Review On Conventional and Advanced Classification Approaches in Remote Sensing Image Processing
Gandla Shivakanth1 , Prakash Singh Tanwar2
Section:Review Paper, Product Type: Journal Paper
Volume-6 ,
Issue-11 , Page no. 871-879, Nov-2018
CrossRef-DOI: https://doi.org/10.26438/ijcse/v6i11.871879
Online published on Nov 30, 2018
Copyright © Gandla Shivakanth, Prakash Singh Tanwar . This is an open access article distributed under the Creative Commons Attribution License, which permits unrestricted use, distribution, and reproduction in any medium, provided the original work is properly cited.
View this paper at Google Scholar | DPI Digital Library
How to Cite this Paper
- IEEE Citation
- MLA Citation
- APA Citation
- BibTex Citation
- RIS Citation
IEEE Style Citation: Gandla Shivakanth, Prakash Singh Tanwar, “Review On Conventional and Advanced Classification Approaches in Remote Sensing Image Processing,” International Journal of Computer Sciences and Engineering, Vol.6, Issue.11, pp.871-879, 2018.
MLA Style Citation: Gandla Shivakanth, Prakash Singh Tanwar "Review On Conventional and Advanced Classification Approaches in Remote Sensing Image Processing." International Journal of Computer Sciences and Engineering 6.11 (2018): 871-879.
APA Style Citation: Gandla Shivakanth, Prakash Singh Tanwar, (2018). Review On Conventional and Advanced Classification Approaches in Remote Sensing Image Processing. International Journal of Computer Sciences and Engineering, 6(11), 871-879.
BibTex Style Citation:
@article{Shivakanth_2018,
author = {Gandla Shivakanth, Prakash Singh Tanwar},
title = {Review On Conventional and Advanced Classification Approaches in Remote Sensing Image Processing},
journal = {International Journal of Computer Sciences and Engineering},
issue_date = {11 2018},
volume = {6},
Issue = {11},
month = {11},
year = {2018},
issn = {2347-2693},
pages = {871-879},
url = {https://www.ijcseonline.org/full_paper_view.php?paper_id=3260},
doi = {https://doi.org/10.26438/ijcse/v6i11.871879}
publisher = {IJCSE, Indore, INDIA},
}
RIS Style Citation:
TY - JOUR
DO = {https://doi.org/10.26438/ijcse/v6i11.871879}
UR - https://www.ijcseonline.org/full_paper_view.php?paper_id=3260
TI - Review On Conventional and Advanced Classification Approaches in Remote Sensing Image Processing
T2 - International Journal of Computer Sciences and Engineering
AU - Gandla Shivakanth, Prakash Singh Tanwar
PY - 2018
DA - 2018/11/30
PB - IJCSE, Indore, INDIA
SP - 871-879
IS - 11
VL - 6
SN - 2347-2693
ER -
![]() |
![]() |
![]() |
746 | 370 downloads | 329 downloads |
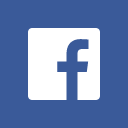
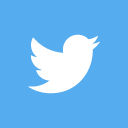
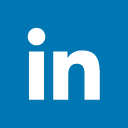
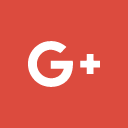
Abstract
Nowadays remote sensing image classification process has been most commonly used for object identification. It identifies the object in the remote sensing images by assigning the land cover classes to pixels. In this paper, a review on conventional and advanced remote sensing image classification techniques such as supervised, unsupervised, per pixel, sub pixel and object based image analysis processes has been provided. Further, a brief description about the effective features of different image classification algorithms like Fuzzy classifier, classification based on Artificial Neural Network (ANN), classification based on Support Vector Machine (SVM), Evolutionary Algorithms (EA) and Optimum Path Forest classification algorithms were also given. In the next section of paper various classification methodologies with their characteristics and examples of classifiers are explained. Moreover, this study compares the frequently used image classification algorithms and suggests the remote sensing image classifier to choose the best image classification technique based on the performance of classification that improves the accuracy range.
Key-Words / Index Term
Remote sensing, Image classification, ANN, SVM, Optimum Path Forest
References
[1] Y. Zhong, A. Ma, Y. soon Ong, Z. Zhu, L. Zhang, “Computational intelligence in optical remote sensing image processing”, Applied Soft Computing, Vol.64, pp.75-93, 2017.
[2] T. Lillesand, R.W. Kiefer, J. Chipman, “Remote Sensing and Image Interpretation”, 7th Edition, 2015.
[3] N. Alajlan, Y. Bazi, F. Melgani, R.R. Yager, “Fusion of supervised and unsupervised learning for improved classification of hyperspectral images”, Information Sciences, Vol.217, pp.39-55, 2012.
[4] G. Zhang, Z. Cao, Y. Gu, “A hybrid classifier based on rough set theory and support vector machines”, In International Conference on Fuzzy Systems and Knowledge Discovery, Springer, Berlin, Heidelberg, pp.1287-1296, 2005.
[5] D. Lu, Q. Weng, “A survey of image classification methods and techniques for improving classification performance”, International journal of Remote sensing, Vol.28 Issue.5, pp.823-870, 2007.
[6] M. Salah, “A survey of modern classification techniques in remote sensing for improved image classification”, Journal of Geomatics, Vol.11, Issue.1, pp.21, 2017.
[7] P. Kamavisdar, S. Saluja, S. Agrawal, “A survey on image classification approaches and techniques”, International Journal of Advanced Research in Computer and Communication Engineering, Vol.2, Issue.1, pp.1005-1009, 2013.
[8] S. Abburu, S.B. Golla, “Satellite image classification methods and techniques: A review”, International journal of computer applications, Vol.119, Issue.8, 2015.
[9] M.S. Prasad, T.S. Savithri, I.V.M. Krishna, “Techniques iIn Image Classification; A Survey”, Global Journal of Research In Engineering. Vol.15, Issue.6, 2015.
[10] M. Nair, J.S. Bindhu, “Supervised techniques and approaches for satellite image classification”, International Journal of Computer Applications, Vol.134, Issue.16, 2016.
[11] S.R. Hale, B.N. Rock, “Impact of topographic normalization on land-cover classification accuracy”, Photogrammetric Engineering & Remote Sensing, Vol.69, Issue.7, pp.785-791, 2003.
[12] D.G. Hadjimitsis, C.R.I. Clayton, V.S. Hope, “An assessment of the effectiveness of atmospheric correction algorithms through the remote sensing of some reservoirs”, International Journal of Remote Sensing, Vol.25, Issue.18, pp.3651-3674, 2004.
[13] E. Pretorius, “Image classification towards mapping of vegetation structure: a practical approach”, In International Cartographic Conference, Dresden, Germany, 2013.
[14] T. Kavzoglu, P.M. Mather, “The use of backpropagating artificial neural networks in land cover classification”, International journal of remote sensing, Vol.24, Issue.23, pp.4907-4938, 2003.
[15] J.T. Tou, R.C. Gonzalez, "Pattern recognition principles", Image Rochester NY, Vol.7, 1974.
[16] L. Černá, M. Chytrý, “Supervised classification of plant communities with artificial neural networks”, Journal of Vegetation Science, Vol.16, Issue.4, pp.407-414, 2005.
[17] S.D. Jawak, P. Devliyal, A.J. Luis, “A comprehensive review on pixel oriented and object oriented methods for information extraction from remotely sensed satellite images with a special emphasis on cryospheric applications”, Advances in Remote Sensing, Vol.4, Issue.03, p.177, 2015.
[18] S.K. Langley, H.M. Cheshire, K.S. Humes, “A comparison of single date and multitemporal satellite image classifications in a semi-arid grassland”, Journal of Arid Environments, Vol.49, Issue.2, pp.401-411, 2001.
[19] L. Castellana, A. D’Addabbo, G. Pasquariello, “A composed supervised/unsupervised approach to improve change detection from remote sensing”, Pattern Recognition Letters, Vol.28, Issue.4, pp.405-413, 2007.
[20] S. Lewinski, K. Zaremski, “Examples of object-oriented classification performed on high-resolution satellite images”, Miscellanea geographica, Vol.11, pp.349-358, 2004.
[21] P. Fisher, “The pixel: a snare and a delusion. International Journal of Remote Sensing”, Vol.18, Issue.3, pp.679-685, 1997.
[22] M. Xu, P. Watanachaturaporn, P.K. Varshney, M.K. Arora, “Decision tree regression for soft classification of remote sensing data”, Remote Sensing of Environment, Vol.97, Issue.3, pp.322-336, 2005.
[23] S.K. Singh, V. Kumar, S. Kanga, "Land Use/Land Cover Change Dynamics and River Water Quality Assessment Using Geospatial Technique: a case study of Harmu River, Ranchi (India)", International Journal of Scientific Research in Computer Science and Engineering, Vol.5, Issue.3, pp.17-24, 2017.
[24] J.J. Settle, S.A. Briggs, “Fast Maximum-likelihood Classification of Remotely Sensed Imagery”, International Journal of Remote Sensing, Vol.8, pp.723-734, 1987.
[25] R.S. Dwivedi, S. Kandrika, K.V. Ramana, “Comparison of classifiers of remote-sensing data for land-use/land-cover mapping”, Current Science, pp.328-335, 2004.
[26] P.J. Deer, P. Eklund, “A study of parameter values for a Mahalanobis distance fuzzy classifier”, Fuzzy Sets and Systems, Vol.137, Issue.2, pp.191-213, 2003.
[27] K. Kyrimis, “Monitoring land cover change detection with remote sensing methods in Magnesia prefecture in Greece”, Fresenius Environmental Bulletin, Vol.9, Issue.9/10, pp.659-666, 2000.
[28] H. Zhu, O. Basir, “An adaptive fuzzy evidential nearest neighbor formulation for classifying remote sensing images”, IEEE Transactions on Geoscience and Remote Sensing, Vol.43, Issue.8, pp.1874-1889, 2005.
[29] G. Mountrakis, J. Im, C. Ogole, “Support vector machines in remote sensing: A review”, ISPRS Journal of Photogrammetry and Remote Sensing, Vol.66, Issue.3, pp.247-259, 2011.
[30] H. Jiang, D. Zhao, Y. Cai, S. An, “A method for application of classification tree models to map aquatic vegetation using remotely sensed images from different sensors and dates”, Sensors, Vol.12, Issue.9, pp.12437-12454, 2012.
[31] P.O. Gislason, J.A. Benediktsson, J.R. Sveinsson, “Random Forests for Land Cover Classification”, Pattern Recognition Letters, Vol.27, pp.294-300, 2006.
[32] M. Marconcini, G. Camps-Valls, L. Bruzzone, “A composite semisupervised SVM for classification of hyperspectral images”, IEEE Geoscience and Remote Sensing Letters, Vol.6, Issue.2, pp.234-238, 2009.
[33] M.H. Tseng, S.J. Chen, G.H. Hwang, M.Y. Shen, “A genetic algorithm rule-based approach for land-cover classification”, ISPRS Journal of Photogrammetry and Remote Sensing, Vol.63, Issue.2, pp.202-212, 2008.
[34] P. Shanmugam, Y.H. Ahn, S. Sanjeevi, “A comparison of the classification of wetland characteristics by linear spectral mixture modelling and traditional hard classifiers on multispectral remotely sensed imagery in southern India”, ecological modelling, Vol.194, Issue.4, pp.379-394, 2006.
[35] C.E. Woodcock, S. Gopal, “Fuzzy set theory and thematic maps: accuracy assessment and area estimation”, International Journal of Geographical Information Science, Vol.14, Issue.2, pp.153-172, 2000.
[36] B. Mannan, A.K. Ray, “Crisp and fuzzy competitive learning networks for supervised classification of multispectral IRS scenes”, International Journal of Remote Sensing, Vol.24, Issue.17, pp.3491-3502, 2003.
[37] C.C. Yang, S.O. Prasher, P. Enright, C. Madramootoo, M. Burgess, P.K. Goel, I. Callum, “Application of decision tree technology for image classification using remote sensing data”, Agricultural Systems, Vol.76, Issue.3, pp.1101-1117, 2003.
[38] C. Wu, “Normalized spectral mixture analysis for monitoring urban composition using ETM+ imagery”, Remote Sensing of Environment, Vol.93, Issue.4, pp.480-492. 2004.
[39] J. Zhang, G.M. Foody, “A fuzzy classification of sub-urban land cover from remotely sensed imagery”, International journal of remote sensing, Vol.19, Issue.14, pp.2721-2738, 1998.
[40] N.S. Lele, "Image Classification Using Convolutional Neural Network", International Journal of Scientific Research in Computer Science and Engineering, Vol.6, Issue.3, pp.22-26, 2018.
[41] S.W. Myint, “Urban vegetation mapping using sub‐pixel analysis and expert system rules: a critical approach”, International Journal of Remote Sensing, Vol.27, Issue.13, pp.2645-2665, 2006.
[42] D.A. Roberts, M. Gardner, R. Church, S. Ustin, G. Scheer, R.O. Green, “Mapping chaparral in the Santa Monica Mountains using multiple endmember spectral mixture models”, Remote sensing of environment, Vol.65, Issue.3, pp.267-279, 1998.
[43] C. Wu, A.T. Murray, “Estimating impervious surface distribution by spectral mixture analysis”, Remote sensing of Environment, Vol.84, Issue.4, pp.493-505, 2003.
[44] J. Tang, L. Wang, S.W. Myint, “Improving urban classification through fuzzy supervised classification and spectral mixture analysis”, International Journal of Remote Sensing, Vol.28, Issue.18, pp.4047-4063, 2007.
[45] T. Blaschke, “Object based image analysis for remote sensing”, ISPRS journal of photogrammetry and remote sensing, Vol.65, Issue.1, pp.2-16, 2010.
[46] S.W. Myint, P. Gober, A. Brazel, S. Grossman-Clarke, Q. Weng, “Per-pixel vs. object-based classification of urban land cover extraction using high spatial resolution imagery”, Remote sensing of environment, Vol.115, Issue.5, pp.1145-1161, 2011.
[47] A.S. Banu, P. Vasuki, S.M.M. Roomi, A.Y. Khan, "SAR Image Classification by Wavelet Transform and Euclidean Distance with Shanon Index Measurement", International Journal of Scientific Research in Network Security and Communication, Vol.6, Issue.3, pp.13-17, 2018.
[48] N.R. Pal, D. Bhandari, “On object background classification”, International journal of systems science, Vol.23, Issue.11, pp.1903-1920, 1992.
[49] U.C. Benz, P. Hofmann, G. Willhauck, I. Lingenfelder, M. Heynen, “Multi-resolution, object-oriented fuzzy analysis of remote sensing data for GIS-ready information”, ISPRS Journal of photogrammetry and remote sensing, Vol.58, Issue.3-4, pp.239-258, 2004.
[50] Y. Zhong, L. Zhang, “An adaptive artificial immune network for supervised classification of multi-/hyperspectral remote sensing imagery”, IEEE Transactions on Geoscience and Remote Sensing, Vol.50, Issue.3, pp.894-909, 2012.
[51] L. Zhang, Y. Zhong, B. Huang, P. Li, “A resource limited artificial immune system algorithm for supervised classification of multi/hyper‐spectral remote sensing imagery”, International Journal of Remote Sensing, Vol.28, Issue.7, pp.1665-1686, 2007.
[52] B. Dixon, N. Candade, “Multispectral landuse classification using neural networks and support vector machines: one or the other, or both?”, International Journal of Remote Sensing, Vol.29, Issue.4, pp.1185-1206, 2008.
[53] C. Joshi, J. De Leeuw, A.K. Skidmore, I.C. Van Duren, H. Van Oosten, “Remotely sensed estimation of forest canopy density: A comparison of the performance of four methods”, International Journal of Applied Earth Observation and Geoinformation, Vol.8, Issue.2, pp.84-95, 2006.
[54] F. Ratle, G. Camps-Valls, J. Weston, “Semisupervised neural networks for efficient hyperspectral image classification”, IEEE Transactions on Geoscience and Remote Sensing, Vol.48, Issue.5, pp.2271-2282, 2010.
[55] L. Bruzzone, M. Chi, M. Marconcini, “A novel transductive SVM for semisupervised classification of remote-sensing images”, IEEE Transactions on Geoscience and Remote Sensing, Vol.44, Issue.11, pp.3363-3373, 2006.
[56] L. Gómez-Chova, G. Camps-Valls, J. Munoz-Mari, J. Calpe, “Semisupervised image classification with Laplacian support vector machines”, IEEE Geoscience and Remote Sensing Letters, Vol.5, Issue.3, pp.336-340, 2008.
[57] X. Yao, Y. Liu, “A new evolutionary system for evolving artificial neural networks”, IEEE transactions on neural networks, Vol.8, Issue.3, pp.694-713, 1997.
[58] M. Cruz-Ramírez, C. Hervás-Martínez, M. Jurado-Expósito, F. López-Granados, “A multi-objective neural network based method for cover crop identification from remote sensed data”, Expert Systems with Applications, Vol.39, Issue.11, pp.10038-10048, 2012.
[59] Y. Zhong, L. Zhang, P. Li, “Multispectral remote sensing image classification based on simulated annealing clonal selection algorithm”, in Preceedings IEEE International Geoscience and Remote Sensing Symposium (IGARSS `05), pp.3745-3748, 2005.
[60] B. Gong, J. Im, G. Mountrakis, “An artificial immune network approach to multi-sensor land use/land cover classification”, Remote Sensing of Environment, Vol.115, Issue.2, pp.600-614, 2011.
[61] C. Oscar, H. Francisco, H. Frank, “Genetic fuzzy systems: evolutionary tuning and learning of fuzzy knowledge bases”, World Scientific. Vol.19, 2001.
[62] D.G. Stavrakoudis, G.N. Galidaki, I.Z. Gitas, J.B. Theocharis, “A genetic fuzzy-rule-based classifier for land cover classification from hyperspectral imagery”, IEEE Transactions on Geoscience and Remote Sensing, Vol.50, Issue.1, pp.130-148, 2012.
[63] C. Puente, G. Olague, S.V. Smith, S.H. Bullock, A. Hinojosa-Corona, M.A. González-Botello, “A genetic programming approach to estimate vegetation cover in the context of soil erosion assessment”, Photogrammetric Engineering & Remote Sensing, Vol.77, Issue.4, pp.363-376, 2011.
[64] J. Keuchel, S. Naumann, M. Heiler, A. Siegmund, “Automatic land cover analysis for Tenerife by supervised classification using remotely sensed data”, Remote sensing of environment, Vol.86, Issue.4, pp.530-541, 2003.
[65] J.A. Gualtieri, R.F. Cromp, “Support vector machines for hyperspectral remote sensing classification”, In 27th AIPR Workshop: Advances in Computer-Assisted Recognition, International Society for Optics and Photonics, Vol.3584, pp.221-233, 1999.
[66] L. Hermes, D. Frieauff, J. Puzicha, J.M. Buhmann, “Support vector machines for land usage classification in Landsat TM imagery”, In Geoscience and Remote Sensing Symposium, 1999. IGARSS`99 Proceedings. IEEE 1999 International, Vol.1, pp.348-350, 1999.
[67] V. Vapnik, “Estimation of dependences based on empirical data [in Russian]”, Nauka, Moscow, 1979.
[68] G. Camps-Valls, L. Gómez-Chova, J. Calpe-Maravilla, J.D. Martín-Guerrero, E. Soria-Olivas, L. Alonso-Chordá, J. Moreno, “Robust support vector method for hyperspectral data classification and knowledge discovery”, IEEE Transactions on Geoscience and Remote sensing, Vol.42, Issue.7, pp.1530-1542, 2004.
[69] F. Melgani, L. Bruzzone, “Classification of hyperspectral remote sensing images with support vector machines”, IEEE Transactions on geoscience and remote sensing, Vol.42, Issue.8, pp.1778-1790, 2004.
[70] M. Fauvel, J. Chanussot, J.A. Benediktsson, “Evaluation of kernels for multiclass classification of hyperspectral remote sensing data”, In Acoustics, Speech and Signal Processing, ICASSP Proceedings, IEEE International Conference, Vol.2, pp.II-II, 2006.
[71] J.C. Bezdec, “Pattern Recognition with Fuzzy Objective Function Algorithms”, Plenum Press, New York, 1981.
[72] J.P. Papa, A.X. FalcãO, V.H.C. De Albuquerque, J.M.R. Tavares, “Efficient supervised optimum-path forest classification for large datasets”, Pattern Recognition, Vol. 45, Issue.1, pp.512-520, 2012.
[73] J.P. Papa, A.X. Falcao, C.T. Suzuki, “Supervised pattern classification based on optimum‐path forest”, International Journal of Imaging Systems and Technology, Vol.19, Issue.2, pp.120-131, 2009.
[74] A.X. Falcão, J. Stolfi, R. de Alencar Lotufo, “The image foresting transform: Theory, algorithms, and applications”, IEEE transactions on pattern analysis and machine intelligence, Vol.26, Issue.1, pp.19-29, 2004.
[75] A.A. Spadotto, J.C. Pereira, R.C. Guido, J.P. Papa, A.X. Falcao, A.R. Gatto, P.C. Cola, A.O. Schelp, “Oropharyngeal dysphagia identification using wavelets and optimum path forest”, In Communications, Control and Signal Processing, ISCCSP, 3rd International Symposium, pp.735-740, 2008.
[76] R. Pisani, P. Riedel, “Land use image classification through optimum-path forest clustering”, Geosci. Remote Sens. Symp. (IGARSS), pp.826-829, 2011.
[77] A. Samant, S. Kadge, "Classification of a Retinal Disease based on Different Supervised Learning Techniques", International Journal of Scientific Research in Network Security and Communication, Vol.5, Issue.3, pp.9-13, 2017.