An Efficient Approach on Big Data for Stock Prediction with the Aid of Optimal Machine Learning Approach
T. P Sameerapallavi1 , B. Manjula2
Section:Review Paper, Product Type: Journal Paper
Volume-7 ,
Issue-2 , Page no. 482-486, Feb-2019
CrossRef-DOI: https://doi.org/10.26438/ijcse/v7i2.482486
Online published on Feb 28, 2019
Copyright © T. P Sameerapallavi, B. Manjula . This is an open access article distributed under the Creative Commons Attribution License, which permits unrestricted use, distribution, and reproduction in any medium, provided the original work is properly cited.
View this paper at Google Scholar | DPI Digital Library
How to Cite this Paper
- IEEE Citation
- MLA Citation
- APA Citation
- BibTex Citation
- RIS Citation
IEEE Style Citation: T. P Sameerapallavi, B. Manjula, “An Efficient Approach on Big Data for Stock Prediction with the Aid of Optimal Machine Learning Approach,” International Journal of Computer Sciences and Engineering, Vol.7, Issue.2, pp.482-486, 2019.
MLA Style Citation: T. P Sameerapallavi, B. Manjula "An Efficient Approach on Big Data for Stock Prediction with the Aid of Optimal Machine Learning Approach." International Journal of Computer Sciences and Engineering 7.2 (2019): 482-486.
APA Style Citation: T. P Sameerapallavi, B. Manjula, (2019). An Efficient Approach on Big Data for Stock Prediction with the Aid of Optimal Machine Learning Approach. International Journal of Computer Sciences and Engineering, 7(2), 482-486.
BibTex Style Citation:
@article{Sameerapallavi_2019,
author = {T. P Sameerapallavi, B. Manjula},
title = {An Efficient Approach on Big Data for Stock Prediction with the Aid of Optimal Machine Learning Approach},
journal = {International Journal of Computer Sciences and Engineering},
issue_date = {2 2019},
volume = {7},
Issue = {2},
month = {2},
year = {2019},
issn = {2347-2693},
pages = {482-486},
url = {https://www.ijcseonline.org/full_paper_view.php?paper_id=3691},
doi = {https://doi.org/10.26438/ijcse/v7i2.482486}
publisher = {IJCSE, Indore, INDIA},
}
RIS Style Citation:
TY - JOUR
DO = {https://doi.org/10.26438/ijcse/v7i2.482486}
UR - https://www.ijcseonline.org/full_paper_view.php?paper_id=3691
TI - An Efficient Approach on Big Data for Stock Prediction with the Aid of Optimal Machine Learning Approach
T2 - International Journal of Computer Sciences and Engineering
AU - T. P Sameerapallavi, B. Manjula
PY - 2019
DA - 2019/02/28
PB - IJCSE, Indore, INDIA
SP - 482-486
IS - 2
VL - 7
SN - 2347-2693
ER -
![]() |
![]() |
![]() |
303 | 196 downloads | 115 downloads |
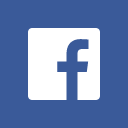
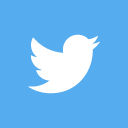
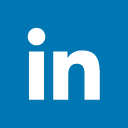
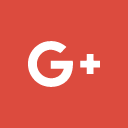
Abstract
The research on stock market is considered as an important issue from recent years. The investment in stock market is performed based on prediction and analysis. The current market economy has numerous variables which need to be considered before doing a transaction in stock market. So, the analysis of the variables manually is a tough task. In order to predict the variables in the stock market and analyse the affecting factors machine learning approach is best suited. The machine learning can provide prediction of different aspects such as index value, higher stock price, exchange rate etc. Different machine learning approaches like naive Bayes classifier, support vector machine, Artificial neural networks are reviewed which helps in stock price prediction and the market prediction. stock market prediction helps the investors and traders make better and quick decisions and ensure profits. Furthermore, advantages and limitations are discussed based on the prediction accuracy and performance.
Key-Words / Index Term
Stock market, Prediction, Big data, Machine learning
References
[1] G. V. Attigeri, M. M. Manohara Pai, M. Radhika Pai, N. Aparna, “Stock market prediction: A big data approach”. In: TENCON 2015-2015 IEEE Region 10 Conference Macao, pp. 1-4 November 2015.
[2] M. Birasnav, R. Mittal, S. Loughlin, “Linking leadership behaviours and information exchange to improve supply chain performance: A conceptual model”, Global Journal of Flexible Systems Management, Vol. 16, issue, 2, pp. 205-217, 2015.
[3] Birgul Egeli, O. Meltem, B. Bertan, “Stock market prediction using artificial neural networks”, Decision Support Systems, Vol. 22, pp.171-185, 2003.
[4] J. Bollen, H. Mao, X. Zeng, “Twitter mood predicts the stock market”, Journal of Computational Science, Vol. 2, issue, 1, pp.1–8, 2011.
[5] S. Chauhan, N. Agarwal, A.K. Kar, “Addressing big data challenges in smart cities: A systematic literature review”.
Info, Vol. 18, issue, 4, pp. 73–90, 2016.
[6] P. Grover, A.K. Kar, “Big data analytics: a review on theoretical contributions and tools used in literature” Global Journal of Flexible Systems Management, Vol. 18 issue, 3, pp. 203-229, 2017.
[7] Hell Strom, T.A. Random, “Walk through the Stock Market, Licentiate Thesis” Department of Computer Science, Umea University, Sweden, 1998.
[8] Z. Hu, J. Zhu, K. Tse, “Stocks market prediction using support vector machine”. In: 6th International Conference on Information Management, Innovation Management and Industrial Engineering, 23-24 November, 2013.
[9] Leung, C. K. S., MacKinnon, R. K., & Wang, Y. (2014). A machine learning approach for stock price prediction. In: IDEAS’14 Proceedings of the 18th International Database Engineering & Applications Symposium, ACM, July 7-9 2014, Porto, Portugal.
[10] Li, X., Xie, H., Wang, R., Cai, Y., Cao, J., Wang, F. ... & Deng, X. (2014). Empirical analysis: stock market prediction via extreme learning machine. Neural Computing and Applications, 27(1), 67-78.
[11 ]Mahajan, S. D., Deshmukh, K. V., Thite, P. R., Samel ,B. Y., & Chate, P. J.(2016). Stock Market Prediction and Analysis Using Naïve Bayes.International Journal on Recent and Innovation Trends in Computing and Communication, 4(11), 121-124.
[12] O’Connor, A. J. (2013). The power of popularity: An empirical study of the relationship between social media fan counts and brand company stock prices. Social Science Computer Review, 31(2), 229-235.
[13] Patel, J., Shah, S., Thakkar, P., & Kotecha, K. (2014). Predicting stock and stock price index movement using trend deterministic data preparation and machine learning techniques. Expert Systems with Applications, 42(1), 259-268.
[14] Sharma, A., Bhuriya, D., & Singh, U. (2017). Survey of stock market prediction using machine learning approach. In: International Conference on Electronics, Communication and Aerospace Technology, 20-22 April 2017, Indore, Madhya Pradesh.
[15] Wang, F., Zhang, Y., Xiao, H., Kaung, L., & Lai, Y. (2015). Enhancing Stock Price Prediction with a Hybrid Approach Based Extreme Learning Machine. In: International Conference on Data Mining Workshops, Atlantic City, New jersey, 14-17 November 2015.
[16] Feng wang.,Zhujong Zhao.,Xiaodong Li.,Fei yu.,Hao Zhang(2014).Stock Volatility prediction using multi-kernal learning machine. In: International Joint Conference on Neural Networks (IJCNN), july 6-11, Beijing, China, IEEE, 2014.