words / Index Term
Multilayer Perceptron, Index of Industrial Production, Mean Absolute Percentage Error, Forecasting, Time Series
References
[1] Awodele, O., & Jegede, O. (2009). Neural networks and its application in engineering. Science & IT.
[2] Sharma, V., Rai, S., & Dev, A. (2012). A comprehensive study of artificial neural networks. International Journal of Advanced research in computer science and software engineering, 2(10).
[3] N. Saranya, B.S.E. Zoraida, "Predicting Energy Consumption of a House using Neural Network", International Journal of Computer Sciences and Engineering, Vol.6, Issue.7, pp.275-277, 2018.
[4] K. Mohankumar, K. Sangeetha, "A Study on Earthquake Prediction Using Neural Network Algorithms", International Journal of Computer Sciences and Engineering, Vol.6, Issue.10, pp.200-204, 2018.
[5] Oancea, B., & Ciucu, Ş. C. (2014). Time series forecasting using neural networks. arXiv preprint arXiv:1401.1333.
[6] Crone, S. F. (2003). Artificial neural networks for time series prediction-A novel approach to inventory management using asymmetric cost functions. In IC-AI (pp. 193-199).
[7] Collantes-duarte, J., & Rivas-echeverría, F. (2002). Time Series Forecasting using ARIMA, Neural Networks and Neo Fuzzy Neurons. In 3rd WSEAS International Conference on Neural Networks and Applications (NNA`02), Fuzzy Sets and Fuzzy Systems (FSFS`02), Evolutionary Computation (EC`02), Interlaken, Switzerland February (pp. 11-14).
[8] Björklund, S., & Uhlin, T. (2017). Artificial neural networks for financial time series prediction and portfolio optimization (Doctoral dissertation, MA thesis. Linköping University).
[9] Claveria, O., & Torra, S. (2014). Forecasting tourism demand to Catalonia: Neural networks vs. time series models. Economic Modelling, 36, 220-228.
[10] Teixeira, J. P., & Fernandes, P. O. (2014). Tourism time series forecast with artificial neural networks. Tékhne, 12(1-2), 26-36.
[11] Yu, Lean & HUANG, WEI & Lai, Kin Keung & NAKAMORI, YOSHITERU & Wang, Shouyang. (2007). Neural Networks in Finance and Economics Forecasting. International Journal of Information Technology & Decision Making (IJITDM). 06. 113-140. 10.1142/S021962200700237X.
[12] Moody, J. (1995). Economic forecasting: Challenges and neural network solutions.
[13] Gonzalez, S. (2000). Neural networks for macroeconomic forecasting: a complementary approach to linear regression models (pp. 2000-07). Canada: Department of Finance.
[14] Tkacz, G., & Hu, S. (1999). Forecasting GDP growth using artificial neural networks (pp. 99-3). Ottawa: Bank of Canada.
[15] Nakamura, E. (2005). Inflation forecasting using a neural network. Economics Letters, 86(3), 373-378.
[16] Moody, J., Levin, U., & Rehfuss, S. (1993). Predicting the US index of industrial production.
[17] Heravi, S., Osborn, D. R., & Birchenhall, C. R. (2004). Linear versus neural network forecasts for European industrial production series. International Journal of Forecasting, 20(3), 435-446.
[18] Singh, S. S., Devi, L. T., & Deb Roy, T. (2016). Time series analysis of index of industrial production of India. IOSR Journal of Mathematics, 12(3), 1-7.
[19] Potdar, K., & Kinnerkar, R. (2017, July). A non-linear autoregressive neural network model for forecasting Indian index of industrial production. In IEEE Region 10 Symposium (TENSYMP), 2017 (pp. 1-5). IEEE.
[20] The data has been published by Ministry of Statistics and Programme Implementation and sourced from Open Government Data (OGD) Platform of India [https://data.gov.in/resources/monthly-indices-all-india-index-industrial-production-nic-2008-2-digit-and-sectoral-leve-0]. Released under National Data Sharing and Accessibility Policy (NDSAP): https://data.gov.in/sites/default/files/NDSAP.pdf
[21] Nikolaos Kourentzes (2017). nnfor: Time Series Forecasting with Neural Networks. R package version 0.9.2. https://CRAN.R-project.org/package=nnfor