Content Based Image Retrivel on Non-Parametric Statistical Tests of Hypothesis
S. Selvaraj1 , K. Seetharaman2
Section:Review Paper, Product Type: Journal Paper
Volume-7 ,
Issue-1 , Page no. 563-568, Jan-2019
CrossRef-DOI: https://doi.org/10.26438/ijcse/v7i1.563568
Online published on Jan 31, 2019
Copyright © S. Selvaraj, K. Seetharaman . This is an open access article distributed under the Creative Commons Attribution License, which permits unrestricted use, distribution, and reproduction in any medium, provided the original work is properly cited.
View this paper at Google Scholar | DPI Digital Library
How to Cite this Paper
- IEEE Citation
- MLA Citation
- APA Citation
- BibTex Citation
- RIS Citation
IEEE Style Citation: S. Selvaraj, K. Seetharaman, “Content Based Image Retrivel on Non-Parametric Statistical Tests of Hypothesis,” International Journal of Computer Sciences and Engineering, Vol.7, Issue.1, pp.563-568, 2019.
MLA Style Citation: S. Selvaraj, K. Seetharaman "Content Based Image Retrivel on Non-Parametric Statistical Tests of Hypothesis." International Journal of Computer Sciences and Engineering 7.1 (2019): 563-568.
APA Style Citation: S. Selvaraj, K. Seetharaman, (2019). Content Based Image Retrivel on Non-Parametric Statistical Tests of Hypothesis. International Journal of Computer Sciences and Engineering, 7(1), 563-568.
BibTex Style Citation:
@article{Selvaraj_2019,
author = {S. Selvaraj, K. Seetharaman},
title = {Content Based Image Retrivel on Non-Parametric Statistical Tests of Hypothesis},
journal = {International Journal of Computer Sciences and Engineering},
issue_date = {1 2019},
volume = {7},
Issue = {1},
month = {1},
year = {2019},
issn = {2347-2693},
pages = {563-568},
url = {https://www.ijcseonline.org/full_paper_view.php?paper_id=3543},
doi = {https://doi.org/10.26438/ijcse/v7i1.563568}
publisher = {IJCSE, Indore, INDIA},
}
RIS Style Citation:
TY - JOUR
DO = {https://doi.org/10.26438/ijcse/v7i1.563568}
UR - https://www.ijcseonline.org/full_paper_view.php?paper_id=3543
TI - Content Based Image Retrivel on Non-Parametric Statistical Tests of Hypothesis
T2 - International Journal of Computer Sciences and Engineering
AU - S. Selvaraj, K. Seetharaman
PY - 2019
DA - 2019/01/31
PB - IJCSE, Indore, INDIA
SP - 563-568
IS - 1
VL - 7
SN - 2347-2693
ER -
![]() |
![]() |
![]() |
431 | 167 downloads | 118 downloads |
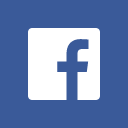
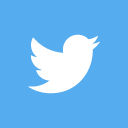
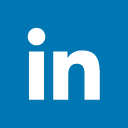
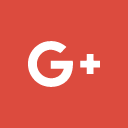
Abstract
The field of image processing is addressed significantly by the role of CBIR. Peculiar query is the main feature on which the image retrieval of content based problems is dependent. Relevant information is required for the submission of sketches or drawing and similar type of features. Many algorithms are used for the extraction of features which are related to similar nature. The process can be optimized by the use of feedback from the retrieval step. Analysis of color and shape can be done by the visual contents of image. Here neural network, Relevance feedback techniques based on image retrieval are discussed.
Key-Words / Index Term
CBIR, Hypothesis, Image Retrival, Image Processing, Extraction
References
[1] J.Huang, S.R. Kunar, M.Mitra, W.J. Zhu, and R.Zabih, Image indexing using color correlogram, in: Proc. IEEE Comp. Soc. Conf. Comp. Vis. and Pattern Recognition, vol. 1, 1997, pp. 762-768.
[2] M.Stricker and M. Orengo, Similarity of color images, in: Storage and Retrieval for Image and Video Databases, Proc. SPIE 2420, vol. 1, 1995, pp. 381-392.
[3] A.Pentland, R.Picard, and S. Sclaroff, Photobook: Content-based manipulation of image databases, International Journal of Computer Vision 18(3) (1996) 233-254.
[4] Chiou-Shaan Fuh, Shun-Wen Cho, and Kai Essig, Hierarchical color image region segmentation for content-based image retrieval system, IEEE Transactions on Image Processing 9(1) (2000) 156-162.
[5] F. Jing, M. Li, H.J. Zhang, and B.Zhang, An efficient and effective region-based image retrieval framework, IEEE Transactions on Image Processing 13(5) (2004) 699-709.
[6] Jun-Wei Hsieh and W.Eric L. Grimson, Spatial template extraction for image retrieval by region matching, IEEE Transactions on Image Processing 12(11) (2003) 1404-1415.
[7] S. Belongie, C.Carson, H.Greenspan, and J.Malik, Recognition of images in large databases using color and texture, IEEE Transactions on Pattern Analysis and Machine Intelligence 24(8) (2002) 1026-1038.
[8] F. Jing, B.Zhang, F.Z.Lin, W.Y.Ma, and H.J.Zhang, A novel region-based image retrieval method using relevance feedback, in: Proc. 3rd ACM Int. Workshop on Multimedia Information Retrieval (MIR), 2001.
[9] Y.Deng and B.S.Manjunath, (1999) “An efficient low-dimensional color indexing scheme for regionbased image retrieval, in: Proc. IEEE Int. Conf. ASSP”, Vol.6, pp. 3017-3020.
[10] Ing-Sheen Hsieh and Huo-Chin Fan, (2001) “Multiple classifiers for color flag and trademark image retrieval”, IEEE Transactions on Image Processing, Vol. 10, No.6, pp. 938-950.
[11] J.R. Smith and C.S. Li, (1999), “Image classification and querying using composite region templates, Journal of Computer Vision and Image Understanding”, Vol. 75, No. 12, pp. 165-174. [12] J.Z. Wang and
[12] Y.P. Du, (2001) “Scalable integrated region-based image retrieval using IRM and statistical clustering”, in: Proc. ACM and IEEE Joint Conference on Digital Libraries, VA, 2001, pp. 268-277.
[13] Rahimi, M, M.H. Alizadeh, R. Rajabi and N. Mehrshad, The Comparison of Innovative Image Processing and Goniometer Methods in Q Angle Measurement ,World Applied Sciences Journal,18(2),2001,226-232.
[14] Rui, Yong. Relevance feedback a power tool for interactive content-based image retrieval, Circuits and Systems for Video Technology, IEEETransactions, 1998, 644-655.
[15] MacArthur, S. D, Brodley, C. E, Kak, A. C, & Broderick, L. S, Interactive content-based image retrieval using relevance feedback, Computer Vision and Image Understanding,88(2), 2002,55- 75 ,2002.
[16] Arevalillo-Herráez, Miguel, and Francesc J.Ferri, An improved distance-based relevance feedback strategy for image retrieval, Image and Vision Computing,31(10),2013,704-713.
[17] da Silva, André Tavares, Jefersson Alex dos Santos, Alexandre Xavier Falcao, Ricardo da S. Torres, and Léo Pini Magalhães, Incorporating multiple distance spaces in optimum-path forest classification to improve feedback-based learning, Computer Vision and Image Understanding ,116 (4),2012,510-523.
[18] Piras, Luca, and Giorgio Giacinto, Synthetic pattern generation for imbalanced learning in image retrieval, Pattern Recognition Letters ,33(16),2012,2198-2205.
[19] Metternich, Michael J, and Marcel Worring, Track based relevance feedback for tracing persons in surveillance videos, Computer Vision and Image Understanding ,117 (3),2013,229-237.
[20] Saneifar, Hassan, Stéphane Bonniol, Pascal Poncelet, and Mathieu Roche, Enhancing passage retrieval in log files by query expansion based on explicit and pseudo relevance feedback, Computers in Industry,2014,1-15.
[21] Wang, Xiang-Yang, Yong-Wei Li, Hong-Ying Yang, and Jing-Wei Chen, An image retrieval scheme with relevance feedback using feature reconstruction and SVM reclassification, Neurocomputing,(127),2014, 214- 230.
[22] Shyu, Mei-Ling, Shu-Ching Chen, Min Chen, and Chengcui Zhang, A unified framework for image database clustering and content-based retrieval, Proceedings. 2nd ACM international workshop. on Multimedia databases, 2004, 19-27.
[23] Quack, Till, Ullrich Mönich, Lars Thiele, and B. S. Manjunath, Cortina: a system for large-scale, content-based web image retrieval, Proceedings.12th annual ACM international .conference on Multimedia, 2004 ,508-511.
[24] Lin, Wei-Hao, Rong Jin, and Alexander Hauptmann, Web image retrieval re-ranking with
[25] relevance model , Proceedings. IEEE/WIC International Conference .on Web Intelligence, 2003,242-248.
[26] Wang, Xin-Jing, et al,Multi-model similarity propagation and its application for web image
[27] retrieval, Proceedings .12th annual ACM international conference. On Multimedia, 2004,944-951.
[28] Schaefer, Gerald, and Michal Stich, UCID: an uncompressed color image database, Proc. SPIE 5307 .on Storage and Retrieval Methods and Applications for Multimedia, 2004, 472-480.
[29] Yu, Hui, Mingjing Li, Hong-Jiang Zhang, and JufuFeng, Color texture moments for content-based image retrieval In Image Processing, Proceedings. International Conference on IEEE, (3), 2002,929- 932.
[30] Kekre, H. B, and Sudeep D. Thepade, Boosting Block Truncation Coding with Kekre`s LUV Color Space for Image Retrieval, International Journal of Electrical, Computer & Systems Engineering,2(3),2008.
[31] Gagaudakis, George, and Paul L. Rosin, Incorporating shape into histograms for CBIR,Pattern Recognition, 35 (1),2002,81-91.
[32] Zhang, Dengsheng, and GuojunLu, Content-Based Shape Retrieval Using Different Shape Descriptors: A Comparative Study, ICME, 2001, 1-4.
[33] Mussarat Yasmin, Muhammad Sharif, Sajjad Mohsin, Image Retrieval Techniques Using Shapes of Object: A Survey, Sci.Int (Lahore), 25(4), 2013, 723-729.
[34] Yasmin, Mussarat, Sajjad Mohsin, Isma Irum, and Muhammad Sharif, Content based image retrieval by shape, color and relevance feedback, Life Science Journal 10 (4s), 2013, 593-598.
[35] Prasad, B. G., K. K. Biswas, and S. K. Gupta,Region-based image retrieval using integrated color, shape, and location index, Computer vision and image understanding ,94 (1),2004,193-233.
[36] Singh, Chandan, Improving image retrieval using combined features of Hough transform and Zernike moments, Optics and Lasers in Engineering ,49(12),2011,1384-1396.
[37] Cheng, Heng-Da, X. H. Jiang, Ying Sun, and Jingli Wang, Color image segmentation advances and prospects, Pattern recognition, 34 (12), 2001,2259-2281.
[38] Qin, Chanchan, Guoping Zhang, Yicong Zhou, Wenbing Tao, and Zhiguo Cao, Integration of the saliency-based seed extraction and random walks for image segmentation, Neurocomputing, (129), 2013, 378–391.
[39] Zhang, Jun, and Lei Ye, Series feature aggregation for content-based image retrieval, Computers & electrical engineering ,36(4), 2010, 691-701.
[40] Seetharaman, K, and S. Sathiamoorthy,Color image retrieval using statistical model and radial basis function neural network, Egyptian Informatics Journal ,15 (1),2014,59-68.
[41] Sánchez-Cruz, Hermilo, and Ernesto Bribiesca, Polygonal approximation of contour shapes using corner detectors, Journal of applied research and technology, 7(3), 2009,275-290.
[42] Tao, Dacheng, Xiaoou Tang, Xuelong Li, and Yong Rui, Direct kernel biased discriminant analysis: a new content-based image retrieval relevance feedback algorithm, Multimedia IEEE Transactions ,8 (4) ,2006,716-727.
[43] Yasmin, Mussarat, Muhammad Sharif, and Sajjad Mohsin, Neural Networks in Medical Imaging Applications: A Survey, World Applied Sciences Journal, 22 (1) ,2013.
[44] Noda, Kuniaki, Hiroaki Arie, Yuki Suga, and Tetsuya Ogata, Multimodal integration learning of robot behavior using deep neural networks, Robotics and Autonomous Systems ,62 (6), 2014.721-736.
[45] Kurtz, Camille, Christopher F. Beaulieu, Sandy Napel, and Daniel L. Rubin, A hierarchical
[46] knowledge-based approach for retrieving similar medical images described with semantic annotations, Journal of biomedical informatics,2014,1-18.
[47] Gao, Chao, Dongguo Zhou, and YongcaiGuo, Automatic iterative algorithm for image segmentation using a modified pulse-coupled neural network, Neurocomputing,(119),2013,332-338.
[48] Son, Le Hoang, Nguyen Duy Linh, and Hoang Viet Long, A lossless DEM compression for fast retrieval method using fuzzy clustering and MANFIS neural network, Engineering Applications of Artificial Intelligence, 2013,33-42.
[49] Humberto Sossn, ElizabethGuevara, Efficient training for dendrite morphological neural networks, Neurocomputing, 2013, 132-142.
[50] Sudipta.Mukhopadhyay, A new content-based image retrieval technique using fuzzy class membership,NeuroComputing, 34(6), 2013,646– 654.
[51] Abrahams, Alan S. Eloise Coupey, Eva X. Zhong, Reza Barkhi, and Pete S. Manasantivongs, Audience targeting by B-to-B advertisement classification: A neural network approach, Expert Systems with Applications, 40,(8),2013,2777- 2791.
[52] Milanova, Mariofanna, Roumen Kountchev, Stuart Rubin, Vladimir Todorov, and Roumiana Kountcheva,Content Based Image Retrieval Using Adaptive Inverse Pyramid Representation, ,In (First Ed) ,Human Interface and the Management of Information and Interaction ,(Springer Berlin Heidelberg, 2009)304-314.
[53] Yasmin, Mussarat, Sajjad Mohsin, and Muhammad Sharif, Intelligent Image Retrieval Techniques A Survey, Journal of Applied Research and Technology,12 (1),2013,87-103.
[54] Seo, Kwang-Kyu , An application of one-class support vector machines in content-based image retrieval, Expert Systems with Applications, 33 (2), 2007,491-498.
[55] Su, Zhong, Hongjiang Zhang, Stan Li, and Shaoping Ma, Relevance feedback in content-based image retrieval Bayesian framework, feature subspaces and progressive learning, Image
[56] Processing IEEE Transactions, 12 (8),2003,924- 937.
[57] Lew, Michael S, Nicu Sebe, Chabane Djeraba, and Ramesh Jain, Content-based multimedia
[58] information retrieval State of the art and challenges, ACM Transactions on Multimedia Computing, Communications, and Applications (TOMCCAP), 2 (1), 2006,1-19.
[59] K. Seetharaman, M. Jeyakarthic, Statistical distributional approach for scale and rotation invariant color image retrieval using multivariate parametric tests and orthogonality condition, Journal of Visual Communication Image Representation, 25(5), 2014, pp. 727- 739.