A Survey on Different Data Mining Techniques for Crop Yield Prediction
R. Beulah1
Section:Survey Paper, Product Type: Journal Paper
Volume-7 ,
Issue-1 , Page no. 738-744, Jan-2019
CrossRef-DOI: https://doi.org/10.26438/ijcse/v7i1.738744
Online published on Jan 31, 2019
Copyright © R. Beulah . This is an open access article distributed under the Creative Commons Attribution License, which permits unrestricted use, distribution, and reproduction in any medium, provided the original work is properly cited.
View this paper at Google Scholar | DPI Digital Library
How to Cite this Paper
- IEEE Citation
- MLA Citation
- APA Citation
- BibTex Citation
- RIS Citation
IEEE Style Citation: R. Beulah , “A Survey on Different Data Mining Techniques for Crop Yield Prediction,” International Journal of Computer Sciences and Engineering, Vol.7, Issue.1, pp.738-744, 2019.
MLA Style Citation: R. Beulah "A Survey on Different Data Mining Techniques for Crop Yield Prediction." International Journal of Computer Sciences and Engineering 7.1 (2019): 738-744.
APA Style Citation: R. Beulah , (2019). A Survey on Different Data Mining Techniques for Crop Yield Prediction. International Journal of Computer Sciences and Engineering, 7(1), 738-744.
BibTex Style Citation:
@article{Beulah_2019,
author = {R. Beulah },
title = {A Survey on Different Data Mining Techniques for Crop Yield Prediction},
journal = {International Journal of Computer Sciences and Engineering},
issue_date = {1 2019},
volume = {7},
Issue = {1},
month = {1},
year = {2019},
issn = {2347-2693},
pages = {738-744},
url = {https://www.ijcseonline.org/full_paper_view.php?paper_id=3576},
doi = {https://doi.org/10.26438/ijcse/v7i1.738744}
publisher = {IJCSE, Indore, INDIA},
}
RIS Style Citation:
TY - JOUR
DO = {https://doi.org/10.26438/ijcse/v7i1.738744}
UR - https://www.ijcseonline.org/full_paper_view.php?paper_id=3576
TI - A Survey on Different Data Mining Techniques for Crop Yield Prediction
T2 - International Journal of Computer Sciences and Engineering
AU - R. Beulah
PY - 2019
DA - 2019/01/31
PB - IJCSE, Indore, INDIA
SP - 738-744
IS - 1
VL - 7
SN - 2347-2693
ER -
![]() |
![]() |
![]() |
1384 | 750 downloads | 196 downloads |
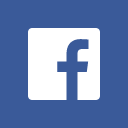
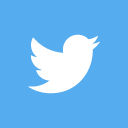
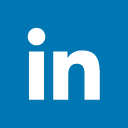
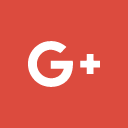
Abstract
Crop growing is measured as the stamina of India, is the improvement of plant for foodstuff, bio-fuel, counteractive plants and other harvest for behind and enhancing human life. Farming is an unique business crop creation which is contingent on different attributes such as soil, climate, irrigation, precipitation, insect killer weeds, fertilizers, nurturing, temperature, harvesting and other factors. An accurate crop yield prediction helps support decision makers in the agriculture sector to envisage the yield effectively. Data mining techniques play a vital role in the study of data for crop yield prediction. Data mining is the computing method of discovering patterns in hefty datasets involving methods at the connection of machine learning, artificial intelligence, record and system database. This piece of writing presents a detailed examination of various techniques planned for crop yield prediction. At first, dissimilar techniques developed by previous researchers are calculated in detail. Then, a relative analysis is carried out to know the precincts of each technique and afford a suggestion for further enhancement in crop yield prediction successfully.
Key-Words / Index Term
Agriculture, crop yield prediction, data mining, machine learning technique
References
[1] Bhanose, S. S., Bogawar, K. A., Dhotre, A. G., & Gaidhani, B. R. “Crop and Yield Prediction Model”, International Journal of Advance Scientific Research and Engineering Trends, 1(1), pp.23-28, 2016.
[2] Ramesh, D., & Vardhan, B. V. “Analysis of crop yield prediction using data mining techniques”, International Journal of Research in Engineering and Technology, 4(1), pp.47-473, 2015
[3] Gandhi, N., Armstrong, L. J., & Petkar, O. “Predicting Rice crop yield using Bayesian networks”, In Advances in Computing, Communications and Informatics (ICACCI), 2016 International Conference on pp. 795-799, 2016.
[4] Shastry, K. A., Sanjay, H. A., & Deshmukh, A. “A Parameter Based Customized Artificial Neural Network Model for Crop Yield Prediction”, Journal of Artificial Intelligence, vol,9, pp. 23-32, 2016.
[5] Pantazi, X. E., Moshou, D., Alexandridis, T., Whetton, R. L., & Mouazen, A. M. “Wheat yield prediction using machine learning and advanced sensing techniques”, Computers and Electronics in Agriculture, 121, pp.57-65, 2016.
[6] Wang, J., Li, Z., Qin, X., Yang, X., Gao, Z., & Qin, Q. (2014, July). “Hyperspectral predicting model of soil salinity in Tianjin costal area using partial least square regression”, In Geoscience and Remote Sensing Symposium (IGARSS), 2014 IEEE International pp. 3251-3254, 2014.
[7] Liu, H. L., Liu, H. B., Lei, Q. L., Zhai, L. M., Wang, H. Y., Zhang, J. Z., ... & Liu, X. X. “Using the DSSAT model to simulate wheat yield and soil organic carbon under a wheat-maize cropping system in the North China Plain” Journal of Integrative Agriculture, 16(10), pp. 2300-2307, 2017.
[8] Garg, B., Aggarwal, S., & Sokhal, J. “Crop yield forecasting using fuzzy logic and regression model”. Computers & Electrical Engineering, 2017.
[9] Jeong, J. H., Resop, J. P., Mueller, N. D., Fleisher, D. H., Yun, K., Butler, E. E., ... & Kim, S. H. “Random forests for global and regional crop yield predictions”, PLoS One, 11(6), e0156571. 2016.
[10] Villanueva, M. B., & Salenga, M. L. M. “Bitter Melon Crop Yield Prediction using Machine Learning Algorithm”, International Journal of Advanced Computer Science and Applications (IJACSA), 9 (3), pp.1-6, 2018
[11] Brdar, S., Culibrk, D., Marinkovic, B., Crnobarac, J., & Crnojevic, V. (2011, “Support vector machines with features contribution analysis for agricultural yield prediction. In Second International Workshop on Sensing Technologies in Agriculture”, Forestry and Environment (EcoSense 2011), Belgrade, Serbia pp. pp.43-47, 2011.
[12] Huang, X., Huang, G., Yu, C., Ni, S., & Yu, L. “A multiple crop model ensemble for improving broad-scale yield prediction using Bayesian model averaging”, Field Crops Research, 211, pp.114-124, 2017.
[13] Asha Patil, Kalyani Patil, Kalpesh Lad, “Leaf Disease detection using Image Processing Techniques”, International Journal of Scientific Research in Computer Science and Engineering” Vol:6 pp.33-36, 2018.