A Review On Exploring Online Ad Images Using A Clustering Approach
Krushil Bhadani1 , Bijal Talati2
Section:Review Paper, Product Type: Journal Paper
Volume-6 ,
Issue-11 , Page no. 695-698, Nov-2018
CrossRef-DOI: https://doi.org/10.26438/ijcse/v6i11.695698
Online published on Nov 30, 2018
Copyright © Krushil Bhadani, Bijal Talati . This is an open access article distributed under the Creative Commons Attribution License, which permits unrestricted use, distribution, and reproduction in any medium, provided the original work is properly cited.
View this paper at Google Scholar | DPI Digital Library
How to Cite this Paper
- IEEE Citation
- MLA Citation
- APA Citation
- BibTex Citation
- RIS Citation
IEEE Citation
IEEE Style Citation: Krushil Bhadani, Bijal Talati, “A Review On Exploring Online Ad Images Using A Clustering Approach,” International Journal of Computer Sciences and Engineering, Vol.6, Issue.11, pp.695-698, 2018.
MLA Citation
MLA Style Citation: Krushil Bhadani, Bijal Talati "A Review On Exploring Online Ad Images Using A Clustering Approach." International Journal of Computer Sciences and Engineering 6.11 (2018): 695-698.
APA Citation
APA Style Citation: Krushil Bhadani, Bijal Talati, (2018). A Review On Exploring Online Ad Images Using A Clustering Approach. International Journal of Computer Sciences and Engineering, 6(11), 695-698.
BibTex Citation
BibTex Style Citation:
@article{Bhadani_2018,
author = {Krushil Bhadani, Bijal Talati},
title = {A Review On Exploring Online Ad Images Using A Clustering Approach},
journal = {International Journal of Computer Sciences and Engineering},
issue_date = {11 2018},
volume = {6},
Issue = {11},
month = {11},
year = {2018},
issn = {2347-2693},
pages = {695-698},
url = {https://www.ijcseonline.org/full_paper_view.php?paper_id=3228},
doi = {https://doi.org/10.26438/ijcse/v6i11.695698}
publisher = {IJCSE, Indore, INDIA},
}
RIS Citation
RIS Style Citation:
TY - JOUR
DO = {https://doi.org/10.26438/ijcse/v6i11.695698}
UR - https://www.ijcseonline.org/full_paper_view.php?paper_id=3228
TI - A Review On Exploring Online Ad Images Using A Clustering Approach
T2 - International Journal of Computer Sciences and Engineering
AU - Krushil Bhadani, Bijal Talati
PY - 2018
DA - 2018/11/30
PB - IJCSE, Indore, INDIA
SP - 695-698
IS - 11
VL - 6
SN - 2347-2693
ER -
![]() |
![]() |
![]() |
460 | 394 downloads | 285 downloads |
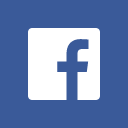
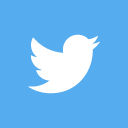
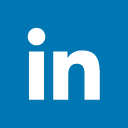
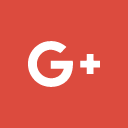
Abstract
Online advertising is a huge, rapidly growing advertising market in today`s world. One common form of online advertising is using image ads. A decision is made (often in real time) every time a user sees an ad, and the advertiser is eager to determine the best ad to display. Consequently, many algorithms have been developed that calculate the optimal ad to show to the current user at the present time. Typically, these algorithms focus on variations of the ad, optimizing among different properties such as background color, image size, or set of images but none of them define the property of objects. Our study looks at new qualities of ads that can be determined before an ad is shown (rather than online optimization) and defines which ad image’s objects are most likely to be successful. We present a set of algorithms that utilize machine learning to investigate online advertising and to construct object detection models which can foresee objects that are likely to be in successive ad image. The focus of results is to get high success rate in ad image with objects appear in it. In this paper we are finding the best classifier among the all.
Key-Words / Index Term
Object Detection, Machine Learning, And Prediction Model
References
[1] Michael Fire, Jonathan Schler. “Exploring Online Ad Images Using a Deep Convolutional Neural Network Approach” 2017.
[2] Jinsu Lee, Junseong Bang, and Seong Yang. “Object Detection With Sliding Window in Images Including Multiple Similar Objects”.
[3] Meera M K, Shajee Mohan B S. “bject Recognition in Images” 2016.
[4] Jie Sheng Tham, Yoong Choon Chang and Mohammad Faizal Ahmad Fauzi “Object Recognition using Depth Information of a Consumer Depth Camera” IEEE 2015.
[5] Kyung Mo Koo, Eui Young Cha. “Image recognition performance enhancements using image normalization” 2017.
[6] Hideaki Yanagisawa, Takuro Yamashita, Hiroshi Watanabe.” A Study on Object Detection Method from Manga Images using CNN” 2018.
[7] Csurka, G., C. R. Dance, L. Fan, J. Willamowski, and C. Bray. “Visual Categorization with Bags of Keypoints.” Workshop on Statistical Learning in Computer Vision. ECCV 1 (1–22), 1–2.
[8] Jie Sheng Tham, Yong-Shen Chen, Mohammad Faizal Ahmad Fauzi, Yoong Choon Chang. “Depth Image Object Recognition using Moment Invariants”2016.
[9] Astha Gautam, Anjana Kumari and Pankaj Singh “The Concept of Object Recognition” IJARCSSE 2015.
[10] Dr. R.Muralidharan ” Object Recognition Using K-Nearest Neighbor Supported By Eigen Value Generated From the Features of an Image” IJIRCCE 2014.
[11] Mingqiang Yang, Kidiyo Kpalma and Joseph Ronsin ” A Survey of Shape Feature Extraction Techniques” IETR-INSA 2008
[12] Divya Srivastava, Rajesh Wadhvani and Manasi Gyanchandani “Review: Color Feature Extraction Methods for Content Based Retrieval Image”
[13] Link” https://in.mathworks.com/help/vision/ref/vision.cascadeobjectdetector-system-object.html”.