Ultrasound Medical Image Representation For Systematic Learning
V. Mary Kiruba Rani1 , S.S. Dhenakaran2
Section:Research Paper, Product Type: Journal Paper
Volume-6 ,
Issue-12 , Page no. 930-933, Dec-2018
CrossRef-DOI: https://doi.org/10.26438/ijcse/v6i12.930933
Online published on Dec 31, 2018
Copyright © V. Mary Kiruba Rani, S.S. Dhenakaran . This is an open access article distributed under the Creative Commons Attribution License, which permits unrestricted use, distribution, and reproduction in any medium, provided the original work is properly cited.
View this paper at Google Scholar | DPI Digital Library
How to Cite this Paper
- IEEE Citation
- MLA Citation
- APA Citation
- BibTex Citation
- RIS Citation
IEEE Style Citation: V. Mary Kiruba Rani, S.S. Dhenakaran, “Ultrasound Medical Image Representation For Systematic Learning,” International Journal of Computer Sciences and Engineering, Vol.6, Issue.12, pp.930-933, 2018.
MLA Style Citation: V. Mary Kiruba Rani, S.S. Dhenakaran "Ultrasound Medical Image Representation For Systematic Learning." International Journal of Computer Sciences and Engineering 6.12 (2018): 930-933.
APA Style Citation: V. Mary Kiruba Rani, S.S. Dhenakaran, (2018). Ultrasound Medical Image Representation For Systematic Learning. International Journal of Computer Sciences and Engineering, 6(12), 930-933.
BibTex Style Citation:
@article{Rani_2018,
author = {V. Mary Kiruba Rani, S.S. Dhenakaran},
title = {Ultrasound Medical Image Representation For Systematic Learning},
journal = {International Journal of Computer Sciences and Engineering},
issue_date = {12 2018},
volume = {6},
Issue = {12},
month = {12},
year = {2018},
issn = {2347-2693},
pages = {930-933},
url = {https://www.ijcseonline.org/full_paper_view.php?paper_id=3441},
doi = {https://doi.org/10.26438/ijcse/v6i12.930933}
publisher = {IJCSE, Indore, INDIA},
}
RIS Style Citation:
TY - JOUR
DO = {https://doi.org/10.26438/ijcse/v6i12.930933}
UR - https://www.ijcseonline.org/full_paper_view.php?paper_id=3441
TI - Ultrasound Medical Image Representation For Systematic Learning
T2 - International Journal of Computer Sciences and Engineering
AU - V. Mary Kiruba Rani, S.S. Dhenakaran
PY - 2018
DA - 2018/12/31
PB - IJCSE, Indore, INDIA
SP - 930-933
IS - 12
VL - 6
SN - 2347-2693
ER -
![]() |
![]() |
![]() |
399 | 287 downloads | 164 downloads |
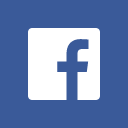
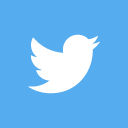
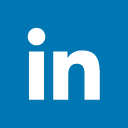
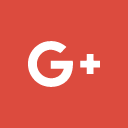
Abstract
Ultrasound screened image is the output screen of ultrasound device. The nature of ultrasound screened image is noisy. These images are produced by sound waves by scanning inside the body. High - Frequency sound waves in the range 1 MHZ to 15 MHZ transmitted from the probe passed through gel to the body and output is produced. Though the technology is improved by detecting the kind of input image format, Ultrasound medical images have a high impact over natural color images since the pixel values range similar. The objective of the article is differentiating computer generated medical images among the collection of images in a dataset. Most of the research approaches have modeled images by its features and detecting it with several images. However, with advance growth in technology, the image quality is better in dimensional effects and thus visually differentiating the images is a significant task. A systematic filtering group of images is the ultimate aim of the work. A number of computer generated medical image in excess of the dataset and the approach starts compares the given digital image to store medical images in the form of key metrics. The values are used to identify medical images. The proposed method has achieved up to 95% of accuracy in identifying ultrasound medical images.
Key-Words / Index Term
Systematic approach, natural image, medical image, classifier, matrix
References
[1] Eric Tokuda, Helio Pedrini , Anderson Rocha, “Computer generated images vs. digital photographs: A synergetic feature and classifier combination approach”, J. Vis. Commun. Image R. 24 (2013) 1276–1292
[2] T.-T. Ng, S.-F. Chang, J. Hsu, L. Xie, M.-P. Tsui, Physics-motivated features for distinguishing photographic images and computer graphics, in: ACM Multimedia (ACMMM), Singapore, 2005, pp. 239–248.
[3] A. da Silva Pinto, H. Pedrini, W.R. Schwartz, A. Rocha, Video-based face spoofing detection through visual rhythm analysis, in: 25th Conference on Graphics, Patterns and Images (SIBGRAPI), Ouro Preto, Brazil, 2012, pp. 221– 228.
[4] T. Pouli, E. Reinhard, Image statistics and their applications in computer graphics, Tech. rep., Eurographics State of the Art Report (STAR), 2010.
[5] E. Dirik, H. Sencar, N. Memon, Source camera identification based on sensor dust characteristics, in: IEEE Signal Processing Applications for Public Security and Forensics (SAFE), USA, 2007, pp. 1–6.
[6] F. Peng, J. Liu, M. Long, Identification of natural images and computer generated graphics based on hybrid features, International Journal of Digital Crime and Forensics (IJDCF) 4 (2013) 1–16.
[7] W. Li, T. Zhang, E. Zheng, X. Ping, Identifying photorealistic computer graphics using second-order difference statistics, in: International Conference on Fuzzy Systems and Knowledge Discovery (FSKD), China, vol. 5, 2010, pp. 2316–2319.
[8] H. Farid, M.J. Bravo, Image forensic analyses that elude the human visual system, in PIE Symposium on Electronic Imaging (SEI), CA, 2010, pp. 754106– 754106–10.