Survey On Semantic Segmentation
P.S.Gunde 1 , S.K.Shirgave 2
Section:Survey Paper, Product Type: Journal Paper
Volume-6 ,
Issue-12 , Page no. 603-606, Dec-2018
CrossRef-DOI: https://doi.org/10.26438/ijcse/v6i12.603606
Online published on Dec 31, 2018
Copyright © P.S.Gunde, S.K.Shirgave . This is an open access article distributed under the Creative Commons Attribution License, which permits unrestricted use, distribution, and reproduction in any medium, provided the original work is properly cited.
View this paper at Google Scholar | DPI Digital Library
How to Cite this Paper
- IEEE Citation
- MLA Citation
- APA Citation
- BibTex Citation
- RIS Citation
IEEE Citation
IEEE Style Citation: P.S.Gunde, S.K.Shirgave, “Survey On Semantic Segmentation,” International Journal of Computer Sciences and Engineering, Vol.6, Issue.12, pp.603-606, 2018.
MLA Citation
MLA Style Citation: P.S.Gunde, S.K.Shirgave "Survey On Semantic Segmentation." International Journal of Computer Sciences and Engineering 6.12 (2018): 603-606.
APA Citation
APA Style Citation: P.S.Gunde, S.K.Shirgave, (2018). Survey On Semantic Segmentation. International Journal of Computer Sciences and Engineering, 6(12), 603-606.
BibTex Citation
BibTex Style Citation:
@article{_2018,
author = {P.S.Gunde, S.K.Shirgave},
title = {Survey On Semantic Segmentation},
journal = {International Journal of Computer Sciences and Engineering},
issue_date = {12 2018},
volume = {6},
Issue = {12},
month = {12},
year = {2018},
issn = {2347-2693},
pages = {603-606},
url = {https://www.ijcseonline.org/full_paper_view.php?paper_id=3386},
doi = {https://doi.org/10.26438/ijcse/v6i12.603606}
publisher = {IJCSE, Indore, INDIA},
}
RIS Citation
RIS Style Citation:
TY - JOUR
DO = {https://doi.org/10.26438/ijcse/v6i12.603606}
UR - https://www.ijcseonline.org/full_paper_view.php?paper_id=3386
TI - Survey On Semantic Segmentation
T2 - International Journal of Computer Sciences and Engineering
AU - P.S.Gunde, S.K.Shirgave
PY - 2018
DA - 2018/12/31
PB - IJCSE, Indore, INDIA
SP - 603-606
IS - 12
VL - 6
SN - 2347-2693
ER -
![]() |
![]() |
![]() |
323 | 262 downloads | 221 downloads |
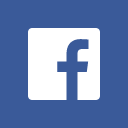
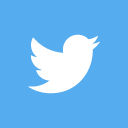
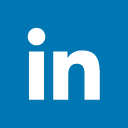
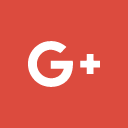
Abstract
The Image segmentation is referred to as one of the most important processes of image processing. Image segmentation is the technique of dividing or partitioning an image into parts, called segments. It is mostly useful for applications like image compression or object recognition, because for these types of applications, it is inefficient to process the whole image. So, image segmentation is used to segment the parts from image for further processing. Semantic image segmentation is a vast area for computer vision and machine learning researchers. Many vision applications need accurate and efficient image segmentation and segment classification mechanisms for assessing the visual contents and perform the real-time decision making. There exist several image segmentation techniques, which partition the image into several parts based on certain image features like pixel intensity value, color, texture, etc. These all techniques are categorized based on the segmentation method used. The application area includes remote sensing, autonomous driving, indoor navigation, video surveillance and virtual or augmented reality systems etc. This survey paper provides a review of different traditional methods of image segmentation.
Key-Words / Index Term
Image segmentation, Conditional Random Field, Deep learning, semantic video segmentation
References
[1]S.Maldonado-Bascon, S.Lafuente-Arroyo, P. Gil-Jimenez, H. Gomez-Moreno, and F. Lopez-Ferreras, “Road-sign detection and recognition based on support vector machines,” Intelligent Transportation Systems, IEEE Transactions on,vol. 8, no. 2, pp. 264–278, Jun. 2007.
[2]N.Moon, E. Bullitt, K. Van Leemput, and G. Gerig, “Automatic brain and tumor segmentation,” in Medical Image Computing and Computer-Assisted Intervention MICCAI 2002. Springer, 2002, pp.372–379.
[3]G.-Q. Wei, K. Arbter, and G. Hirzinger, “Automatic tracking of laparoscopic instruments by color coding,” in CVRMed-MRCAS’97, ser. 1997, vol. 1205, pp. 357–366.
[4]“Memory based active contour algorithm using pixel-level classified images for colon crypt segmentation,” Computerized Medical Imaging and Graphics, Nov. 2014.
[5] C. Huang, L. Davis, and J. Townshend, “An assessment of support vector machines for land cover classification,” International Journal of remote sensing, vol. 23, no. 4, pp. 725–749, 2002.
[6] Krahenbuhl, P. and Koltun, V. E_cient inference in fully connected crfs with gaussian edge potentials.In NIPS, 2011.
[7]Jonathan Long, Evan Shelhamer, Trevor Darrell Fully Convolutional Networks for Semantic Segmentation CVPR 2015 arXiv:1411.4038
[8]B. Hariharan, P. Arbelaez, R. Girshick, and J. Malik. Hypercolumns for object segmentation and _new grained localization. In Computer Vision and Pattern Recognition, 2015
[9]L.C. Chen, G. Papandreou, I. Kokkinos, K. Murphy, and A. L. Yuille. Semantic image segmentation with deep convolutional nets and fully connected crfs. In ICLR, 2015
[10] S. Zheng, S. Jayasumana, B. Romera-Paredes, V. Vineet, Z. Su, D. Du, C. Huang, and P. Torr. “Conditional random fields as recurrent neural networks”. In ICCV, 2015
[11]Kundu, Abhijit, Vineet, Vibhav, and Koltun, Vladlen. “Feature space optimization for semantic video segmentation”. In CVPR, 2016
[12]M. Everingham, L. Van Gool, C.K.I. Williams, J. Winn, A. Zisserman, "The PASCAL Visual Object Classes Challenge 2007 (VOC2007) Results", 2010
[13]G. J. Brostow, J. Fauqueur, and R. Cipolla. “Semantic object classes in video: A high-de nition ground truth database”. Pattern Recognition Letters, 30(2), 2009
[14]M. Cordts, M. Omran, S. Ramos, T. Rehfeld, M. Enzweiler, R. Benenson, U. Franke, S. Roth, and B. Schiele. “The Cityscapes dataset for semantic urban scene understanding”. In CVPR, 2016
[15]A. Geiger, P. Lenz, C. Stiller, and R. Urtasun, “Vision meets robotics The kitti dataset," 2013
[16]A. Geiger, P. Lenz, and R. Urtasun, “Are we ready for autonomous driving? the kitti vision benchmark suite," in CVPR, 2012