Revelation of Down Syndrome Using Artificial Neural Network
Vincy Devi V. K1 , ajesh R2
Section:Research Paper, Product Type: Journal Paper
Volume-6 ,
Issue-11 , Page no. 526-530, Nov-2018
CrossRef-DOI: https://doi.org/10.26438/ijcse/v6i11.526530
Online published on Nov 30, 2018
Copyright © Vincy Devi V. K, Rajesh R . This is an open access article distributed under the Creative Commons Attribution License, which permits unrestricted use, distribution, and reproduction in any medium, provided the original work is properly cited.
View this paper at Google Scholar | DPI Digital Library
How to Cite this Paper
- IEEE Citation
- MLA Citation
- APA Citation
- BibTex Citation
- RIS Citation
IEEE Citation
IEEE Style Citation: Vincy Devi V. K, Rajesh R, “Revelation of Down Syndrome Using Artificial Neural Network,” International Journal of Computer Sciences and Engineering, Vol.6, Issue.11, pp.526-530, 2018.
MLA Citation
MLA Style Citation: Vincy Devi V. K, Rajesh R "Revelation of Down Syndrome Using Artificial Neural Network." International Journal of Computer Sciences and Engineering 6.11 (2018): 526-530.
APA Citation
APA Style Citation: Vincy Devi V. K, Rajesh R, (2018). Revelation of Down Syndrome Using Artificial Neural Network. International Journal of Computer Sciences and Engineering, 6(11), 526-530.
BibTex Citation
BibTex Style Citation:
@article{K_2018,
author = {Vincy Devi V. K, Rajesh R},
title = {Revelation of Down Syndrome Using Artificial Neural Network},
journal = {International Journal of Computer Sciences and Engineering},
issue_date = {11 2018},
volume = {6},
Issue = {11},
month = {11},
year = {2018},
issn = {2347-2693},
pages = {526-530},
url = {https://www.ijcseonline.org/full_paper_view.php?paper_id=3200},
doi = {https://doi.org/10.26438/ijcse/v6i11.526530}
publisher = {IJCSE, Indore, INDIA},
}
RIS Citation
RIS Style Citation:
TY - JOUR
DO = {https://doi.org/10.26438/ijcse/v6i11.526530}
UR - https://www.ijcseonline.org/full_paper_view.php?paper_id=3200
TI - Revelation of Down Syndrome Using Artificial Neural Network
T2 - International Journal of Computer Sciences and Engineering
AU - Vincy Devi V. K, Rajesh R
PY - 2018
DA - 2018/11/30
PB - IJCSE, Indore, INDIA
SP - 526-530
IS - 11
VL - 6
SN - 2347-2693
ER -
![]() |
![]() |
![]() |
661 | 553 downloads | 254 downloads |
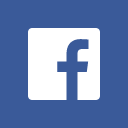
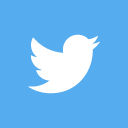
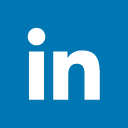
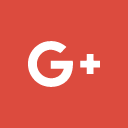
Abstract
A disorder in genetic chromosome 21 is popularly known as the Down syndrome (trisomy 21). It results in development and intellectual delays, but if we are able to give the exact care early on, it can make a good difference. Studies show that we can detect the Down syndrome in the early stages of pregnancy by identifying the absence of the fetal nasal bone. The common method followed in this issue is the visual identification of the ‘absence’ using ultra sonogram image of the nasal bone region. However, the visual identification technique is inefficient and difficult to follow. Thus, image processing based visual extraction technique can play a role in this case. In this paper, we have the raw data, which is employed to train the Back Propagation Neural Network (BPNN). The ultrasonogram images can be analyzed using this feed forward trained neural network and the detection can be made with appreciably low error rates. MATLAB is the platform used in this work for training the Artificial Neural Network (ANN).
Key-Words / Index Term
Down syndrome, Back Propagation Neural Network, Feature extraction, chromosomal abnormalities
References
[1] ACOG Committee, “Screening for fetal chromosomal abnormalities”, Obstest Gynecol , No. 77, pp. 217-228, 2007.
[2] Jiri D.SonekMD et.al., “Prenatal ultrasonographic diagnosis of nasal bone abnormalities in three fetuses with Down syndrome”, American Journal of Obstetrics and Gynecology, Vol. 186, Issue 1, pp. 139-141, 2002.
[3] S. Cicero et.al., “Absence of nasal bone in fetuses with trisomy 21 at 11 – 14 weeks of gestation: an observational study”, Lancet , No. 358, pp. 1665–1667, 2001.
[4] Orlandi et.al., “Measurement of nasal bone length at 11–14 weeks of pregnancy and its potential role in Down syndrome risk assessment”, Ultrasound Obstet Gynecol, Vol. 22, pp. 36-39, 2003.
[5] J. Sonek and K.H Nicolaides, “Prenatal ultrasonographic diagnosis of nasal bone abnormalities in three fetuses with Down syndrome”, Am. J. Obstet Gynecol, Vol. 186, pp. 139–141, 2002.
[6] B. Bromley et.al., “Fetal nose bone length: a marker for Down syndrome in the second trimester”, J. Ultrasound Med, Vol. 21,: pp. 1387 –1394, 2002.
[7] S. Cicero et.al., “Nasal bone hypoplasia in trisomy 21 at 15 – 22 weeks gestation”, Ultrasound Obstet Gynecol, Vol. 21, pp. 15–18, 2003.
[8] J.D. Sonek. “Nasal bone assessment in prenatal screening for trisomy 21”, Am. J. Obstet Gynecol, Vol. 195, pp. 1219–1230, 2006.
[9] M.S Beksac et.al., “Assessment of antepartum fetal heart rate tracings using neural networks”, In: Van Geijn HP, Copra, FJA, eds, A Critical Appraisal of Fetal Surveillance. Amsterdam: Elsevier, pp. 354-362, 1994.
[10] M.S. Beksac et.al., “Development and application of a simple expert system for the interpretation of the antepartum fetal heart rate tracings”, Eur. J. Obstet Gynecol Reprod Biol, No. 37: pp. 133-141, 1990.
[11] D.R. Hush and B.G. Home, “Progress in supervised neural networks”, IEEE Signal Processing Magazine, pp. 8-39, 1993..
[12] N.J Wald et al., “Maternal serum screening for Down`s syndrome in early pregnancy”, BMJ, Vol. 297, pp. 883-887, 1988.
[13] Coppedè et.al., “Polymorphisms in folatemetabolizing genes, chromosome damage, and risk of Down syndrome in Italian women: identification of key factors using artificial neural networks”, BMC Medical Genomics, Vol. 42, No. 3, 2010.
[14] S. Penco, et.al., “Assessment of the Role of Genetic Polymorphism in Venous Thrombosis Through Artificial Neural Networks”, Annals of Human Genetics, Vol. 69, No. 693-706, 2005.
[15] P.J.C Lisboa, “A review of evidence of health benefit from artificial neural networks in medical intervention”, Neural Networks, Vol. 15, pp. 11-39, 2002.
[16] E. Grossi et.al., “International experience on the use of artificial neural networks in gastroenterology”, Dig Liver Dis, Vol. 39, pp. 278-85, 2007.
[17] M. Tabaton et.al., “Artificial Neural Networks Identify the Predictive Values of Risk Factors on the Conversion of Amnestic Mild Cognitive Impairment”, J Alzheimers Dis., Vol. 19, pp. 1035-1040, 2010.
[18] M.E Street et.al., “Placental determinants of fetal growth: identification of key factors in the insulinlike growth factor and cytokine systems using artificial neural networks”, BMC Pediatr, Vol. 8, No.24, 2008.
[19] E. Grossi, “Neuropathological findings processed by artificial neural networks (ANNs) can perfectly distinguish Alzheimer’s patients from controls in the Nun Study”, BMC Neurol, Vol. 7, No. 15, 2007.
[20] Mosbesh R.Kaloop and Jong Wan Hu, “Optimizing the De-Noise Neural Network Model for GPS Time Series Monitoring of Structures”, Sensors, Vol. 15, No. 9, pp. 24428-24444, 2015.
[21] Kayhan Gulez et.al., “Power Op-Amp Based Active Filter Design with Self Adjustable Gain Control by Neural Networks for EMI Noise Problem”. In: D.S Huang, X.P Zhang, G.B Huang (eds), Advances in Intelligent Computing. ICIC 2005. Lecture Notes in Computer Science, Vol. 3644. Springer, Berlin, Heidelberg Huang (Eds.): ICIC 2005, Part I, LNCS 3644, pp. 243 – 252, 2005.
[22] P Kaur et.al., “Accurate Prediction of Fetal Images for measuring growth of Fetus using Genetic Algorithm and Back -Propagation Technique of Neural Network”, Int. J. Sci. Res. in Computer Science and Engineering, Vol.5, Issue 3, pp. 30-41, 2017.
[23] V. Davis and S. Devane, “Diagnosis of Brain Hemorrhage Using Artificial Neural Network”, Int. J. Sci. Res. in Network Security and Communication, Vol. 5, Issue 1, pp. 20-23, 2017.
[24] Vijo M Joy and S. Krishnakumar, “ANN Based Load Scheduling Method for Power Management Systems”, IJEECS, Vol. 6, Issue 10, pp. 310-313, 2017.
[25] Vijo M Joy and S. Krishnakumar, “Optimal Design of Power Sheduling using Artificial Neural Network in An Isolated Power System”, International Journal of Pure and Applied Mathematics, Volume 118 No. 8, pp. 289-294, 2018.
[26] Vijo M Joy and S Krishnakumar, “Efficient Load Scheduling Method For Power Management,” IJSTR, vol.5, issue 1, January 2016.
[27] Divyansh Mathur, “Maximum Power Point Tracking with Artificial Neural Network”. International Journal of Emerging Science and Engineering, Vol. 2, No.3, 2014.