Breast Cancer Segmentation Using Global Thresholding and Region Merging
Nidhi Singh1 , S. Veenadhari2
Section:Research Paper, Product Type: Journal Paper
Volume-6 ,
Issue-12 , Page no. 292-297, Dec-2018
CrossRef-DOI: https://doi.org/10.26438/ijcse/v6i12.292297
Online published on Dec 31, 2018
Copyright © Nidhi Singh, S. Veenadhari . This is an open access article distributed under the Creative Commons Attribution License, which permits unrestricted use, distribution, and reproduction in any medium, provided the original work is properly cited.
View this paper at Google Scholar | DPI Digital Library
How to Cite this Paper
- IEEE Citation
- MLA Citation
- APA Citation
- BibTex Citation
- RIS Citation
IEEE Style Citation: Nidhi Singh, S. Veenadhari, “Breast Cancer Segmentation Using Global Thresholding and Region Merging,” International Journal of Computer Sciences and Engineering, Vol.6, Issue.12, pp.292-297, 2018.
MLA Style Citation: Nidhi Singh, S. Veenadhari "Breast Cancer Segmentation Using Global Thresholding and Region Merging." International Journal of Computer Sciences and Engineering 6.12 (2018): 292-297.
APA Style Citation: Nidhi Singh, S. Veenadhari, (2018). Breast Cancer Segmentation Using Global Thresholding and Region Merging. International Journal of Computer Sciences and Engineering, 6(12), 292-297.
BibTex Style Citation:
@article{Singh_2018,
author = {Nidhi Singh, S. Veenadhari},
title = {Breast Cancer Segmentation Using Global Thresholding and Region Merging},
journal = {International Journal of Computer Sciences and Engineering},
issue_date = {12 2018},
volume = {6},
Issue = {12},
month = {12},
year = {2018},
issn = {2347-2693},
pages = {292-297},
url = {https://www.ijcseonline.org/full_paper_view.php?paper_id=3331},
doi = {https://doi.org/10.26438/ijcse/v6i12.292297}
publisher = {IJCSE, Indore, INDIA},
}
RIS Style Citation:
TY - JOUR
DO = {https://doi.org/10.26438/ijcse/v6i12.292297}
UR - https://www.ijcseonline.org/full_paper_view.php?paper_id=3331
TI - Breast Cancer Segmentation Using Global Thresholding and Region Merging
T2 - International Journal of Computer Sciences and Engineering
AU - Nidhi Singh, S. Veenadhari
PY - 2018
DA - 2018/12/31
PB - IJCSE, Indore, INDIA
SP - 292-297
IS - 12
VL - 6
SN - 2347-2693
ER -
![]() |
![]() |
![]() |
486 | 386 downloads | 277 downloads |
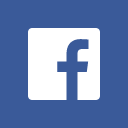
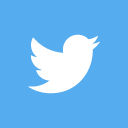
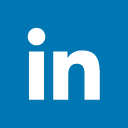
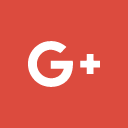
Abstract
Recently, more attention is being given to detect breast cancer in women. But, Due to the lack the diagnostic to suggest whether breast cancer is presented in a person is still a research issue. The proposed work gives a hybrid methodology based on global thresholding and region merging for segmentation of breast cancer in Mammogram Images. In the proposed algorithm we use wiener filtering to remove Gaussian noise then apply image normalization based on histogram shrink to enhance the quality of image. Next, Global thresholding using Otsu’s method is used in order to segment the masses resulting Region of Interest(ROI) and then Region merging is used to extract segmented masses from image. Accuracy rate of the proposed method is 82% and Error rate is only 18%.
Key-Words / Index Term
Breast Cancer, Gaussian Noise, Mammogram Mass, Otsu’s Method, Region Merging
References
[1] Saurabh PrasadLori Mann BruceJohn E Ball,” A Multi-classifier and Decision Fusion Framework for Robust Classification of Mammographic Masses” International Conference of the IEEE Engineering in Medicine and Biology , 2008.
[2] Islam M.J., Ahmadi M., Sid-Ahmed M.A. (2010) Computer-Aided Detection and Classification of Masses in Digitized Mammograms Using Artificial Neural Network. Advances in Swarm Intelligence. ICSI 2010.
[3] S. Baeg, N. Kehtarnavaz,”Texture based classification of mass abnormalities in mammograms”, 13th IEEE Symposium on Computer-Based Medical Systems, 2000..
[4] R. C. Gonzalez and R. E. Woods, Digital Image Processing, 3 ed.: Pearson Prentice Hall, 2007.
[5] A.J. Mendez, P.J. Tahoces, M.J. Lado, M. Souto, J.L. Correa, and J.J. Vidal, J.J, “Automatic Detection of Breast Border and Nipple in Digital Mammograms”, Computer Methods and Programs in Biomedicine, vol. 49, pp. 253–262, 1996.
[6] R. Chandrasekhar, and Y. Attikiouzel, Y. Automatic, “Breast Border Segmentation by Background Modeling and Subtraction”, in: M.J. Yaffe (Ed.), Proceedings of the 5th International Workshop on Digital Mammography (IWDM), Medical Physics Publishing, Toronto, Canada, 2000, pp. 560–565.
[7] M. A. Wirth, A. Stapinski, “Segmentation of the breast region in mammograms using active contours”, in Visual Communications and Image Processing, pp.1995–2006.
[8] Leonardo de Oliveira Martins, E.C. da Silva, A.C. Silva, A. C. de Paiva,” Classification of Breast Masses in Mammogram Images Using Ripley’s K Function and Support Vector Machine“, International Workshop on Machine Learning and Data Mining in Pattern Recognition ,MLDM 2009.
[9] J. Dheeba, N. Albert Singh, S. Tamil Selvi ‘Computer-aided detection of breast cancer on mammograms: A swarm intelligence optimized wavelet neural network approach’, Journal of Biomedical Informatics 49 (2014) 45–52, 2014 Elsevier Inc.
[10] K.Subashini, K.Jeyanthi, ‘Masses detection and classification in ultrasound images’, IOSR Journal of Pharmacy and Biological Sciences (IOSR-JPBS),Volume 9, Issue 3 Ver. II (May -Jun. 2014), PP 48-51
[11] Milos Radovic,Marina Djokovic,Aleksandar Peulic,Nenad Filipovic,”Application of data mining algorithms for mammogram classification”,13th IEEE International Conference on BioInformatics and BioEngineering,2014
[12] F. Jin, P. Fieguth, L. Winger and E. Jernigan,”Adaptive Wiener Filtering Of Noisy Images And Image Sequences ” , IEEE conference 2013.
[13] Otsu N.,“A Threshold Selection Method from Graylevel Histograms”, IEEE Transactions on Systems, Man&Cybernatics, Vol. 9, Issue 1, 1979, pp. 62-66.
[14] [Online] Available: ttp://www.wiau.man.ac.uk.services/MIAS / MIASweb.htm.