Challenges and Analysis of Big Data: A Review
Aparpreet Singh1 , Sandeep Sharma2
Section:Review Paper, Product Type: Journal Paper
Volume-6 ,
Issue-11 , Page no. 849-858, Nov-2018
CrossRef-DOI: https://doi.org/10.26438/ijcse/v6i11.849858
Online published on Nov 30, 2018
Copyright © Aparpreet Singh, Sandeep Sharma . This is an open access article distributed under the Creative Commons Attribution License, which permits unrestricted use, distribution, and reproduction in any medium, provided the original work is properly cited.
View this paper at Google Scholar | DPI Digital Library
How to Cite this Paper
- IEEE Citation
- MLA Citation
- APA Citation
- BibTex Citation
- RIS Citation
IEEE Citation
IEEE Style Citation: Aparpreet Singh, Sandeep Sharma, “Challenges and Analysis of Big Data: A Review,” International Journal of Computer Sciences and Engineering, Vol.6, Issue.11, pp.849-858, 2018.
MLA Citation
MLA Style Citation: Aparpreet Singh, Sandeep Sharma "Challenges and Analysis of Big Data: A Review." International Journal of Computer Sciences and Engineering 6.11 (2018): 849-858.
APA Citation
APA Style Citation: Aparpreet Singh, Sandeep Sharma, (2018). Challenges and Analysis of Big Data: A Review. International Journal of Computer Sciences and Engineering, 6(11), 849-858.
BibTex Citation
BibTex Style Citation:
@article{Singh_2018,
author = {Aparpreet Singh, Sandeep Sharma},
title = {Challenges and Analysis of Big Data: A Review},
journal = {International Journal of Computer Sciences and Engineering},
issue_date = {11 2018},
volume = {6},
Issue = {11},
month = {11},
year = {2018},
issn = {2347-2693},
pages = {849-858},
url = {https://www.ijcseonline.org/full_paper_view.php?paper_id=3256},
doi = {https://doi.org/10.26438/ijcse/v6i11.849858}
publisher = {IJCSE, Indore, INDIA},
}
RIS Citation
RIS Style Citation:
TY - JOUR
DO = {https://doi.org/10.26438/ijcse/v6i11.849858}
UR - https://www.ijcseonline.org/full_paper_view.php?paper_id=3256
TI - Challenges and Analysis of Big Data: A Review
T2 - International Journal of Computer Sciences and Engineering
AU - Aparpreet Singh, Sandeep Sharma
PY - 2018
DA - 2018/11/30
PB - IJCSE, Indore, INDIA
SP - 849-858
IS - 11
VL - 6
SN - 2347-2693
ER -
![]() |
![]() |
![]() |
620 | 371 downloads | 338 downloads |
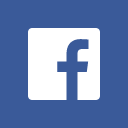
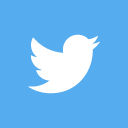
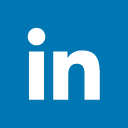
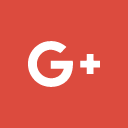
Abstract
Data in almost every field concerning daily needs is increasing by leaps and bounds. The problem of analysing such volume of data is enormous as tools and techniques may not be compatible of such volumes. In order to tackle the issue, data mining mechanisms are employed. Research mechanisms corresponding to big data analytics is discussed in this work. Also in case of misclassified data, it is required to tackle that data and then perform mining and classification. The mechanisms used to detect and predict anomalies along with misclassification are presented in comparative form. The objective of this work is to extract useful information regarding techniques used for big data analytics for future enhancements. Techniques used to minimise degree of misclassification in big data is analysed in comprehensive manner. These techniques extract useful patterns that could be used to observe big data in quick time.
Key-Words / Index Term
Big data, misclassified data, data mining
References
[1] C. Li, L. Zhu, and Z. Luo, “Big data mining based on time frequency for underdetermined BSS using density component analysis,” 2016 IEEE Int. Symp. Signal Process. Inf. Technol. ISSPIT 2016, pp. 188–192, 2017.
[2] K. Yang, X. Jia, and K. Ren, “Secure and Verifiable Policy Update Outsourcing for Big Data Access Control in the Cloud,” IEEE Trans. Parallel Distrib. Syst., vol. 26, no. 12, pp. 3461–3470, Dec. 2015.
[3] A. R. Rao and D. Clarke, “A fully integrated open-source toolkit for mining healthcare big-data: Architecture and applications,” Proc. - 2016 IEEE Int. Conf. Healthc. Informatics, ICHI 2016, pp. 255–261, 2016.
[4] D. V. Dimitrov, “Medical internet of things and big data in healthcare,” Healthc. Inform. Res., vol. 22, no. 3, pp. 156–163, 2016.
[5] B. Alami Milani and N. Jafari Navimipour, “A comprehensive review of the data replication techniques in the cloud environments: Major trends and future directions,” J. Netw. Comput. Appl., vol. 64, pp. 229–238, 2016.
[6] N. Al Nuaimi, “Data mining approaches used for predicting demand for healthcare services ,” 2014 10th Int. Conf. Innov. Inf. Technol. IIT 2014, pp. 42–47, 2014.
[7] J. Zhang, Y. Wang, C. Zhang, and Y. Shi, “Mining contiguous sequential generators in biological sequences,” IEEE/ACM Trans. Comput. Biol. Bioinforma., vol. 13, no. 5, pp. 855–867, 2016.
[8] H. Y. Chang, J. C. Lin, M. L. Cheng, and S. C. Huang, “A novel incremental data mining algorithm based on FP-growth for big data,” Proc. - 2016 Int. Conf. Netw. Netw. Appl. NaNA 2016, pp. 375–378, 2016.
[9] J. A. Rodger, “Informatics in Medicine Unlocked Discovery of medical Big Data analytics : Improving the prediction of traumatic brain injury survival rates by data mining Patient Informatics Processing Software Hybrid Hadoop Hive,” Informatics Med. Unlocked, vol. 1, no. 2015, pp. 17–26, 2016.
[10] J. O. Awoyemi, A. O. Adetunmbi, and S. A. Oluwadare, “Credit card fraud detection using machine learning techniques: A comparative analysis,” 2017 Int. Conf. Comput. Netw. Informatics, pp. 1–9, 2017.
[11] S. Udhaya and H. H. Inbarani, “Bijective Soft set based Classification of Medical Data,” IEEE Access, pp. 3–7, 2013.
[12] N. Khan, I. Yaqoob, I. A. T. Hashem, Z. Inayat, W. K. Mahmoud Ali, M. Alam, M. Shiraz, and A. Gani, “Big Data: Survey, Technologies, Opportunities, and Challenges,” Sci. World J., vol. 2014, pp. 1–18, 2014.
[13] Y.-J. Lee, M. Lee, M.-Y. Lee, S. J. Hur, and O. Min, “Design of a scalable data stream channel for big data processing,” 2015 17th Int. Conf. Adv. Commun. Technol., pp. 537–540, 2015.
[14] J. McHugh, P. E. Cuddihy, J. W. Williams, K. S. Aggour, V. S. Kumar, and V. Mulwad, “Integrated access to big data polystores through a knowledge-driven framework,” Proc. - 2017 IEEE Int. Conf. Big Data, Big Data 2017, vol. 2018-January, pp. 1494–1503, 2018.
[15] I. Taleb and M. A. Serhani, “Big Data Pre-Processing: Closing the Data Quality Enforcement Loop,” Proc. - 2017 IEEE 6th Int. Congr. Big Data, BigData Congr. 2017, no. 1, pp. 498–501, 2017.
[16] R. Barber, C. Garcia-arellano, R. Mueller, A. Storm, G. Lohman, C. Mohan, and H. Pirahesh, “Evolving Databases for New-Gen Big Data Applications,” Cidr, no. 3, 2017.
[17] S. Poledna, M. G. Miess, S. Schmelzer, E. Rovenskaya, S. Hochrainer-stigler, and S. Thurner, “Agent-based Modelling of Systemic Risk : A Big-data Approach Application : Systemic Risk Triggered by Natural Disasters Big-data,” Sebastian Poled. Michael Greg. Miess, Stefan Schmelzer, p. 10, 2015.
[18] T. Jung, X. Y. Li, W. Huang, J. Qian, L. Chen, J. Han, J. Hou, and C. Su, “AccountTrade: Accountable protocols for big data trading against dishonest consumers,” Proc. - IEEE INFOCOM, 2017.
[19] K. Mccormack and M. Smyth, “A Mathematical Solution to String Matching for Big Data Linking,” J. Stat. Sci. Appl., vol. 5, pp. 39–55, 2017.
[20] O. Alipourfard, H. H. Liu, J. Chen, S. Venkataraman, M. Yu, and M. Zhang, “CherryPick: Adaptively Unearthing the Best Cloud Configurations for Big Data Analytics,” 14th USENIX Symp. Networked Syst. Des. Implement. (NSDI 17), pp. 469–482, 2017.
[21] J. Wang, T. Baker, M. Balazinska, D. Halperin, B. Haynes, B. Howe, D. Hutchison, S. Jain, R. Maas, P. Mehta, B. Myers, J. Ortiz, D. Suciu, A. Whitaker, and S. Xu, “The Myria Big Data Management and Analytics System and Cloud Service,” Proc. CIDR, 2017.
[22] A. Malviya, A. Udhani, and S. Soni, “R-tool: Data analytic framework for big data,” 2016 Symp. Colossal Data Anal. Netw., pp. 1–5, 2016.
[23] A. Prakash and I. Limited, “Reconstructing Self Organizing Maps as Spider Graphs for better visual interpretation of large unstructured datasets.”
[24] D. P. and K. Ahmed, “A Survey on Big Data Analytics: Challenges, Open Research Issues and Tools,” Int. J. Adv. Comput. Sci. Appl., vol. 7, no. 2, 2016.
[25] S. Sarraf and R. Canada, “Big Data Application in Functional Magnetic Resonance Imaging using Apache Spark,” Springer, no. December, pp. 6–9, 2016.
[26] I. Olaronke, “Big Data in Healthcare : Prospects , Challenges and Resolutions,” IEEE Access, no. December, pp. 1152–1157, 2016.
[27] A. S. Panayides, C. S. Pattichis, and M. S. Pattichis, “The Promise of Big Data Technologies and Challenges for Image and Video Analytics in Healthcare,” IEEE, pp. 1278–1282, 2016.
[28] F. Permissions, “A Multidimension Taxonomy of Insider Threats in Cloud Computing,” IEEE Access, 2016.
[29] O. Marjanovic and B. Dinter, “Introduction to the HICSS-50 Organizational Issues of Business Intelligence , Business Analytics and Big Data Minitrack,” ACM Comput. Surv., p. 9981331, 2017.
[30] C. Science and M. Studies, “Securing user data on cloud using Fog computing and Decoy technique,” IEEE Access, vol. 7782, pp. 104–110, 2014.
[31] T. Scholl, R. Kuntschke, A. Reiser, and A. Kemper, “Community Training: Partitioning Schemes in Good Shape for Federated Data Grids,” in Third IEEE International Conference on e-Science and Grid Computing (e-Science 2007), 2007, pp. 195–203.
[32] Z. Yu, Q. Wang, Y. Fan, H. Dai, and M. Qiu, “An Improved Classifier Chain Algorithm for Multi-label Classification of Big Data Analysis,” pp. 1298–1301, 2015.