Hybrid Distributed Intrusion Detection System
A.A. Ujeniya1 , R.D. Pawar2 , S.A. Sonawane3 , S.B. Shingade4 , S.R. Khonde5
Section:Research Paper, Product Type: Journal Paper
Volume-6 ,
Issue-12 , Page no. 232-237, Dec-2018
CrossRef-DOI: https://doi.org/10.26438/ijcse/v6i12.232237
Online published on Dec 31, 2018
Copyright © A.A. Ujeniya, R.D. Pawar, S.A. Sonawane, S.B. Shingade, S.R. Khonde . This is an open access article distributed under the Creative Commons Attribution License, which permits unrestricted use, distribution, and reproduction in any medium, provided the original work is properly cited.
View this paper at Google Scholar | DPI Digital Library
How to Cite this Paper
- IEEE Citation
- MLA Citation
- APA Citation
- BibTex Citation
- RIS Citation
IEEE Style Citation: A.A. Ujeniya, R.D. Pawar, S.A. Sonawane, S.B. Shingade, S.R. Khonde, “Hybrid Distributed Intrusion Detection System,” International Journal of Computer Sciences and Engineering, Vol.6, Issue.12, pp.232-237, 2018.
MLA Style Citation: A.A. Ujeniya, R.D. Pawar, S.A. Sonawane, S.B. Shingade, S.R. Khonde "Hybrid Distributed Intrusion Detection System." International Journal of Computer Sciences and Engineering 6.12 (2018): 232-237.
APA Style Citation: A.A. Ujeniya, R.D. Pawar, S.A. Sonawane, S.B. Shingade, S.R. Khonde, (2018). Hybrid Distributed Intrusion Detection System. International Journal of Computer Sciences and Engineering, 6(12), 232-237.
BibTex Style Citation:
@article{Ujeniya_2018,
author = {A.A. Ujeniya, R.D. Pawar, S.A. Sonawane, S.B. Shingade, S.R. Khonde},
title = {Hybrid Distributed Intrusion Detection System},
journal = {International Journal of Computer Sciences and Engineering},
issue_date = {12 2018},
volume = {6},
Issue = {12},
month = {12},
year = {2018},
issn = {2347-2693},
pages = {232-237},
url = {https://www.ijcseonline.org/full_paper_view.php?paper_id=3321},
doi = {https://doi.org/10.26438/ijcse/v6i12.232237}
publisher = {IJCSE, Indore, INDIA},
}
RIS Style Citation:
TY - JOUR
DO = {https://doi.org/10.26438/ijcse/v6i12.232237}
UR - https://www.ijcseonline.org/full_paper_view.php?paper_id=3321
TI - Hybrid Distributed Intrusion Detection System
T2 - International Journal of Computer Sciences and Engineering
AU - A.A. Ujeniya, R.D. Pawar, S.A. Sonawane, S.B. Shingade, S.R. Khonde
PY - 2018
DA - 2018/12/31
PB - IJCSE, Indore, INDIA
SP - 232-237
IS - 12
VL - 6
SN - 2347-2693
ER -
![]() |
![]() |
![]() |
413 | 275 downloads | 281 downloads |
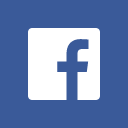
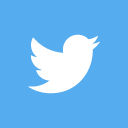
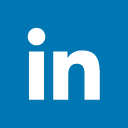
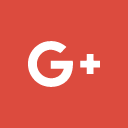
Abstract
There is rise in new Intrusion Detection Systems (IDSs) due to increasing frequency of various malicious activities over network and certain network policy violations. IDS, being an advanced tool and equipment to secure the network parameter by surveillance from the different network risks, is capable of detecting various attacks due to advancements in Computer Science. These advancements include machine learning models which can be integrated into an IDS for increasing the Detection Rate of attacks and minimizing the False Alarm Rate (false positives). In this paper, Hybrid Distributed IDS (HDIDS) is proposed in which strengths of Signature-based and Anomaly-based detection are combined together to detect different types of Denial of Service (DoS) attacks. HDIDS is presented by combining an anomaly-based detection algorithm and multiple signature-based detection algorithms. The signature-based multiple classifiers ensemble and can detect real time attack based on majority of votes from each classifier. Ensembled output use voting technique which are simplest to implement and produce favourable results. Anomaly based classifier has intensive focus over new and unknown attacks in distributed network. The dataset used for training the classifiers is ISCX CICIDS-17 consisting of latest attacks and 88 features providing better options for feature selection with respect to each classifier.
Key-Words / Index Term
— Machine Learning, Hybrid, Intrusion Detection System, Anomaly-based classifier, Signature-based classifier, Ensemble
References
[1] C. Guo, Y. Ping, N. Liu, S. Luo, “A two-level hybrid approach for intrusion detection”, Science Direct, Neurocomputing, Appl. 214, pp. 391–400, June 2016
[2] G. P. Spathoulas and S. K. Katsikas, “Reducing false positives in intrusion detection systems”, Science Direct, Computers and Security, Appl. 29, pp. 35-44, July 2009
[3] P. Casas, J. Mazel, P. Owezarski, “Unsupervised Network Intrusion Detection Systems: Detecting the Unknown without Knowledge”, Science Direct, Computer Communication, pp. 772-783, Jan 2012
[4] H. Sarvari, M M. Keikha, “Improving the Accuracy of Intrusion Detection Systems by Using the Combination of
Machine Learning Approaches”, IEEE, International Conference of Soft Computing and Pattern Recognition, pp. 334-337, June 2010
[5] A. Shenfield, D. Day, A. Ayesh, “Intelligent intrusion detection systems using artificial neural networks”, Science Direct, ICT Express 4, pp. 95-99, May 2018
[6] P. Aggarwala, S. Sharma, “Analysis of KDD Dataset Attributes - Class wise For Intrusion Detection”, Science Direct, Computer Science, Appl. 57, pp. 842-851, 2015
[7] R. Ashfaq, X. Wang, J. Z. Huang, H. Abbas, Y. He, “Fuzziness based semi-supervised learning approach for intrusion detection system”, Science Direct, Information Science, Appl. 378, pp. 484-497, May 2016
[8] S. Peddabachigaria, A. Abrahamb, C. Grosanc, J. Thomasa, “Modelling intrusion detection system using hybrid intelligent systems”, Science Direct, Journal of Network and Computer Applications, Appl. 30, pp. 114-132, June 2005
[9] S. Khonde, V. Ulagamuthalvi, “A Machine Learning Approach for Intrusion Detection using Ensemble Techniques - A survey”, International journal of scientific research in computer science, Engineering and Information Technology, Vol 3. Issue 1, ISSN - 2456-3307, pp. 328 – 338, 2018
[10] P. Rutravigneshwaran, “A Study of Intrusion Detection System using Efficient Data Mining Techniques”, International Journal of Scientific Research in Network Security and Communication, Volume-5, Issue-6, December 2017