A Review of Classification Techniques in Brain Computer Interface
Parag P. Bharne1 , Deepak Kapgate2
Section:Review Paper, Product Type: Journal Paper
Volume-2 ,
Issue-12 , Page no. 68-72, Dec-2014
Online published on Dec 31, 2014
Copyright © Parag P. Bharne , Deepak Kapgate . This is an open access article distributed under the Creative Commons Attribution License, which permits unrestricted use, distribution, and reproduction in any medium, provided the original work is properly cited.
View this paper at Google Scholar | DPI Digital Library
How to Cite this Paper
- IEEE Citation
- MLA Citation
- APA Citation
- BibTex Citation
- RIS Citation
IEEE Citation
IEEE Style Citation: Parag P. Bharne , Deepak Kapgate, “A Review of Classification Techniques in Brain Computer Interface,” International Journal of Computer Sciences and Engineering, Vol.2, Issue.12, pp.68-72, 2014.
MLA Citation
MLA Style Citation: Parag P. Bharne , Deepak Kapgate "A Review of Classification Techniques in Brain Computer Interface." International Journal of Computer Sciences and Engineering 2.12 (2014): 68-72.
APA Citation
APA Style Citation: Parag P. Bharne , Deepak Kapgate, (2014). A Review of Classification Techniques in Brain Computer Interface. International Journal of Computer Sciences and Engineering, 2(12), 68-72.
BibTex Citation
BibTex Style Citation:
@article{Bharne_2014,
author = {Parag P. Bharne , Deepak Kapgate},
title = {A Review of Classification Techniques in Brain Computer Interface},
journal = {International Journal of Computer Sciences and Engineering},
issue_date = {12 2014},
volume = {2},
Issue = {12},
month = {12},
year = {2014},
issn = {2347-2693},
pages = {68-72},
url = {https://www.ijcseonline.org/full_paper_view.php?paper_id=335},
publisher = {IJCSE, Indore, INDIA},
}
RIS Citation
RIS Style Citation:
TY - JOUR
UR - https://www.ijcseonline.org/full_paper_view.php?paper_id=335
TI - A Review of Classification Techniques in Brain Computer Interface
T2 - International Journal of Computer Sciences and Engineering
AU - Parag P. Bharne , Deepak Kapgate
PY - 2014
DA - 2014/12/31
PB - IJCSE, Indore, INDIA
SP - 68-72
IS - 12
VL - 2
SN - 2347-2693
ER -
![]() |
![]() |
![]() |
3966 | 3486 downloads | 3763 downloads |
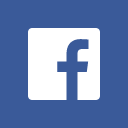
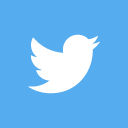
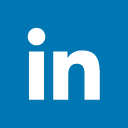
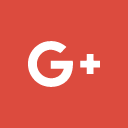
Abstract
In this paper we review the classification algorithm for the Brain Computer Interface. The Characteristics of the EEG signals are changes over time, updating the classifiers of the Brain Computer Interface, (BCI). It will eventually improve the performance of the system. The development of the new adaptive classifiers are not easy because of we cannot predict the intension of the user, in some cases it may be possible to predict the labels of the EEG segments using some information of the state. We briefly present commonly used algorithm and their description of properties. By literature review we presented them in terms of performance. The main point come into Scenario that there is no comprehensive review of signal processing algorithm for EEG Signals. The aim of this review paper is to obtain the limitations and importance of the algorithm is to provide a guideline to the researchers in these fields. Techniques employed for the signal preprocessing, feature extraction and feature classification are discussed, but review focused on signals classification.
Key-Words / Index Term
BCI, EEG, SSVEP-NIRS Hybrid BCI, EEG-EMG Hybrid BCI , EEG-EOG Hybrid BCI
References
[1] Yuanqing Li, Jiahui Pan, Fei Wang, and Zhuliang Yu “A Hybrid BCI System Combining P300 and SSVEP and Its Application to Wheelchair Control”, IEEE Trans. Biomed. Eng, vol. 60, no. 11, pp.1-7, Nov. 2013.
[2] Quan Liu ,Kun Chen, Qingsong Ai, sheng quan xie “Review: recent development of signals processing algorithms for SSVEP based brain computer interface”,Journal of Medical and Biological Engineering, , 12 Aug 2013, pp.299-309, Oct.2014
[3] H. Zhang, C. Guan and C. Wang, “Asynchronous P300-based brain computer interfaces: A computational approach with statistical models,” IEEE Transaction on Biomed. Eng., vol. 55, no. 6, pp. 1754–1763, Jun. 2008.
[4] R. Ortner, B. Allison, G. Korisek, H. Gaggl, and G. Pfurtscheller, “An SSVEP BCI to control a hand orthosis for persons with tetraplegia,” IEEE Trans. Neural Syst. Rehabil. Eng., vol. 19, no. 99, pp. 1–5, Feb. 2011.
[5] Xinyi Yong, Mehrdad Fatourechi, Rabab K. Ward and Gary E. Birch “Adaptive Classification in a Self-Paced Hybrid Brain-Computer Interface System”, 34th Annual International Conference of the IEEE EMBS San Diego, California USA, 28 August - 1 September, 2012
[6] Jinyi Long, Yuanqing Li*, Tianyou Yu, and Zhenghui Gu “Target Selection With Hybrid feature for BCI-Based 2-D Cursor Control”, IEEE Trans. Biomed. Eng, vol. 59, no. 1, pp.1-4, Jan. 2012.
[7] M. Rajya Lakshmi, Dr. T. V. Prasad, Dr. V. Chandra Prakash, “ Survey on EEG Signal Processing Methods ”, International Journal of Advanced Research in Computer Science and Software Engineering, Volume 4, Issue 1, January 2014.
[8] Ali Bashashati, Mehrdad Fatourechi, Rabab K Ward and Gary E Birch, “A survey of signal processing algorithms in brain–computer interfaces based on electrical brain signals”, Journal of Neural Eng. 4 (2007) .pp.32–57, sep. 2014
[9] Luis Fernando Nicolas-Alonso * and Jaime Gomez-Gil, “Review Brain Computer Interfaces, a Review”, Sensors 2012, pp. 1211-1279, sept. 2014.
[10] Setare Amiri, Ahmed Rabbi, Leila Azinfar and Reza Fazel-Rezai, “A Review of P300, SSVEP, and Hybrid P300/SSVEP Brain-Computer Interface Systems”, Intechopen human computer interaction, June 2013, pp1-20, sept.2014.
[11] Setare Amiri, Reza Fazel-Rezai, and Vahid Asadpour, “A Review of Hybrid Brain-Computer Interface Systems”, Advances in Human-Computer Interaction Volume 2013 (2013), Article ID 187024, 8 pages.
[12] Tarik al ani and Salila Trad, “signal processing and classification approaches for brain computer interface”,, Intelligent and Biosensors, Vernon S. Somerset (Ed.), ISBN: 978-953-7619-58-9, InTech, DOI: 10.5772/7032.
[13] Ming Cheng et. Al., “Design and Implementation of a Brain-Computer Interface With High Transfer Rates”, IEEE TRANSACTIONS ON BIOMEDICAL ENGINEERING, VOL. 49, NO. 10, OCTOBER 2002.
[14] F Lotte , M Congedo , A Lécuyer , F Lamarche, “A Review of Classification Algorithms for EEG-based Brain-Computer Interfaces”, citeseerx.
[15] Friday Zinzendoff, Okwonu and Abdul Rahman Othman, “A Model Classification Technique for Linear Discriminant Analysis for Two Groups”, International Journal of Computer Science Issues, Vol. 9, Issue 3, No 2, May 2012.
[16] Minkyu Ahn, Mijin Lee, Jinyoung Choi and Sung Chan Jun, “A Review of Brain-Computer Interface Games and an Opinion Survey from Researchers, Developers and Users”, Sensors 2014.
[17] A. Khorshidtalab, M.J.E. Salami, “EEG Signal Classification for Real-Time Brain-Computer Interface Applications: A Review”, 2011 4th International Conference on Mechatronics (ICOM), 17-19 May 2011
[18] Deon Garrett, David A. Peterson, Charles W. Anderson, and Michael H. Thaut, “Comparison of Linear, Nonlinear, and Feature Selection Methods for EEG Signal Classification” , IEEE transaction. on neural systems and rehabilitation eng, VOL. 11, NO. 2, JUNE 2003.
[19] Mythra H V, Veenakumari H M, Sanjeev Kubakaddi, “ Multi-Class EEG Classification for Brain Computer Interface”, International Journal of Scientific & Engineering Research, Volume 4, Issue 9, September-2013.
[20] Zongyuan Zhao, Shuxiang Xu, Byeong Ho Kang Mir Md Jahangir Kabir, “Investigation of Multilayer Perceptron and Class Imbalance Problems for Credit Rating”, International Journal of Computer and Information Technology ,Volume 03– Issue 04, July 2014