Privacy Preserving Big Data Usings Combine Anonymous And Encryption Approach-Survey
Vidhi Desai1
Section:Survey Paper, Product Type: Journal Paper
Volume-6 ,
Issue-11 , Page no. 676-680, Nov-2018
CrossRef-DOI: https://doi.org/10.26438/ijcse/v6i11.676680
Online published on Nov 30, 2018
Copyright © Vidhi Desai . This is an open access article distributed under the Creative Commons Attribution License, which permits unrestricted use, distribution, and reproduction in any medium, provided the original work is properly cited.
View this paper at Google Scholar | DPI Digital Library
How to Cite this Paper
- IEEE Citation
- MLA Citation
- APA Citation
- BibTex Citation
- RIS Citation
IEEE Citation
IEEE Style Citation: Vidhi Desai, “Privacy Preserving Big Data Usings Combine Anonymous And Encryption Approach-Survey,” International Journal of Computer Sciences and Engineering, Vol.6, Issue.11, pp.676-680, 2018.
MLA Citation
MLA Style Citation: Vidhi Desai "Privacy Preserving Big Data Usings Combine Anonymous And Encryption Approach-Survey." International Journal of Computer Sciences and Engineering 6.11 (2018): 676-680.
APA Citation
APA Style Citation: Vidhi Desai, (2018). Privacy Preserving Big Data Usings Combine Anonymous And Encryption Approach-Survey. International Journal of Computer Sciences and Engineering, 6(11), 676-680.
BibTex Citation
BibTex Style Citation:
@article{Desai_2018,
author = {Vidhi Desai},
title = {Privacy Preserving Big Data Usings Combine Anonymous And Encryption Approach-Survey},
journal = {International Journal of Computer Sciences and Engineering},
issue_date = {11 2018},
volume = {6},
Issue = {11},
month = {11},
year = {2018},
issn = {2347-2693},
pages = {676-680},
url = {https://www.ijcseonline.org/full_paper_view.php?paper_id=3224},
doi = {https://doi.org/10.26438/ijcse/v6i11.676680}
publisher = {IJCSE, Indore, INDIA},
}
RIS Citation
RIS Style Citation:
TY - JOUR
DO = {https://doi.org/10.26438/ijcse/v6i11.676680}
UR - https://www.ijcseonline.org/full_paper_view.php?paper_id=3224
TI - Privacy Preserving Big Data Usings Combine Anonymous And Encryption Approach-Survey
T2 - International Journal of Computer Sciences and Engineering
AU - Vidhi Desai
PY - 2018
DA - 2018/11/30
PB - IJCSE, Indore, INDIA
SP - 676-680
IS - 11
VL - 6
SN - 2347-2693
ER -
![]() |
![]() |
![]() |
439 | 306 downloads | 314 downloads |
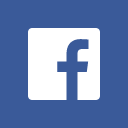
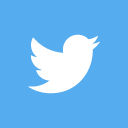
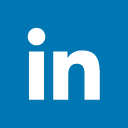
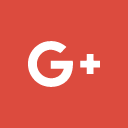
Abstract
In today’s world each individual wish that his private information is not revealed in some or the other way. Privacy preservation plays a vital role in preventing individual private data preserved from the praying eyes. Anonymization techniques enable publication of information which permit analysis and guarantee privacy of sensitive information in data against variety of attacks. The problem is that information loss and distortion are unavoidable by anonymization job. To reduce the distortion, this paper presents an efficient method that is based on deep anonymization detection. In the method, data publishers analyze the anonymization work, and determine if it is deep or light. If it is thought as deep anonymization, high information distortion is allowed when being distributed to a third party after anonymization. Otherwise, information distortion is kept as low as possible when anonymizing Big-Data to provide the receivers with more meaningful data. The decision for deep anonymization is done by considering a domain data characteristic, data receiver’s purpose, and data criticality. Anonymization approaches are used to develop to reduce information loss or increase privacy protection. It aimed to give comparative evolution of the various algorithms. These algorithms are compared for efficiency (in terms of time) and utility loss. We analysis that paillier encryption is more efficient than other algorithms
Key-Words / Index Term
Privacy, Anonymization, encryption, Big Data
References
[1] Latanya Sweeney. k-anonymity: a model for protecting privacy. International Journal on Uncertainty, Fuzziness and Knowledge-Based Systems, 10(5):557570, 2002
[2] Turban and J.E. Aronaon. Decision support Systems and Intelligent Systems, Prentice-Hall, New Jersey, USA, 2001
[3] P. P. de Wolf, J.M.Gouweleeuw, P. Kooiman, L. Wil-lenborg, Reflections on PRAM. Statistical data protection, proceedings of the conference, Lisbon, 1998.
[4] R. J. Bayardo and R. Agrawal. Data Privacy through Optimal k- Anonymization. In Proc. of ICDE-2005, 2005 [13] Stephen Lee Hansen and Sumitra Mukherjee. A Polynomial Algorithm for Optimal Microaggregation
[5] Matthias Schmid1 and Hans Schneeweiss, 2005, The Effect of Microaggregation Procedures on the Estimation of Linear Models: A Simulation Study
[6] M. Lindh and J. Nolin, “Information We Collect: Surveillance and Privacy in the Implementation of Google Apps for Education,” European Educational Research J., vol. 15, no. 6, 2016, pp. 644–663.
[7] A. Narayanan and V. Shmatikov, “Myths and Fallacies of `Personally Identifiable Information`,” Comm. ACM, vol. 53, no. 6, 2010, pp. 24–26.
[8] M. Barbaro, T. Zeller, and S. Hansell, “A Face Is Exposed for AOL Searcher no. 4417749,” The New York Times, vol. 9, no. 2008, 9 August 2006; www.nytimes.com/2006/08/09/technology/09aol.html
[9] M. Jensen, “Challenges of Privacy Protection in Big Data Analytics,” IEEE Int’l Congress on Big Data (Big Data Congress), 2013; doi.org/10.1109/BigData.Congress.2013.39.
[10] ISO/IEC 27040, Information Technology – Security Techniques – Storage, standard ISO/IEC 27040, Int’l Organization for Standardization, 2015; www.iso.org/standard/44404.html.
[11] 2016 Data Breach Investigations Report, report, Verizon, 2016; www.verizonenterprise.com/resources/reports/rp_DBIR_2016_Report_en_xg.pdf.
[12] Latanya Sweeney. k-anonymity: a model for protecting privacy. International Journal on Uncertainty, Fuzziness and Knowledge-Based Systems, 10(5):557570, 2002
[13] Turban and J.E. Aronaon. Decision support Systems and Intelligent Systems, Prentice-Hall, New Jersey, USA, 2001
[14] P. P. de Wolf, J.M.Gouweleeuw, P. Kooiman, L. Wil-lenborg, Reflections on PRAM. Statistical data protection, proceedings of the conference, Lisbon, 1998.
[15] R. J. Bayardo and R. Agrawal. Data Privacy through Optimal k- Anonymization. In Proc. of ICDE-2005, 2005 [13] Stephen Lee Hansen and Sumitra Mukherjee. A Polynomial Algorithm for Optimal Microaggregation
[16] Matthias Schmid1 and Hans Schneeweiss, 2005, The Effect of Microaggregation Procedures on the Estimation of Linear Models: A Simulation Study
[17] X. Zhang, C. Liu, S. Nepal, C. Yang, J. Chen, ”Privacy Preservation over Big Data in Cloud Systems,” Security, Privacy and Trust in Cloud Systems, pp 239-257, Springer.
[18] J. Sedayao, Enhancing cloud security using data anonymization, White Paper, Intel Coporation.
[19] Top Ten Big Data Security and Privacy Challenges, Technical report, Cloud Security Alliance, November 2012
[20] J. Salido, ”Differential privacy for everyone,” White Paper, Microsoft Coproration, 2012.
[21] Big Data Privacy Preservation, Ericsson Labs, http://labs.ericsson.com/blog/privacy-preservation-in-big-data-analytics.
[22] O. Heffetz and K. Ligett, Privacy and data-based research, NBER Working Paper, September 2013.
[23] M. V. Dijk, A. Juels, ”On the impossibility of cryptog-raphy alone for privacy-preserving cloud computing,” Proceed-ings of the 5th USENIX conference on Hot topics in security, August 10, 2010, pp.1-8.
[24] F. H. Cate, V. M. Schnberger, ”Notice and Consent in a World of Big Data,” Microsoft Global Privacy Summit Summary Report and Outcomes, Nov 2012.
[25] P. Samarati and L. Sweeney, ”Protecting privacy when disclosing information: k-anonymity and its enforcement through generalization and cell suppression,” Technical report, SRI International, 1998.
[26] Y. Lindell and B. Pinkas. Privacy preserving data mining. In CRYPTO, 2000.
[27] M. Naor and B. Pinkas. Computationally secure obliv-ious transfer. Journal of Cryptology, 2005