Efficient and Effective Implicit-Feedback-Based Content-Aware Collaborative Filtering For Location Recommendation
B. SaiSrilekha1 , K.S. Yuvaraj2
Section:Survey Paper, Product Type: Journal Paper
Volume-7 ,
Issue-2 , Page no. 644-648, Feb-2019
CrossRef-DOI: https://doi.org/10.26438/ijcse/v7i2.644648
Online published on Feb 28, 2019
Copyright © B. SaiSrilekha, K.S. Yuvaraj . This is an open access article distributed under the Creative Commons Attribution License, which permits unrestricted use, distribution, and reproduction in any medium, provided the original work is properly cited.
View this paper at Google Scholar | DPI Digital Library
How to Cite this Paper
- IEEE Citation
- MLA Citation
- APA Citation
- BibTex Citation
- RIS Citation
IEEE Style Citation: B. SaiSrilekha, K.S. Yuvaraj, “Efficient and Effective Implicit-Feedback-Based Content-Aware Collaborative Filtering For Location Recommendation,” International Journal of Computer Sciences and Engineering, Vol.7, Issue.2, pp.644-648, 2019.
MLA Style Citation: B. SaiSrilekha, K.S. Yuvaraj "Efficient and Effective Implicit-Feedback-Based Content-Aware Collaborative Filtering For Location Recommendation." International Journal of Computer Sciences and Engineering 7.2 (2019): 644-648.
APA Style Citation: B. SaiSrilekha, K.S. Yuvaraj, (2019). Efficient and Effective Implicit-Feedback-Based Content-Aware Collaborative Filtering For Location Recommendation. International Journal of Computer Sciences and Engineering, 7(2), 644-648.
BibTex Style Citation:
@article{SaiSrilekha_2019,
author = {B. SaiSrilekha, K.S. Yuvaraj},
title = {Efficient and Effective Implicit-Feedback-Based Content-Aware Collaborative Filtering For Location Recommendation},
journal = {International Journal of Computer Sciences and Engineering},
issue_date = {2 2019},
volume = {7},
Issue = {2},
month = {2},
year = {2019},
issn = {2347-2693},
pages = {644-648},
url = {https://www.ijcseonline.org/full_paper_view.php?paper_id=3719},
doi = {https://doi.org/10.26438/ijcse/v7i2.644648}
publisher = {IJCSE, Indore, INDIA},
}
RIS Style Citation:
TY - JOUR
DO = {https://doi.org/10.26438/ijcse/v7i2.644648}
UR - https://www.ijcseonline.org/full_paper_view.php?paper_id=3719
TI - Efficient and Effective Implicit-Feedback-Based Content-Aware Collaborative Filtering For Location Recommendation
T2 - International Journal of Computer Sciences and Engineering
AU - B. SaiSrilekha, K.S. Yuvaraj
PY - 2019
DA - 2019/02/28
PB - IJCSE, Indore, INDIA
SP - 644-648
IS - 2
VL - 7
SN - 2347-2693
ER -
![]() |
![]() |
![]() |
285 | 183 downloads | 105 downloads |
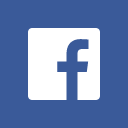
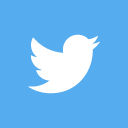
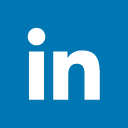
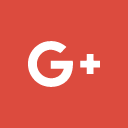
Abstract
Location recommendation assumes a basic job in helping individuals find appealing spots. In spite of the fact that ongoing examination has considered how to prescribe areas with social and topographical data, few of them tended to the chilly begin issue of new clients. Since portability records are regularly shared on interpersonal organizations, semantic data can be utilized to handle this test. A run of the mill technique is to nourish them into express input based substance mindful community oriented sifting, however they require drawing negative examples for better learning execution, as clients` negative inclination isn`t noticeable in human versatility. Be that as it may, earlier investigations have observationally appeared based strategies don`t perform well. To this end, we propose a versatile Implicit-criticism based Content-mindful Collaborative Filtering (ICCF) structure to join semantic substance and to avoid negative examining. We at that point build up a productive improvement calculation, scaling straightly with information size and highlight measure, and quadratically with the element of inert space. We further set up its association with chart Laplacian regularized framework factorization. At long last, we assess ICCF with a vast scale LBSN dataset in which clients have profiles and literary substance. The outcomes demonstrate that ICCF outflanks a few contending baselines, and that client data isn`t successful for enhancing proposals yet in addition adapting to cold-begin situations.
Key-Words / Index Term
Implicit feedback; Content-aware; Location recommendation; Weighted matrix factorization
References
[1] D. Lian, Y. Ge, F. Zhang, N. J. Yuan, X. Xie, T. Zhou, and Y. Rui, “Content-aware collaborative filtering for location recommendation based on human mobility data,” IEEE, 2015.
[2] C. R. Cloninger, T. R. Przybeck, and D. M. Svrakic, The Temperament and Character Inventory (TCI): A guide to its development and use.center for psychobiology of personality, Washington UniversitySt. Louis, MO, 1994.
[3] V. Zheng, B. Cao, Y. Zheng, X. Xie, and Q. Yang, “Collaborative filtering meets mobile recommendation: A user-centered approach,” in Proceedings of AAAI’10. AAAl Press, 2010.
[4] M. Ye, P. Yin, W.-C. Lee, and D.-L. Lee, “Exploiting geographical influence for collaborativepoint-of-interest recommendation,” in2011
[5] W.-Y. Zhu, W.-C. Peng, L.-J. Chen, K. Zheng, and X. Zhou, “Modeling user mobility for location promotion in location-based social networks,” in Proceedings of KDD’15. ACM, 2015.
[6] Maddali M.V.M. Kumar, G. Rajesh, “Cloud based Structure Approach of Content-As-A-Service for Supplier Impartial of Mobile Gadgets”, International Journal of Advanced Trends in Computer Science and Engineering, Vol. – 03, Issue – 05, pp: 282-287, 2014.
[7] H. Gao, J. Tang, X. Hu, and H. Liu, “Exploring temporal effects for location recommendation on location-based social networks,” in Proceedings of RecSys’13. ACM, 2013.
[8] Q. Yuan, G. Cong, Z. Ma, A. Sun, and N. M. Thalmann, “Timeaware point-of-interest recommendation,” in Proceedings of SIGIR’13. ACM, 2013, pp. [9] Q. Yuan, G. Cong, and A. Sun, “Graph-based point-of-interest recommendation with geographical and temporal influences,” in Proceedings of CIKM’14. 2014.
[10] A. Noulas, S. Scellato, N. Lathia, and C. Mascolo, “A random walk around the city: New venue recommendation in location-based social networks,” in Proceedings of SocialCom’12. IEEE, 2012,
[11] D. Yang, D. Zhang, Z. Yu, and Z. Wang, “A sentiment-enhanced personalized location recommendation system,” in Proceedings ofHT’13. ACM, 2013.
[12] B. Liu and H. Xiong, “Point-of-interest recommendation in location based social networks with topic and location awareness,” in Proceedings of SDM’13. SIAM, 2013.
[13] D. Lian, C. Zhao, X. Xie, G. Sun, E. Chen, and Y. Rui, “Geomf: joint geographical modeling and matrix factorization for point-of-interest recommendation,” in 2014.
[14] C. Cheng, H. Yang, I. King, and M. Lyu, “Fused matrix factorization with geographical and social influence in location-based social networks,” in 2012.
[15] Y. Liu, W. Wei, A. Sun, and C. Miao, “Exploiting geographical neighborhood characteristics for location recommendation,” in 2014.
[16] P. Lops, M. De Gemmis, and G. Semeraro, “Content-based recommender
systems: State of the art and trends,” in 2011
[17] S. Rendle, “Factorization machines Assistant Professor in the Department of
(TIST), 2012.
[18] T. Chen, W. Zhang, Q. Lu, K. Chen, Z. Zheng, and Y. Yu, “Svdfeature: a toolkit for feature-based collaborative filtering,” Journal ofMachine Learning Research 2012.
[19] D. Agarwal and B.-C. Chen, “Regression-based latent factor models,” ACM, 2009.
[21] Defu Lian, Yong Ge, Fuzheng Zhang, Nicholas Jing Yuan, Xing Xie, Tao Zhou, and Yong RuiScalable Content-Aware Collaborative Filtering for Location Recommendation,2018.