Significance of Spectral & Wavelet features in diagnosis of Alzheimer’s Disease
N. N. Kulkarni1 , K. R. Kasture2
Section:Research Paper, Product Type: Journal Paper
Volume-7 ,
Issue-2 , Page no. 553-559, Feb-2019
CrossRef-DOI: https://doi.org/10.26438/ijcse/v7i2.553559
Online published on Feb 28, 2019
Copyright © N. N. Kulkarni, K. R. Kasture . This is an open access article distributed under the Creative Commons Attribution License, which permits unrestricted use, distribution, and reproduction in any medium, provided the original work is properly cited.
View this paper at Google Scholar | DPI Digital Library
How to Cite this Paper
- IEEE Citation
- MLA Citation
- APA Citation
- BibTex Citation
- RIS Citation
IEEE Style Citation: N. N. Kulkarni, K. R. Kasture, “Significance of Spectral & Wavelet features in diagnosis of Alzheimer’s Disease,” International Journal of Computer Sciences and Engineering, Vol.7, Issue.2, pp.553-559, 2019.
MLA Style Citation: N. N. Kulkarni, K. R. Kasture "Significance of Spectral & Wavelet features in diagnosis of Alzheimer’s Disease." International Journal of Computer Sciences and Engineering 7.2 (2019): 553-559.
APA Style Citation: N. N. Kulkarni, K. R. Kasture, (2019). Significance of Spectral & Wavelet features in diagnosis of Alzheimer’s Disease. International Journal of Computer Sciences and Engineering, 7(2), 553-559.
BibTex Style Citation:
@article{Kulkarni_2019,
author = {N. N. Kulkarni, K. R. Kasture},
title = {Significance of Spectral & Wavelet features in diagnosis of Alzheimer’s Disease},
journal = {International Journal of Computer Sciences and Engineering},
issue_date = {2 2019},
volume = {7},
Issue = {2},
month = {2},
year = {2019},
issn = {2347-2693},
pages = {553-559},
url = {https://www.ijcseonline.org/full_paper_view.php?paper_id=3704},
doi = {https://doi.org/10.26438/ijcse/v7i2.553559}
publisher = {IJCSE, Indore, INDIA},
}
RIS Style Citation:
TY - JOUR
DO = {https://doi.org/10.26438/ijcse/v7i2.553559}
UR - https://www.ijcseonline.org/full_paper_view.php?paper_id=3704
TI - Significance of Spectral & Wavelet features in diagnosis of Alzheimer’s Disease
T2 - International Journal of Computer Sciences and Engineering
AU - N. N. Kulkarni, K. R. Kasture
PY - 2019
DA - 2019/02/28
PB - IJCSE, Indore, INDIA
SP - 553-559
IS - 2
VL - 7
SN - 2347-2693
ER -
![]() |
![]() |
![]() |
289 | 193 downloads | 143 downloads |
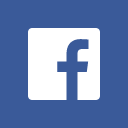
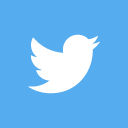
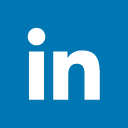
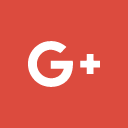
Abstract
Alzheimer Disease is one of the leading neuro-degenerative diseases. It is the most expensive disease in the modern society which is characterized by cognitive, intellectual as well as behavioral disturbance. Due to this, the early diagnosis of the disease is essential. Electroencephalography can be used as standardized tools for diagnosis of Alzheimer Disease. This paper discusses the important aspects of Electroencephalography & spectral & wavelet based features for early diagnosis of Alzheimer’s disease. This paper discusses the use of the different spectral based features such as Relative EEG power in various bands of EEG signal. In this study, it is observed that the EEG of the Alzheimer patients slows down & the EEG of the AD infected patients is less complex as that compared to the Controlled patients. In present research work, classification accuracy of 96% is achieved by use of K nearest Neighbor classifier by combination of Spectral & Wavelet based features. EEG can be therefore used as the tool for the early & automated diagnosis of Alzheimer disease.
Key-Words / Index Term
Alzheimer Disease, Dementia, EEG, Spectral features, Wavelet features, K nearesst neighbor classifier
References
[1] Mattson M., “Pathways towards and away from Alzheimer’s disease”, Nature, Vol. 430, pp. 631–639, Aug.2004.
[2] Meek P.D., McKeithan K., and Shumock G.T.,”Economics considerations of Alzheimer’s disease”, Pharmacotherapy, Vol. 18, pp.68–73, March-April 1998,
[3] World Health Organization and Alzheimer’s disease International (2012) Dementia: a public health priority, Technical report.
[4] De Carli C, Kaye JA, Horwitz B, Rapoport SI., “Critical analysis of the use of computer-assisted transverse axial tomography to study human brain in aging and dementia of the Alzheimer type”, Neurology, Vol .40 ,pp. 872–83, 1990.
[5] D. Fo¨rstl H, Kurz A., “Clinical features of Alzheimer’s disease.” Eur ArchPsychiatry Clin Neurosci, Vol.249, pp.288–90, 1999.
[6] Bianchetti A, Trabucch M., “Clinical aspects of Alzheimer’s disease” AgingClin Exp Res, Vol 13, pp. 221–230, 2001.
[7] Béatrice Duthey, A Public Health Approach to Innovation‖, Update on 2004 Background Paper written by SaloniTanna, February 2013.
[8] Kulkarni N. (2019) EEG Signal Analysis for Mild Alzheimer’s Disease Diagnosis by Means of Spectral- and Complexity-Based Features and Machine Learning Techniques. In: Kulkarni A., Satapathy S., Kang T., Kashan A. (eds) Proceedings of the 2nd International Conference on Data Engineering and Communication Technology. Advances in Intelligent Systems and Computing, vol 828. Springer, Singapore
[9] Justin Dauwels, Francois Vialatte, and Andrzej Cichocki, “Diagnosis of Alzheimer’s disease from EEG Signals: Where Are We Standing?” Current Alzheimer Research, Vol. 7 Issue 6, pp. 487-505, September 2010. .
[10] Andrzej Cichocki, Sergei L. Shishkin, ToshimitsuMusha, Zbigniew Leonowicz , Takashi Asada, Takayoshi Kurachi, “EEG filtering Based on blind source separation (BSS) for early detection of Alzheimer’s disease‖, Clinical Neurophysiology”, Vol 116, No.3, pp.729-737, March 2005.
[11] Aapo Hyvarinen, Erkki Oja, “Independent Component Analysis: Algorithms & Applications”, Journal of Neural Networks, Vol. 13, Issue.4, pp.411-430, 2000.
[12] Co Melissant, Alexander Ypma, Edward E.E. Frietman, Corn elis J.Stam, “A method for detection of Alzheimer’s disease using ICA enhanced EEG measurement”, Artificial Intelligence in Medicine, Vol. 33, No.3, pp. 209-222, March 2005.
[13] Jaeseung Jeong, “EEG dynamics in patients with Alzheimer’s disease”, Artificial Intelligence in Medicine, Vol 33, Issue 1 pp. 209-222, 2005.
[14] Simon-Shlomo Poil, WillemdeHaan, Wiesje M.vAnder Flier, HuibertD.Mansvelder Philip Scheltens and KlausLinkenkaer-Hansen, “Integrative EEG biomarkers predict progression to Alzheimer’s disease at the MCI stage”, Frontiers in Aging Neuroscience, Vol.5, Oct 2013, pp. 1-6, Article ID 58.
[15] Raymundo Cassani, Tiago H. Falk, Francisco J .Fraga, Paulo A. M. Kanda and Renato Anghinah, “The effects of automated artifact removal algorithms on electroencephalography-based Alzheimer’s disease diagnosis”, Frontiers in Aging Neuroscience, Volume 6, Article 55, pp. 1 – 13, March 2014.
[16] Kulkarni N, Bairagi V, “EEG-based diagnosis of alzheimer disease: a review and novel approaches for feature extraction and classification techniques”, Academic Press, Cambridge, 2018.
[17] Parham Ghorbanian, David Devilbiss, “Identification of Resting & Active State EEG features of Alzheimer’s Disease Using Discrete Wavelet Transform”, Annals of Biomedical Engineering, The Journal of Biomedical Engineering Society, Vol.41, No. 6, pp.1243-1257, June 2013.
[18] Andrea Rueda, Fabio A. Gonzalez, “Extracting Salient Brain Patterns for imaging based Classification of Neurodegenerative Diseases”, IEEE Trans. Med. Imaging, Vol. 33, No.6, pp. 1262-1274, June 2014.
[19] R. Viswanathan, K. Perumal, "Segmentation Technique to Detect the AD in Hippocampus Shape using Region Growing in Support Vector Machine", International Journal of Scientific Research in Computer Science and Engineering, Vol.6, Issue.1, pp.56-59, 2018.
[20] Meenu Shukla, Sanjiv Sharma, "Analysis of Efficient Classification Algorithm for Detection of Phishing Site", International Journal of Scientific Research in Computer Science and Engineering, Vol.5, Issue.3, pp.136-141, 2017