Mutual city/state weather forecasting by ANN and HMM – Survey
Unnati Acharya1 , G.J.Sahani 2
Section:Survey Paper, Product Type: Journal Paper
Volume-6 ,
Issue-11 , Page no. 792-796, Nov-2018
CrossRef-DOI: https://doi.org/10.26438/ijcse/v6i11.792796
Online published on Nov 30, 2018
Copyright © Unnati Acharya, G.J.Sahani . This is an open access article distributed under the Creative Commons Attribution License, which permits unrestricted use, distribution, and reproduction in any medium, provided the original work is properly cited.
View this paper at Google Scholar | DPI Digital Library
How to Cite this Paper
- IEEE Citation
- MLA Citation
- APA Citation
- BibTex Citation
- RIS Citation
IEEE Citation
IEEE Style Citation: Unnati Acharya, G.J.Sahani, “Mutual city/state weather forecasting by ANN and HMM – Survey,” International Journal of Computer Sciences and Engineering, Vol.6, Issue.11, pp.792-796, 2018.
MLA Citation
MLA Style Citation: Unnati Acharya, G.J.Sahani "Mutual city/state weather forecasting by ANN and HMM – Survey." International Journal of Computer Sciences and Engineering 6.11 (2018): 792-796.
APA Citation
APA Style Citation: Unnati Acharya, G.J.Sahani, (2018). Mutual city/state weather forecasting by ANN and HMM – Survey. International Journal of Computer Sciences and Engineering, 6(11), 792-796.
BibTex Citation
BibTex Style Citation:
@article{Acharya_2018,
author = {Unnati Acharya, G.J.Sahani},
title = {Mutual city/state weather forecasting by ANN and HMM – Survey},
journal = {International Journal of Computer Sciences and Engineering},
issue_date = {11 2018},
volume = {6},
Issue = {11},
month = {11},
year = {2018},
issn = {2347-2693},
pages = {792-796},
url = {https://www.ijcseonline.org/full_paper_view.php?paper_id=3245},
doi = {https://doi.org/10.26438/ijcse/v6i11.792796}
publisher = {IJCSE, Indore, INDIA},
}
RIS Citation
RIS Style Citation:
TY - JOUR
DO = {https://doi.org/10.26438/ijcse/v6i11.792796}
UR - https://www.ijcseonline.org/full_paper_view.php?paper_id=3245
TI - Mutual city/state weather forecasting by ANN and HMM – Survey
T2 - International Journal of Computer Sciences and Engineering
AU - Unnati Acharya, G.J.Sahani
PY - 2018
DA - 2018/11/30
PB - IJCSE, Indore, INDIA
SP - 792-796
IS - 11
VL - 6
SN - 2347-2693
ER -
![]() |
![]() |
![]() |
521 | 382 downloads | 322 downloads |
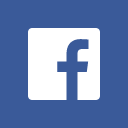
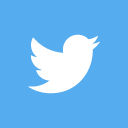
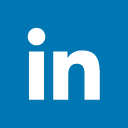
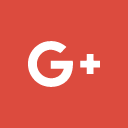
Abstract
Weather forecasting is an important apply for in meteorology and has been one of the most scientifically and technologically difficult problems around the world. Weather prediction approaches are challenged by difficult weather phenomena with incomplete explanation and past data. Weather phenomena have many parameters that are impossible to enumerate and measure. Increasing development on statement systems enabled weather forecast expert systems to combine and divide resources and thus hybrid system has emerged. Even though these improvements on weather forecast, these expert systems can’t be fully reliable since weather forecast is main problem. A predictive Neural Network model and Hidden Markov Model was also residential for the weather prediction program and the outcome compared with real weather data for the predicted periods. The results show that given enough case data, Data Mining techniques can be used for weather forecasting and climate change studies. Data mining is a process that uses a variety of data analysis tools to find out patterns and relationships in data that may be used to create applicable prediction. The proposed ANN and HMM evaluates the presentation of the developed models by applying unusual neurons, hidden layers and transfer functions to predict temperature for 365 days of the year. The criteria used for suitable model selection is mean square error (MSE).
Key-Words / Index Term
ANN, HMM, weather predication, regression, training, testing, Numerical Weather Forecasting etc
References
[1] Sivaramakrishnan T R and Meganathan S. (2011) Association Rule Mining and Classifier Approach for Quantitative Spot Rainfall Prediction. Journal of Theoretical and Applied Information Technology. 34(2), 173-177.
[2] S.Meganathan and T. R. Sivaramakrishnan, “Pattern Visualization on Meteorological Data for Rainfall Prediction Model”, Journal of Theoretical and Applied Information Technology, 35(2), 169-174, 2012.
[3] Sivaramakrishnan T R and Meganathan S. (2012) Data mining as a tool for precipitation prediction. Archives Des Sciences. 65(3), 8.
[4] Sivaramakrishnan T R and Meganathan S. (2012) Point rainfall prediction using data mining technique. Res. Journal of App. Sciences, Engineering and Technology. 4(13), 1899-1902.
[5] Sivaramakrishnan T R and Meganathan S. (2013) Association rule mining and classifier approach for 48 – hour rainfall prediction over Cuddalore station of east coast of India. Res. Journal of App. Sciences, Engineering and Technology. 5(14), 3692-3696.
[6] S.Meganathan, M. Poornima and A. Sumathi, “Proximity measures in clustering on patient diagnosis system”, International Journal of Applied Engineering Research, Vol.9(22), 12133-12139, 2014.
[7] S.Meganathan, T.R.Sivaramakrishnan, R.Balakrishnan and N.Rajeshkumar, “Rain Prediction using Environmental Data”, Rasayan Journal of Chemistry, 8(1), 56-58, 2015
[8] S.Meganathan, T.R.Sivaramakrishnan, M. Poornima and A.Sumathi, (2015) “Extraction of Winter Temperature Patterns for Agricultural Operations”, Research Journal of Pharmaceutical Biological and Chemical Sciences, 6(3), 1439-1442, 2015.
[9] Meganathan S, Sivaramakrishnan T R. (2015) A Technique for Spot Forecasting, MAUSAM, 66(1), 33-42.
[10] Kothawale DR, Revadekar JV, Rupa Kumar K, Recent trends in premonsoon daily temperature extremes over India. Journal of Earth System Science, 119(1), 51-65, 2010.
[11] Lahoz D, Miguel MS, Classification temp data with self organizing maps, Monografias del Seminario Matematico Garcia de Galdeano, 31,389-397, 2004.
[12] Sarah N Kohail, Alaa M El-Halees, Implementation of data mining techniques for meteorological data analysis. Int. J of Information Communication, Technology and Research, 1(3),96-100, 2011.
[13] Sarawagi S, Thomas S, Agrawal R. Integrating Association Rule Mining with Databases: Alternatives and Implications. Data Mining and Knowledge Discovery Journal, 4(2), 89-125, 2000.