Sarcasm Recognition in Twitter
Sakshi Thakur1 , Sarbjeet Singh2 , Makhan Singh3
Section:Research Paper, Product Type: Journal Paper
Volume-7 ,
Issue-1 , Page no. 241-248, Jan-2019
CrossRef-DOI: https://doi.org/10.26438/ijcse/v7i1.241248
Online published on Jan 31, 2019
Copyright © Sakshi Thakur, Sarbjeet Singh, Makhan Singh . This is an open access article distributed under the Creative Commons Attribution License, which permits unrestricted use, distribution, and reproduction in any medium, provided the original work is properly cited.
View this paper at Google Scholar | DPI Digital Library
How to Cite this Paper
- IEEE Citation
- MLA Citation
- APA Citation
- BibTex Citation
- RIS Citation
IEEE Citation
IEEE Style Citation: Sakshi Thakur, Sarbjeet Singh, Makhan Singh, “Sarcasm Recognition in Twitter,” International Journal of Computer Sciences and Engineering, Vol.7, Issue.1, pp.241-248, 2019.
MLA Citation
MLA Style Citation: Sakshi Thakur, Sarbjeet Singh, Makhan Singh "Sarcasm Recognition in Twitter." International Journal of Computer Sciences and Engineering 7.1 (2019): 241-248.
APA Citation
APA Style Citation: Sakshi Thakur, Sarbjeet Singh, Makhan Singh, (2019). Sarcasm Recognition in Twitter. International Journal of Computer Sciences and Engineering, 7(1), 241-248.
BibTex Citation
BibTex Style Citation:
@article{Thakur_2019,
author = {Sakshi Thakur, Sarbjeet Singh, Makhan Singh},
title = {Sarcasm Recognition in Twitter},
journal = {International Journal of Computer Sciences and Engineering},
issue_date = {1 2019},
volume = {7},
Issue = {1},
month = {1},
year = {2019},
issn = {2347-2693},
pages = {241-248},
url = {https://www.ijcseonline.org/full_paper_view.php?paper_id=3491},
doi = {https://doi.org/10.26438/ijcse/v7i1.241248}
publisher = {IJCSE, Indore, INDIA},
}
RIS Citation
RIS Style Citation:
TY - JOUR
DO = {https://doi.org/10.26438/ijcse/v7i1.241248}
UR - https://www.ijcseonline.org/full_paper_view.php?paper_id=3491
TI - Sarcasm Recognition in Twitter
T2 - International Journal of Computer Sciences and Engineering
AU - Sakshi Thakur, Sarbjeet Singh, Makhan Singh
PY - 2019
DA - 2019/01/31
PB - IJCSE, Indore, INDIA
SP - 241-248
IS - 1
VL - 7
SN - 2347-2693
ER -
![]() |
![]() |
![]() |
638 | 355 downloads | 244 downloads |
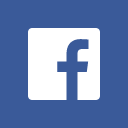
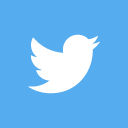
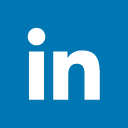
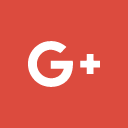
Abstract
Sarcasm is a nuanced form of speech broadly utilized in different online platforms such as social networks, micro-blogs and sarcasm recognition refers to anticipate whether the content is sarcastic or not. Identifying sarcasm in content is among the significant issues confronting sentiment analysis. In sarcasm, individuals express their negative feelings by utilizing positive or strengthened positive words in the content. While talking, individuals regularly utilize intense tonal force and certain gestural pieces of information like rolling of the eyes, hand development, and so forth to reveal sarcasm. Due to these challenges, in the last few decades, researchers have been working rigorously on sarcasm recognition so as to amend the performance of automatic sentiment analysis of data. In this paper, a supervised learning approach, which learns from four different categories of features and their combinations, is presented. These feature sets are employed to classify instances as sarcastic and not-sarcastic using four different classifiers, namely – Naïve Bayes, SVMs, Random Forest and k-Nearest Neighbor classifiers. In particular, it has been tried to explore the impact of sarcastic patterns based on POS tags and the outcomes demonstrate that they are not useful as a feature set for recognizing sarcasm when compared to content words and function words. Using the finest feature set i.e. the combination of content words and function words, a precision and AUC of approximately 85% and 87%, respectively, were achieved. Additionally, the Naïve Bayes classifier gives better results over every single other classifier that has been utilized.
Key-Words / Index Term
Sentiment analysis, Sarcasm, Supervised learning, Feature-sets
References
[1] R. Kumar, V. Ravi. "A survey on opinion mining and sentiment analysis: tasks, approaches and applications", Knowledge-Based Systems, Vol.89, pp.14-46, 2015.
[2] E. Riloff, A. Qadir, P. Surve, L. D. Silva, N. Gilbert, R. Huang, "Sarcasm as contrast between a positive sentiment and negative situation", In Proceedings of the 2013 Conference on Empirical Methods in Natural Language Processing, pp.704-714, 2013.
[3] D. G. Maynard, M. A. Greenwood, "Who cares about sarcastic tweets? investigating the impact of sarcasm on sentiment analysis", In LREC 2014 Proceedings, ELRA, 2014.
[4] Bharti, S. Kumar, K. S. Babu, S. K. Jena, "Parsing-based sarcasm sentiment recognition in twitter data", In Proceedings of the 2015 IEEE/ACM International Conference on Advances in Social Networks Analysis and Mining 2015, ACM, pp. 1373-1380, 2015.
[5] A. Khattri, A. Joshi, P. Bhattacharyya, M. Carman, "Your sentiment precedes you: Using an author’s historical tweets to predict sarcasm", In Proceedings of the 6th workshop on computational approaches to subjectivity, sentiment and social media analysis, pp. 25-30, 2015.
[6] B. Pang, L. Lee, "A sentimental education: Sentiment analysis using subjectivity summarization based on minimum cuts", In Proceedings of the 42nd annual meeting on Association for Computational Linguistics, Association for Computational Linguistics, p.271, 2004.
[7] S. M. Mohammad, P. D. Turney, "Crowdsourcing a word–emotion association lexicon", Computational Intelligence, Vol.29, Issue.3, pp. 436-465, 2013.
[8] R. Justo, T. Corcoran, S. M. Lukin, M. Walker, M. I. Torres, "Extracting relevant knowledge for the detection of sarcasm and nastiness in the social web", Knowledge-Based Systems, Vol.69, pp.124-133, 2014.
[9] S. Mukherjee, P. K. Bala, "Sarcasm detection in microblogs using Naïve Bayes and fuzzy clustering", Technology in Society, Vol.48, pp.19-27, 2017.
[10] M. Bouazizi, T. O. Ohtsuki, "A pattern-based approach for sarcasm detection on Twitter", IEEE Access 4, pp.5477-5488, 2016.
[11] O. Tsur, D. Davidov, A. Rappoport, "ICWSM-A Great Catchy Name: Semi-Supervised Recognition of Sarcastic Sentences in Online Product Reviews", In ICWSM, pp.162-169, 2010.
[12] D. Davidov, O. Tsur, A. Rappoport, "Semi-supervised recognition of sarcastic sentences in twitter and amazon", In Proceedings of the fourteenth conference on computational natural language learning, Association for Computational Linguistics, pp.107-116, 2010.
[13] J. Tepperman, D. Traum, S. Narayanan, "" Yeah Right": Sarcasm Recognition for Spoken Dialogue Systems", In Ninth International Conference on Spoken Language Processing, 2006.
[14] C. C. Liebrecht, F. A. Kunneman, A. P. J. van Den Bosch. "The perfect solution for detecting sarcasm in tweets# not", 2013.
[15] N. Littlestone. "Learning quickly when irrelevant attributes abound: A new linear-threshold algorithm", Machine learning, Vol.2, Issue.4, pp.285-318, 1988.
[16] R. González-Ibánez, S. Muresan, N. Wacholder, "Identifying sarcasm in Twitter: a closer look", In Proceedings of the 49th Annual Meeting of the Association for Computational Linguistics: Human Language Technologies: Short Papers, Association for Computational Linguistics, Vol.2, pp.581-586, 2011.
[17] A. Rajadesingan, R. Zafarani, H. Liu, "Sarcasm detection on twitter: A behavioral modeling approach", In Proceedings of the Eighth ACM International Conference on Web Search and Data Mining, ACM, pp.97-106, 2015.
[18] S. C. Schürer, S. M. Muskal, "Kinome-wide activity modeling from diverse public high-quality data sets", Journal of chemical information and modeling, Vol.53, Issue.1, pp.27-38, 2013.
[19] R. Kohavi, "A study of cross-validation and bootstrap for accuracy estimation and model selection" In Ijcai, Vol.14, Issue.2, pp.1137-1145, 1995.
[20] K. W. Church, "A stochastic parts program and noun phrase parser for unrestricted text", In Proceedings of the second conference on Applied natural language processing, Association for Computational Linguistics, pp.136-143, 1988.
[21] D. Biber, S. Johansson, G. Leech, S. Conrad, E. Finegan, "Longman grammar of spoken and written English", pp.89-110, 1999.
[22] K. P. Murphy, "Naive bayes classifiers", University of British Columbia, 2006.
[23] C. Hsu, C. Chang, C. Lin, "A practical guide to support vector classification", pp.1-16, 2003.
[24] L. Breiman, "Random forests", Machine learning, Vol.45, Issue.1, pp.5-32, 2001.
[25] M. J. Islam, QM Jonathan, M. Ahmadi, M. A. Sid-Ahmed, "Investigating the performance of naive-bayes classifiers and k-nearest neighbor classifiers", In Convergence Information Technology, 2007. International Conference on, IEEE, pp.1541-1546, 2007.
[26] H. Schütze, C. D. Manning, P. Raghavan, “Introduction to information retrieval”, Cambridge University Press, Vol.1, 2008.