Undermining the Fractal and Stationary Nature of Earthquake
Bikash Sadhukhan1 , Somenath Mukherjee2
Section:Research Paper, Product Type: Journal Paper
Volume-6 ,
Issue-12 , Page no. 670-679, Dec-2018
CrossRef-DOI: https://doi.org/10.26438/ijcse/v6i12.670679
Online published on Dec 31, 2018
Copyright © Bikash Sadhukhan, Somenath Mukherjee . This is an open access article distributed under the Creative Commons Attribution License, which permits unrestricted use, distribution, and reproduction in any medium, provided the original work is properly cited.
View this paper at Google Scholar | DPI Digital Library
How to Cite this Paper
- IEEE Citation
- MLA Citation
- APA Citation
- BibTex Citation
- RIS Citation
IEEE Citation
IEEE Style Citation: Bikash Sadhukhan, Somenath Mukherjee, “Undermining the Fractal and Stationary Nature of Earthquake,” International Journal of Computer Sciences and Engineering, Vol.6, Issue.12, pp.670-679, 2018.
MLA Citation
MLA Style Citation: Bikash Sadhukhan, Somenath Mukherjee "Undermining the Fractal and Stationary Nature of Earthquake." International Journal of Computer Sciences and Engineering 6.12 (2018): 670-679.
APA Citation
APA Style Citation: Bikash Sadhukhan, Somenath Mukherjee, (2018). Undermining the Fractal and Stationary Nature of Earthquake. International Journal of Computer Sciences and Engineering, 6(12), 670-679.
BibTex Citation
BibTex Style Citation:
@article{Sadhukhan_2018,
author = {Bikash Sadhukhan, Somenath Mukherjee},
title = {Undermining the Fractal and Stationary Nature of Earthquake},
journal = {International Journal of Computer Sciences and Engineering},
issue_date = {12 2018},
volume = {6},
Issue = {12},
month = {12},
year = {2018},
issn = {2347-2693},
pages = {670-679},
url = {https://www.ijcseonline.org/full_paper_view.php?paper_id=3395},
doi = {https://doi.org/10.26438/ijcse/v6i12.670679}
publisher = {IJCSE, Indore, INDIA},
}
RIS Citation
RIS Style Citation:
TY - JOUR
DO = {https://doi.org/10.26438/ijcse/v6i12.670679}
UR - https://www.ijcseonline.org/full_paper_view.php?paper_id=3395
TI - Undermining the Fractal and Stationary Nature of Earthquake
T2 - International Journal of Computer Sciences and Engineering
AU - Bikash Sadhukhan, Somenath Mukherjee
PY - 2018
DA - 2018/12/31
PB - IJCSE, Indore, INDIA
SP - 670-679
IS - 12
VL - 6
SN - 2347-2693
ER -
![]() |
![]() |
![]() |
536 | 319 downloads | 209 downloads |
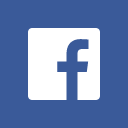
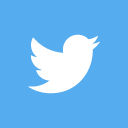
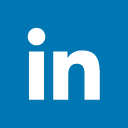
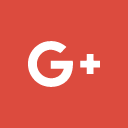
Abstract
In this paper, an investigation has been made to detect the self-similarity and stationarity nature of magnitude of occurred Earthquake by exploring the fractal pattern and the variation nature of frequency of the essential parameter, Magnitude of occurred earthquake across the different place of the world. The time series of magnitude (19.04.1997 to 07.11.2017), of occurred earthquakes, collected from U.S.G.S. have been analysed for exposing the nature of scaling (fractality) and stationary behaviour using different statistical methodologies. Four conventional methods namely Visibility Graph Analysis (VGA), Wavelet Variance Analysis (WVA) Higuchi’s Fractal Dimension (HFD) and Detrended Fluctuation Analysis (DFA) are being used for computing the value of Hurst parameter. In addition, Artificial Neural Network, a pre-trained fully connected 3-layer has finally been used to compute the Hurst parameter. It has been perceived that the specified dataset reveals the anti-persistency and Short-Range Dependency (SRD) behaviour. Binary based ADF, KPSS test and Time Frequency Representation based Smoothed Pseudo Wigner-Ville Distribution (SPWVD) test is incorporated in order to explore the nature of stationarity/non-stationarity of the specified profile, which here displays the non-stationary character of the magnitude of earthquake.
Key-Words / Index Term
Earthquake; Hurst Parameter (H); Fractality; Stationarity; Artificial Neural Network (ANN)
References
[1] E. E. B. Hiroo Kanamori, “The Physics of Earthquakes,” Physics Today, p. 34, June, 2001.
[2] E. Priyadarshini, “An Analysis of the Persistence of Earthquakes in Indonesia using Rescaled Range,” Indian Journal of Science and Technology, vol. 9, no. 21, pp. 1-, 2016.
[3] R. Yulmetyev, F. Gafarov, P. Hanggi, R. Nigmatullin and S. Kayumov, “Possibility between earthquake and explosion seismogram differentiation by discrete stochastic non-Markov processes and local Hurst exponent analysis,” PHYSICAL REVIEW E, vol. 64, no. 066132, pp. 1-14, 2001.
[4] Y. Ogata, “A Prospect of Earthquake Prediction Research,” Statistical Science, vol. 28, no. 4, pp. 521-541, 2013.
[5] G. Preethi and B. Santhi, “Study on Techniques of Earthquake Prediction,” International Journal of Computer Applications, vol. 29, no. 4, pp. 0975 - 8887, 2011.
[6] G. Michas, P. Sammonds and F. Vallianatos, “Dynamic Multifractality in Earthquake Time Series: Insights from the Corinth Rift, Greece,” Pure and Applied Geophysics, vol. 172, no. 7, p. 1909–1921, 2015.
[7] S. Fong and Z. Nannan, “Towards an Adaptive Forecasting of Earthquake Time Series from Decomposable and Salient Characteristics,” in PATTERNS 2011 : The Third International Conferences on Pervasive Patterns and Applications, 2011.
[8] J. B. Panduyos, F. P. Villanueva and R. N. Padua, “Fitting a Fractal Distribution on Philippine Seismic Data: 2011,” SDSSU Multidisciplinary Research Journal, vol. 1, no. 1, pp. 50-58, 2013.
[9] P. k. Dutta, O. P. Mishra and M. K. Naskar, “A Review of Operational Earthquake Forecasting Methodologies Using Linguistic Fuzzy Rule-Based Models From Imprecise Data With Weighted Regression Approach,” Journal of Sustainability Science and Managemen, vol. 8, no. 2, pp. 220-235, 2013.
[10] B. Enescu, Z. R. Struzik and K. Ito, “Wavelet-Based Multifractal Analysis Of Real And Simulated Time Series Of Earthquakes,” Annuals of Disas. Prev. Res. Inst., Kyoto Univ., pp. 1-14, 2004.
[11] K. S. K. Mohankumar, “A Study on Earthquake Prediction Using Neural Network Algorithms,” International Journal of Computer Sciences and Engineering, vol. 6, no. 10, pp. 200 - 204, 2018.
[12] C. Shaganpreet kaur, “Comparative Analysis of Data Mining,” International Journal of Computer Science and Engineering , vol. 6, no. 4, pp. 301-304, 2018.
[13] S. Mukherjee, R. Ray, R. Samanta, K. H. Moffazal and G. Sanyal, “Characterisation of wireless network traffic: Fractality and stationarity,” in ICRCICN 17, IEEE, Kolkata, 2017.
[14] L. Lacasa, B. Luque, J. Luque and J. Nuno, “The visibility graph: Aa new method for estimating the Hurst exponent of fractional Brownian motion,” EPL (Europhysics Letters), vol. 30001, pp. 1-5, 2009.
[15] R. Albert and A. Barabasi, “Statistical mechanics of complex networks.,” Rev Mod Phys, vol. 74(1), pp. 47-97, 2002.
[16] D. Percival and P. Guttorp, “Long Memory Process,the Allan Variance and Wavelets,” pp. 1-15, 1994.
[17] D. Percival, “Estimation of wavelet variance,” pp. 619-631, 1995.
[18] D. Percival and D. Mondal, “M-estimation of wavelet variance,” Elsevier, pp. 623-657, 2012.
[19] R. Ray, M. H. Khondekar, K. Ghosh and A. K. Bhattacharjee, “Memory persistency and nonlinearity in daily mean dew point,” 2015.
[20] T. Higuchi, “Approach to an irregular time series on the basis of the fractal theory,” Physica D: Nonlinear Phenomena, vol. 31, pp. 277-283, 1988.
[21] C. Gomez, A. Mediavilla, R. Hornero, D. Abasolo and A. Fernandez, “Use of the Higuchi`s fractal dimension for the analysis of MEG recordings from Alzheimer`s disease patients.,” Medical engineering & physics, vol. 31, no. 3, pp. 306-13, 4 2009.
[22] M. J. Wairimu, “Features Affecting Hurst Exponent estimation on time series,” in Jomo Kenyatta University Of Agriculture and Technology., Juja, 2013.
[23] K. Hu, P. C. Ivanov, Z. Chen, P. Carpena and H. Eugene Stanley, “Effect of trends on detrended fluctuation analysis,” Phys. Rev. E, vol. 64, no. 1, p. 011114, 6 2001.
[24] C.-K. Peng, S. V. Buldyrev, S. Havlin, M. Simons, H. E. Stanley and A. L. Goldberger, “Mosaic organization of DNA nucleotides,” Phys. Rev. E, vol. 49, no. 2, pp. 1685-1689, 2 1994.
[25] J. W. Kantelhardt, E. Koscielny-Bunde, H. H. A. Rego, S. Havlin and A. Bunde, “Detecting long-range correlations with detrended fluctuation analysis,” Physica A: Statistical Mechanics and its Applications, vol. 295, pp. 441-454, 2001.
[26] S..Ledesma-Orozco, J. Ruiz-Pinales, G. García-Hernández, G. Cerda-Villafaña and D. Hernández-Fusilier, “Hurst Parameter Estimation Using Artificial Neural Networks,” Journal of Applied Research and Technology, vol. 9, p. 15, 2011.
[27] A. L. Maas, A. Y. Hannun and A. Y. Ng, “Rectifier nonlinearities improve neural network acoustic models,” in ICML, 2013.
[28] T. Kocak, “Sigmoid Functions and Their Usage in Artificial Neural Networks,” https://excel.ucf.edu, 2007.
[29] W. contributors, “Mean squared error,” Wikipedia, The Free Encyclopedia, 2018.
[30] T. Tieleman and G. Hinton, “Lecture 6e rmsprop: Divide the gradient by a running average of its recent magnitude,” Coursera, 2012.
[31] “The Augmented Dickey-Fuller Test,” [Online]. Available: https://www.thoughtco.com/the-augmented-dickey-fuller-test-1145985.
[32] H. B. Nielsen, “Non-Stationary Time Series and Unit Root Tests,” [Online]. Available: http://www.econ.ku.dk/metrics/econometrics2_05_ii/slides/08_unitroottests_2pp.pdf.
[33] “Augmented Dickey-Fuller Unit Root Tests,” [Online]. Available: http://faculty.smu.edu/tfomby/eco6375/bj%20notes/adf%20notes.pdf.
[34] D. Kwiatkowski, P. C. B. Phillips, P. Schmidt and Y. Shin, “Testing the null hypothesis of stationarity against the alternative of a unit root: How sure are we that economic time series have a unit root?,” Journal of Econometrics, vol. 54, pp. 159-178, 1992.
[35] W.Wang, Nonlinearity and Forecasting of Streamflow Processes., Stochasticity, 2006.