Speech Corpora, Feature Extraction Techniques and Classifiers with Special Reference to Automatic Speech Recognition
D. Dutta1 , R.D.Choudhury 2 , S.Gogoi 3
Section:Survey Paper, Product Type: Journal Paper
Volume-7 ,
Issue-2 , Page no. 372-378, Feb-2019
CrossRef-DOI: https://doi.org/10.26438/ijcse/v7i2.372378
Online published on Feb 28, 2019
Copyright © D. Dutta, R.D.Choudhury, S.Gogoi . This is an open access article distributed under the Creative Commons Attribution License, which permits unrestricted use, distribution, and reproduction in any medium, provided the original work is properly cited.
View this paper at Google Scholar | DPI Digital Library
How to Cite this Paper
- IEEE Citation
- MLA Citation
- APA Citation
- BibTex Citation
- RIS Citation
IEEE Style Citation: D. Dutta, R.D.Choudhury, S.Gogoi, “Speech Corpora, Feature Extraction Techniques and Classifiers with Special Reference to Automatic Speech Recognition,” International Journal of Computer Sciences and Engineering, Vol.7, Issue.2, pp.372-378, 2019.
MLA Style Citation: D. Dutta, R.D.Choudhury, S.Gogoi "Speech Corpora, Feature Extraction Techniques and Classifiers with Special Reference to Automatic Speech Recognition." International Journal of Computer Sciences and Engineering 7.2 (2019): 372-378.
APA Style Citation: D. Dutta, R.D.Choudhury, S.Gogoi, (2019). Speech Corpora, Feature Extraction Techniques and Classifiers with Special Reference to Automatic Speech Recognition. International Journal of Computer Sciences and Engineering, 7(2), 372-378.
BibTex Style Citation:
@article{Dutta_2019,
author = {D. Dutta, R.D.Choudhury, S.Gogoi},
title = {Speech Corpora, Feature Extraction Techniques and Classifiers with Special Reference to Automatic Speech Recognition},
journal = {International Journal of Computer Sciences and Engineering},
issue_date = {2 2019},
volume = {7},
Issue = {2},
month = {2},
year = {2019},
issn = {2347-2693},
pages = {372-378},
url = {https://www.ijcseonline.org/full_paper_view.php?paper_id=3671},
doi = {https://doi.org/10.26438/ijcse/v7i2.372378}
publisher = {IJCSE, Indore, INDIA},
}
RIS Style Citation:
TY - JOUR
DO = {https://doi.org/10.26438/ijcse/v7i2.372378}
UR - https://www.ijcseonline.org/full_paper_view.php?paper_id=3671
TI - Speech Corpora, Feature Extraction Techniques and Classifiers with Special Reference to Automatic Speech Recognition
T2 - International Journal of Computer Sciences and Engineering
AU - D. Dutta, R.D.Choudhury, S.Gogoi
PY - 2019
DA - 2019/02/28
PB - IJCSE, Indore, INDIA
SP - 372-378
IS - 2
VL - 7
SN - 2347-2693
ER -
![]() |
![]() |
![]() |
623 | 436 downloads | 157 downloads |
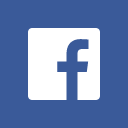
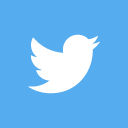
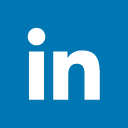
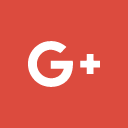
Abstract
in the current years, speech recognition has emerged as an important research area. To carry out further research on automatic speech recognition, a comprehensive review of existing work in this domain stands useful and constructive for the researchers. This paper has presented a recent literature review on speech recognition considering various existing speech corpora, speech features and different models or classifiers used in speech recognition. Different speech databases have been compared in terms of the number of speakers, type of speakers such as native or acted, age and gender of speakers and speech recording environment. Various techniques for speech signal acquisition and pre-processing of the speech signals are also addressed in this work.
Key-Words / Index Term
Automatic speech recognition, boundary detection, Feature-extraction, classifier, Mel-Frequency coefficient, phonemes, Speech Filter
References
[1] Mohamed, Abdel-Rahman, George Dahl, and Geoffrey Hinton. "Deep belief networks for phone recognition." Nips workshop on deep learning for speech recognition and related applications. Vol. 1. No. 9. 2009.
[2] Graves, Alex, Abdel-rahman Mohamed, and Geoffrey Hinton. "Speech recognition with deep recurrent neural networks." In Acoustics, speech and signal processing (icassp), 2013 ieee international conference on, pp. 6645-6649. IEEE, 2013.
[3] Hinton, Geoffrey, Li Deng, Dong Yu, George E. Dahl, Abdel-rahman Mohamed, Navdeep Jaitly, Andrew Senior et al. "Deep neural networks for acoustic modeling in speech recognition: The shared views of four research groups." IEEE Signal processing magazine 29, no. 6 (2012): 82-97.
[4] Cooke, Martin, et al. "An audio-visual corpus for speech perception and automatic speech recognition." The Journal of the Acoustical Society of America 120.5 (2006): 2421-2424.
[5] Yu, Dong, Li Deng, and George Dahl. "Roles of pre-training and fine-tuning in context-dependent DBN-HMMs for real-world speech recognition." In Proc. NIPS Workshop on Deep Learning and Unsupervised Feature Learning. 2010.
[6] Mari, J-F., J-P. Haton, and Abdelaziz Kriouile. "Automatic word recognition based on second-order hidden Markov models." IEEE Transactions on speech and Audio Processing 5, no. 1 (1997): 22-25.
[7] Rabiner, L. R., and J. G. Wilpon. "Some performance benchmarks for isolated word speech recognition systems." Computer Speech & Language 2.3-4 (1987): 343-357.
[8] Utpal Bhattacharjee, "A comparative study of LPCC and MFCC features for the recognition of Assamese phonemes." International Journal of Engineering Research and Technology2,
[9] Wilpon and Jay G., "Automatic recognition of keywords in unconstrained speech using hidden Markov models." In IEEE Transactions on Acoustics, Speech, and Signal Processing38.11 (1990): 1870-1878.
[10] Rabiner, L. R., S. E. Levinson, and M. M. Sondhi. "On the use of hidden Markov models for speaker-independent recognition of isolated words from a medium-size vocabulary." AT&T Bell Laboratories Technical Journal 63.4 (1984): 627-642.
[11] Dahl and George E., "Context-dependent pre-trained deep neural networks for large-vocabulary speech recognition." IEEE Transactions on audio, speech, and language processing 20.1 (2012): 30-42.
[12] S. F. Boll, "Suppression of Acoustic Noise in Speech Using Spectral Subtraction," IEEE Transactions on Acoustics, Speech, and Signal Processing, vol. ASSP-27, no. 2, pp. 113–120, 1979.
[13] M. Karam, H. F. Khazaal, H. Aglan and C. Cole, “Noise removal in speech processing using spectral subtraction”, Journal of Signal and Information Processing, 2014.
[14] A. Agarwal and Y. M. Cheng, “Two-stage Mel-warped Wiener filter for robust speech recognition”, In Proc. ASRU, vol. 99, pp. 67-70, 1999.
[15] G. R. Babu and R. Rao, “Modified Kalman Filter-based Approach in Comparison with Traditional Speech Enhancement Algorithms from Adverse Noisy Environments”, International Journal on Computer Science and Engineering, vol. 3, no. 2, pp. 744-759, 2011.
[16] S.Gogoi and U. Bhattacharjee, “Vocal tract length normalization and sub-band spectral subtraction based robust Assamese vowel recognition system,” In IEEE International Conference on Computing Methodologies and Communication (ICCMC), IEEE, pp. 32-35, 2017.
[17] D. Giuliani, M. Gerosa, and F. Brugnara, "Improved automatic speech recognition through speaker normalization," Computer Speech & Language, vol. 20, no. 1, pp. 107–123, 2006.
[18] L. Lee and R. C. Rose, "Speaker normalization using efficient frequency warping procedures," in IEEE International Conference on Acoustics, Speech, and Signal Processing, IEEE, vol. 1, 1996.
[19] J. Lung et al., "Implementation of Vocal Tract Length Normalization for Phoneme Recognition on TIMIT Speech Corpus," in International Conference on Information Communication and Management, Singapore: IPCSIT, pp. 136–140, 2011.
[20] B . Widmer, "Implementation of Vocal Tract Length Normalization A Study of Methods". [Online]. Available: http : // ssli.ee.washington.edu / people / bwidmer /VTL_Talk/VTL_Talk.PDF. Accessed: 2013.
[21] S. Gogoi and U. Bhattacharjee, "Impact of Vocal Tract Length Normalization on the Speech Recognition Performance of an English Vowel Phoneme Recognizer for the Recognition of Children Voices," International Journal of Computer Trends and Technology (IJCTT), vol. 39, no. 2, pp. 105–109, 2016. [Online]. Available: http://www.ijcttjournal.org/2016/Volume39/number-2/IJCTT-V39P118.pdf. Accessed: Oct. 1, 2016.
[22] G. Garau, S. Renals, and T. Hain, "Applying Vocal Tract Length Normalization to Meeting Recordings," 2005. [Online]. Available: http://www.cstr.ed.ac.uk/downloads/ publications/2005/giuliagarau_eurospeech05.pdf.
[23] H. Jiang, K. Hirose and Q. Hue, “A minimax search algorithm for robust continuous speech recognition,” IEEE Transactions on Speech and Audio Processing, 8(6): 688–694, 2000.
[24] B. Nasersharif and A. Akbari, “Improved HMM entropy for robust sub-band speech recognition,” in13th European In Signal Processing Conference, IEEE, pp. 1–4, 2005.
[25] H. Xu, et al. , “Noise Condition-Dependent Training Based on Noise Classification and SNR Estimation,” IEEE Transactions on Audio, Speech, and Language Processing, 15(8): 2431–2443, 2007.
[26] O. Kalinli, et al., “Noise adaptive training for robust automatic speech recognition,” IEEE Transactions on Audio, Speech, and Language Processing, 18(8): 1889–1901, 2010.
[27] J. Ganitkevitch, “Speaker adaptation using maximum likelihood linear regression,”: Rheinish-Westflesche Technische Hochschule Aachen, the course of Automatic Speech Recognition, www-i6. informatik. rwthaachen. : [Online]. Available: http://www.cs.jhu.edu/~juri/pdf/mllr-rwth-2005.pdf. , 2015.
[28] Muda, Lindasalwa, Mumtaj Begam, and Irraivan Elamvazuthi. "Voice recognition algorithms using mel frequency cepstral coefficient (MFCC) and dynamic time warping (DTW) techniques." arXiv preprint arXiv:1003.4083 (2010).
[29] Ghai, Wiqas, and Navdeep Singh. "Analysis of automatic speech recognition systems for indo-aryan languages: Punjabi a case study." Int J Soft Comput Eng 2.1 (2012): 379-385.
[30] Mousmita Sarma, and Kandarpa Kumar Sarma. "Segmentation and classification of vowel phonemes of assamese speech using a hybrid neural framework." Applied Computational Intelligence and Soft Computing 2012, 2012.sd
[31] Kwon, Oh-Wook, Kwokleung Chan, and Te-Won Lee. Speech feature analysis using variational Bayesian PCA. IEEE Signal Processing Letters 10, no. 5. 2003, pp.137-140.
[32] Mousmita Sarma, Krishna Dutta, and Kandarpa Kumar Sarma. "Speech corpus of assamese numerals extracted using an adaptive pre-emphasis filter for speech recognition." In Computer and Communication Technology (ICCCT), 2010 International Conference on, IEEE, 2010, pp. 461-466.
[33] Mousmita Sarma, Krishna Dutta, and Kandarpa Kumar Sarma. "Speech corpus of assamese numerals extracted using an adaptive pre-emphasis filter for speech recognition." In Computer and Communication Technology (ICCCT), 2010 International Conference on, IEEE, 2010, pp. 461-466.
[34] Mohammadi, Seyed Hamidreza, and Alexander Kain. "Voice conversion using deep neural networks with speaker-independent pre-training." In Spoken Language Technology Workshop (SLT),IEEE, 2014, pp.19-23.
[35] Hasegawa-Johnson, Mark, Jon Gunderson, Adrienne Perlman, and Thomas Huang. "HMM-based and SVM-based recognition of the speech of talkers with spastic dysarthria." In Acoustics, Speech and Signal Processing, 2006. ICASSP 2006 Proceedings. IEEE International Conference on, vol. 3, pp. III-III. IEEE, 2006.
[36] Morgan, Nelson, and Herve Bourlard. "Continuous speech recognition using multilayer perceptrons with hidden Markov models." Acoustics, Speech, and Signal Processing, 1990. ICASSP-90., 1990 International Conference on. IEEE, 1990.
[37] Renals and Steve, "Connectionist probability estimators in HMM speech recognition." IEEE Transactions on Speech and Audio Processing 2.1 (1994): 161-174.
[38] Robinson, A. J., Cook, G. D., Ellis, D. P., Fosler-Lussier, E., Renals, S. J., & Williams, D. A. G. Connectionist speech recognition of broadcast news. Speech Communication, 2002. Pp. 37(1-2), 27-45.
[39] Mohamed, Abdel-Rahman, George Dahl, and Geoffrey Hinton. "Deep belief networks for phone recognition." Nips workshop on deep learning for speech recognition and related applications. Vol. 1. No. 9. 2009.
[40] Mohamed, Abdel-Rahman, Dong Yu, and Li Deng. "Investigation of full-sequence training of deep belief networks for speech recognition." Eleventh Annual Conference of the International Speech Communication Association. 2010.
[41] Dahl, George, Abdel-Rahman Mohamed, and Geoffrey E. Hinton. "Phone recognition with the mean-covariance restricted Boltzmann machine." Advances in neural information processing systems. 2010.
[42] Mohamed, Abdel-Rahman, George E. Dahl, and Geoffrey Hinton. "Acoustic modeling using deep belief networks." IEEE Transactions on Audio, Speech, and Language Processing20.1 (2012): 14-22.
[43] Shaoqin, Yao, and Zhang Linghua. "Voice Conversion Based on Mixed GMM-ANN Model." Journal of Data Acquisition and Processing 2, 2014.
[44] Mridusmita Sharma, and Kandarpa Kumar Sarma. "Dialectal Assamese vowel speech detection using acoustic phonetic features, KNN and RNN." In Signal Processing and Integrated Networks (SPIN), 2015 2nd International Conference on, pp. 674-678. IEEE, 2015.
[45] Acero and Alex, "Live search for mobile: Web services by voice on the cell phone." Acoustics, Speech and Signal Processing, 2008. ICASSP 2008. IEEE International Conference on. IEEE, 2008.
[46] Morgan and Nelson, "Pushing the envelope-aside [speech recognition]." IEEE Signal Processing Magazine 22.5 (2005): 81-88.
[47] Hwang, Mei-Yuh, and Xuedong Huang. "Shared-distribution hidden Markov models for speech recognition." IEEE Transactions on Speech and Audio Processing 1.4 (1993): 414-420.
[48] Rabiner and L. R., "Recognition of isolated digits using hidden Markov models with continuous mixture densities." Bell Labs Technical Journal 64.6 (1985): 1211-1234.
[49] Dahl, George, Abdel-Rahman Mohamed, and Geoffrey E. Hinton. "Phone recognition with the mean-covariance restricted Boltzmann machine." Advances in neural information processing systems. 2010.
[50] Hennebert and Jean, "Estimation of global posteriors and forward-backward training of hybrid HMM/ANN systems." (1997).
[51] Franzini M, Lee KF, Waibel A. Connectionist Viterbi training: a new hybrid method for continuous speech recognition. Acoustics, Speech, and Signal Processing, ICASSP-90., International Conference, IEEE, 1990, pp. 425-428.sd
[52] Levin E. Word recognition using hidden control neural architecture. InAcoustics, Speech, and Signal Processing, ICASSP-90., International Conference, IEEE, 1990, pp.433-436.
[53] Morgan, Nelson, and Herve Bourlard. Continuous speech recognition using multilayer perceptrons with hidden Markov models. In Acoustics, Speech, and Signal Processing, ICASSP-90.,International Conference, IEEE, 1990, pp.413-416.
[54] Niles, Les T., and Harvey F. Silverman. Combining hidden Markov model and neural network classifiers. In Acoustics, Speech, and Signal Processing, ICASSP-90., International Conference, IEEE, pp. 417-420.s
[55] Trentin, Edmondo, and Marco Gori. A survey of hybrid ANN/HMM models for automatic speech recognition. Neurocomputing 37, no. 1-4, 2001, pp. 91-126.
[56] Haffner P, Franzini M, Waibel A. Integrating time alignment and neural networks for high performance continuous speech recognition. InAcoustics, Speech, and Signal Processing, ICASSP-91., International Conference, IEEE, 1991, pp.105-108.
[57] Bengio, Yoshua, Renato De Mori, Giovanni Flammia, and Ralf Kompe. Global optimization of a neural network-hidden Markov model hybrid. IEEE transactions on Neural Networks3, no. 2, 1992, pp.252-259.
[58] Lang, K. J. The development of the time-delay neural network architecture for speech recognition. Technical Report CMU-CS, 1988, pp. 88-152.
[59] Mridusmita Sharma, Mousmita Sarma, and Kandarpa Kumar Sarma. "Recurrent Neural Network based approach to recognize assamese vowels using experimentally derived acoustic-phonetic features." In Emerging Trends and Applications in Computer Science (ICETACS), 2013 1st International Conference on,. IEEE, 2013, pp. 140-143.
[60] Patgiri, Chayashree, Mousmita Sarma, and Kandarpa Kumar Sarma. "Recurrent neural network based approach to recognize assamese fricatives using experimentally derived acoustic-phonetic features." In Emerging Trends and Applications in Computer Science (ICETACS), IEEE, 2013, pp. 33-37.
[61] Lippmann, R., Speech recognition by machines and humans. Speech Communication 22 (1), 1997. PP. 1–15.
[62] Van Leeuwen, D.A., van den Berg, L.G., Steeneken, H.J.M. Human benchmarks for speaker independent large vocabulary recognition performance. In: Proceedings of Euro speech, Madrid, Spain, 1995 pp. 1461– 1464
[63] Meyer, B., Wesker, T., Brand, T., Mertins, A., Kollmeier, B. A human–machine comparison in speech recognition based on a logatome corpus. In: Proceedings of the Workshop on Speech Recognition and Intrinsic Variation, Toulouse, France, 2006.
[64] Sroka, J.J., Braida, L.D. Human and machine consonant recognition. Speech Communication 45, 2005. pp. 401–423.
[65] Moore, R.K. There’s no data like more data: but when will enough be enough? In: Proceedings of the Acoustics Workshop on Innovations in Speech Processing, vol. 23 (3), Stratford-upon-Avon, UK, 2001. pp. 19– 26.
[66] Moore, R.K. A comparison of the data requirements of automatic speech recognition systems and human listeners. In: Proceedings of Euro speech, Geneva, Switzerland, 2003, pp. 2581–2584.
[67] Cooke, M. A glimpsing model of speech recognition in noise. Journal of the Acoustical Society of America 119 (3), 2006. pp. 1562–1573.
[68] Cutler, A., Robinson, T. Response time as a metric for comparison of speech recognition by humans and machines. In J. Clerk Maxwell, A Treatise on Electricity and Magnetism, 3rd ed., vol. 2. Oxford: Clarendon, 1892, Conference of the International Speech Communication Association. 2010, pp.68–73.
[69] Sujatha N. and Prakash K. An Efficient and Scalable Auto Recommender System Based on Users Behavior in Isroset-Journal (IJSRCSE) Vol.6 , Issue.6 , pp.35-40, Dec-2018.
[70] Mutkule Prasad R, Interactive Clothing based on IoT using QR code and Mobile Application Journal (IJSRNSC) , Vol.6 , Issue.6 , pp.1-4, Dec-2018.