A Survey based on Machine Learning Approaches for Detection of Human Behavioural Lie using physiological sensors and Face Recognition System
Bishan Lal Thakur1 , Divyansh Thakur2 , Payal Pandey3
Section:Survey Paper, Product Type: Journal Paper
Volume-6 ,
Issue-11 , Page no. 797-806, Nov-2018
CrossRef-DOI: https://doi.org/10.26438/ijcse/v6i11.797806
Online published on Nov 30, 2018
Copyright © Bishan Lal Thakur, Divyansh Thakur, Payal Pandey . This is an open access article distributed under the Creative Commons Attribution License, which permits unrestricted use, distribution, and reproduction in any medium, provided the original work is properly cited.
View this paper at Google Scholar | DPI Digital Library
How to Cite this Paper
- IEEE Citation
- MLA Citation
- APA Citation
- BibTex Citation
- RIS Citation
IEEE Style Citation: Bishan Lal Thakur, Divyansh Thakur, Payal Pandey, “A Survey based on Machine Learning Approaches for Detection of Human Behavioural Lie using physiological sensors and Face Recognition System,” International Journal of Computer Sciences and Engineering, Vol.6, Issue.11, pp.797-806, 2018.
MLA Style Citation: Bishan Lal Thakur, Divyansh Thakur, Payal Pandey "A Survey based on Machine Learning Approaches for Detection of Human Behavioural Lie using physiological sensors and Face Recognition System." International Journal of Computer Sciences and Engineering 6.11 (2018): 797-806.
APA Style Citation: Bishan Lal Thakur, Divyansh Thakur, Payal Pandey, (2018). A Survey based on Machine Learning Approaches for Detection of Human Behavioural Lie using physiological sensors and Face Recognition System. International Journal of Computer Sciences and Engineering, 6(11), 797-806.
BibTex Style Citation:
@article{Thakur_2018,
author = {Bishan Lal Thakur, Divyansh Thakur, Payal Pandey},
title = {A Survey based on Machine Learning Approaches for Detection of Human Behavioural Lie using physiological sensors and Face Recognition System},
journal = {International Journal of Computer Sciences and Engineering},
issue_date = {11 2018},
volume = {6},
Issue = {11},
month = {11},
year = {2018},
issn = {2347-2693},
pages = {797-806},
url = {https://www.ijcseonline.org/full_paper_view.php?paper_id=3246},
doi = {https://doi.org/10.26438/ijcse/v6i11.797806}
publisher = {IJCSE, Indore, INDIA},
}
RIS Style Citation:
TY - JOUR
DO = {https://doi.org/10.26438/ijcse/v6i11.797806}
UR - https://www.ijcseonline.org/full_paper_view.php?paper_id=3246
TI - A Survey based on Machine Learning Approaches for Detection of Human Behavioural Lie using physiological sensors and Face Recognition System
T2 - International Journal of Computer Sciences and Engineering
AU - Bishan Lal Thakur, Divyansh Thakur, Payal Pandey
PY - 2018
DA - 2018/11/30
PB - IJCSE, Indore, INDIA
SP - 797-806
IS - 11
VL - 6
SN - 2347-2693
ER -
![]() |
![]() |
![]() |
494 | 321 downloads | 272 downloads |
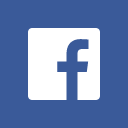
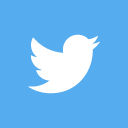
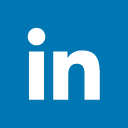
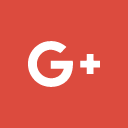
Abstract
At present there is a huge need of system which uses both physiological and facial data to detect human behavioral lie, thus this survey is based on getting insight for developing a machine learning based technique using facial and physiological data for detection of human behavior. The purpose of this survey is to identify various physiological sensors and their parameters along with sensing data, also to know whether physiological signals are robust and can be controlled by human being or not. It also reviews about various machine learning techniques for face recognition system and presented the most effective face recognition system in our survey. By getting significant understanding of physiological data and facial data with their classification rate it becomes possible to deduce a machine learning based algorithm using facial and physiological data for detection of human behavioral lie. This survey compiled the work done by various author to provide the precise information about the machine learning techniques, physiological sensors, face recognition system for human behavioral lie.
Key-Words / Index Term
Machine learning techniques, Physiological Sensors, Face Recognition, Emotion Recognition, Lie Detection
References
[1] El Naqa, I., & Murphy, M. J. (2015). What is machine learning?.In Machine Learning in Radiation Oncology (pp. 3-11).Springer, Cham.
[2] http://www.contrib.andrew.cmu.edu/~mndarwis/ML.html
[3] Mukhopadhyay, S. C. (2015). Wearable sensors for human activity monitoring: A review. IEEE sensors journal, 15(3), 1321-1330.
[4] Sharma, N., &Gedeon, T. (2012). Objective measures, sensors and computational techniques for stress recognition and classification: A survey. Computer methods and programs in biomedicine, 108(3), 1287-1301.
[5] Bakker, J., Pechenizkiy, M., &Sidorova, N. (2011, December). What`s your current stress level? Detection of stress patterns from GSR sensor data. In Data Mining Workshops (ICDMW), 2011 IEEE 11th International Conference on (pp. 573-580).IEEE.
[6] Jerritta, S., Murugappan, M., Nagarajan, R., & Wan, K. (2011, March). Physiological signals based human emotion recognition: a review. In Signal Processing and its Applications (CSPA), 2011 IEEE 7th International Colloquium on (pp. 410-415). IEEE.
[7] Poh, M. Z., Swenson, N. C., & Picard, R. W. (2010). A wearable sensor for unobtrusive, long-term assessment of electrodermal activity.IEEE transactions on Biomedical engineering, 57(5), 1243-1252.
[8] Liu, M., Fan, D., Zhang, X., & Gong, X. (2016, November). Human emotion recognition based on galvanic skin response signal feature selection and svm. In Smart City and Systems Engineering (ICSCSE), International Conference on (pp. 157-160).IEEE.
[9] Jabid, T., Kabir, M. H., &Chae, O. (2010, September). Facial expression recognition using local directional pattern (LDP).In Image Processing (ICIP), 2010 17th IEEE International Conference on (pp. 1605-1608).IEEE.
[10] Lu, C. Y., Min, H., Gui, J., Zhu, L., & Lei, Y. K. (2013). Face recognition via weighted sparse representation. Journal of Visual Communication and Image Representation, 24(2), 111-116.
[11] Zhao, X., Shi, X., & Zhang, S. (2015). Facial expression recognition via deep learning.IETE technical review, 32(5), 347-355.
[12] Cao, Z., Yin, Q., Tang, X., & Sun, J. (2010, June). Face recognition with learning-based descriptor. In Computer Vision and Pattern Recognition (CVPR), 2010 IEEE Conference on (pp. 2707-2714). IEEE.
[13] Mohammed, A. A., Minhas, R., Wu, Q. J., & Sid-Ahmed, M. A. (2011). Human face recognition based on multidimensional PCA and extreme learning machine. Pattern Recognition, 44(10-11), 2588-2597
[14] Wioleta, S. (2013, June). Using physiological signals for emotion recognition. In Human System Interaction (HSI), 2013 The 6th International Conference on (pp. 556-561). IEEE.
[15] Nourbakhsh, N., Wang, Y., Chen, F., &Calvo, R. A. (2012, November). Using galvanic skin response for cognitive load measurement in arithmetic and reading tasks. In Proceedings of the 24th Australian Computer-Human Interaction Conference (pp. 420-423).ACM.
[16] Wagner, J., Kim, J., & André, E. (2005, July). From physiological signals to emotions: Implementing and comparing selected methods for feature extraction and classification. In Multimedia and Expo, 2005.ICME 2005. IEEE International Conference on (pp. 940-943). IEEE.
[17] Koelstra, S., Muhl, C., Soleymani, M., Lee, J. S., Yazdani, A., Ebrahimi, T., ...&Patras, I. (2012). Deap: A database for emotion analysis; using physiological signals. IEEE Transactions on Affective Computing, 3(1), 18-31.
[18] Chaves-González, J. M., Vega-Rodríguez, M. A., Gómez-Pulido, J. A., & Sánchez-Pérez, J. M. (2010). Detecting skin in face recognition systems: A colour spaces study. Digital Signal Processing, 20(3), 806-823.
[19]Guo, G., Li, S. Z., & Chan, K. (2000). Face recognition by support vector machines. In Automatic Face and Gesture Recognition, 2000. Proceedings. Fourth IEEE International Conference on (pp. 196-201). IEEE.
[20]Ahonen, T., Hadid, A., &Pietikainen, M. (2006). Face description with local binary patterns: Application to face recognition. IEEE Transactions on Pattern Analysis & Machine Intelligence, (12), 2037-2041.
[21]He, X., Yan, S., Hu, Y., Niyogi, P., & Zhang, H. J. (2005). Face recognition using laplacianfaces. IEEE transactions on pattern analysis and machine intelligence, 27(3), 328-340
[22]Gao, Y., & Leung, M. K. (2002). Face recognition using line edge map. IEEE Transactions on Pattern Analysis & Machine Intelligence, (6), 764-779.