EEG Feature extraction using DaubechiesWavelet and Classification using Neural Network
Krishna Kumar N J1 , Balakrishna R2
Section:Research Paper, Product Type: Journal Paper
Volume-7 ,
Issue-2 , Page no. 792-799, Feb-2019
CrossRef-DOI: https://doi.org/10.26438/ijcse/v7i2.792799
Online published on Feb 28, 2019
Copyright © Krishna Kumar N J, Balakrishna R . This is an open access article distributed under the Creative Commons Attribution License, which permits unrestricted use, distribution, and reproduction in any medium, provided the original work is properly cited.
View this paper at Google Scholar | DPI Digital Library
How to Cite this Paper
- IEEE Citation
- MLA Citation
- APA Citation
- BibTex Citation
- RIS Citation
IEEE Style Citation: Krishna Kumar N J, Balakrishna R, “EEG Feature extraction using DaubechiesWavelet and Classification using Neural Network,” International Journal of Computer Sciences and Engineering, Vol.7, Issue.2, pp.792-799, 2019.
MLA Style Citation: Krishna Kumar N J, Balakrishna R "EEG Feature extraction using DaubechiesWavelet and Classification using Neural Network." International Journal of Computer Sciences and Engineering 7.2 (2019): 792-799.
APA Style Citation: Krishna Kumar N J, Balakrishna R, (2019). EEG Feature extraction using DaubechiesWavelet and Classification using Neural Network. International Journal of Computer Sciences and Engineering, 7(2), 792-799.
BibTex Style Citation:
@article{J_2019,
author = {Krishna Kumar N J, Balakrishna R},
title = {EEG Feature extraction using DaubechiesWavelet and Classification using Neural Network},
journal = {International Journal of Computer Sciences and Engineering},
issue_date = {2 2019},
volume = {7},
Issue = {2},
month = {2},
year = {2019},
issn = {2347-2693},
pages = {792-799},
url = {https://www.ijcseonline.org/full_paper_view.php?paper_id=3746},
doi = {https://doi.org/10.26438/ijcse/v7i2.792799}
publisher = {IJCSE, Indore, INDIA},
}
RIS Style Citation:
TY - JOUR
DO = {https://doi.org/10.26438/ijcse/v7i2.792799}
UR - https://www.ijcseonline.org/full_paper_view.php?paper_id=3746
TI - EEG Feature extraction using DaubechiesWavelet and Classification using Neural Network
T2 - International Journal of Computer Sciences and Engineering
AU - Krishna Kumar N J, Balakrishna R
PY - 2019
DA - 2019/02/28
PB - IJCSE, Indore, INDIA
SP - 792-799
IS - 2
VL - 7
SN - 2347-2693
ER -
![]() |
![]() |
![]() |
422 | 257 downloads | 167 downloads |
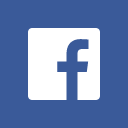
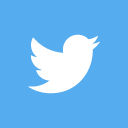
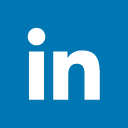
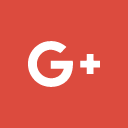
Abstract
lectroencephalography (EEG) is a straightforward technique which gives thought regarding the potential produced on the outside of the mind which helps in understanding the usefulness of the cerebrum. EEG signals play a vital job in recognizing the human feelings. In feeling appraisal using EEG flags, the time span of EEG motions in given number of channels, enthusiastic upgrades, and recurrence groups, nature of statistical feature extraction techniques and highlights important job. In this paper, new highlights are removed using Discrete Wavelet Transform (DWT) and further the feelings are arranged using EEG signs of 10 subjects is gathered and using 24 anodes from the standard 10-20 Electrode Placement System which is set over the whole scalp. Feature Extraction is performed by using DWT and the Decomposition of EEG signals is separated for 8 levels using "db4" wavelet. The feature extracted signs are then grouped using Artificial Neural Network (ANN) and the neural framework which can be compared at for feeling passionate states classification.
Key-Words / Index Term
Electroencephalogram (EEG), Discrete wavelet transform, Feature extraction, Artificial Neural Network (ANN), Daubechies Wavelet
References
[1] Murugappan M, Ramachandran N, Sazali Y. Classification of human emotion from EEG using discrete wavelet transform. Journal of Biomedical Science and Engineering. 2010 Apr 28; 3(04):390.
[2] M. A. Khalilzadeh, S. M. Homam, S. A. Hosseini and V. Niazmand,“Qualitative and Quantitative Evaluation of Brain Activity in Emotional Stress”, Iranian Journal of Neurology, vol.8 (28), pp. 605-618, 2010.
[3] K. Schaaff and T. Schultz, “Towards an EEG-Based Emotion Recognizer for Humanoid Robots”, 18th IEEE International Symposium on Robot and Human Interactive Communication, Toyama, Japan. 2009: pp. 792-796.
[4] Mingyang Li, Wanzhong Chen, Tao Zhang “Classification of epilepsy EEG signals using DWT-based envelope analysis and neural network ensemble”, Biomedical Signal Processing and Control 31 (2017), 357–365.
[5] Jasmin Kevric Abdulhamit Subasi “Comparison of signal decomposition methods in classification of EEG signals for motor-imagery BCI system”, Biomedical Signal Processing and Control 31 (2017), 398–406
[6] Gilsang Yoo, Sanghyun Seo , Sungdae Hong Hyeoncheol Kim “Emotion extraction based on multi bio-signal using back-propagation neural network” ,Springer Science , Business Media ,New York ,2016
[7] Gyanendra K. Verma, Uma Shanker Tiwary, “A Review Multimodal fusion framework: A Multiresolution approach for emotion classification and recognition from physiological signals”, Indian Institute of Information Technology Allahabad, 2014, India
[8] Suwicha Jirayucharoensak,Setha Pan-Ngum ,Pasin Israsena,“Research Article -EEG-Based Emotion Recognition Using Deep Learning Network with Principal Component Based Covariate Shift Adaptation, Hindawi Publishing Corporation Scientific World Journal ,2014.
[9] Amjed S. Al-Fahoum, Ausilah A. Al-Fraihat, “A Review Article-Methods of EEG Signal Features Extraction Using Linear Analysis in Frequency and Time-Frequency Domains”, Hindawi Publishing Corporation,ISRN Neuroscience, Volume 2014.
[10] N. Jatupaiboon, S. Pan-ngum, and P. Israsena, “Real-time EEG based happiness detection system,” Hindawi Publishing Corporation, The Scientific World Journal, vol. 2013, Article ID618649, 2013
[11] Umut Orhan , Mahmut Hekim , Mahmut Ozer, “EEG signals classification using the K-means clustering and a multilayer perceptron neural network model ” , Expert Systems with Applications 38 (2011) ,13475–13481
[12] Abdulhamit Subasi, M. Ismail Gursoy “EEG signal classification using PCA, ICA, LDA and support vector machines”, Expert Systems with Applications 37 (2010), 8659–8666
[13] Neelam Rout “Analysis and Classification Technique Based On ANN for EEG Signals, International Journal of Computer Science and Information Technologies (IJCSIT), Vol. 5 (4), 5103-5105, ISSN: 0975-9646, 2014.
[14] Guler, I., & Ubeyli, E. D. “Adaptive neuro-fuzzy inference system for classification”, Journal of Neuroscience Methods, 148, 113–121, 2005
[15] Alkan, A., Koklukaya, E., & Subasi, A., “Automatic seizure detection in EEG using logistic regression and artificial neural network “Journal of Neuroscience, Methods, 148, 167–176.2005
[16] Ubeyli, E. D. “Combined neural network model employing wavelet coefficients for EEG signals classification” Digital Signal Processing, 19, 297–308.2009a
[17] Subasi, A. “EEG signal classification using wavelet feature extraction and a mixture of expert model”, Expert Systems with Applications, 2007.
[18] Hazarika, N., Chen, J. Z., Tsoi, A. C., & Sergejew, A.“Classification of EEG signals using the wavelet transform. Signal Processing, 59(1), 61–72., 19
[19] XW Wang, D Nie, and BL Lu, ‘‘EEG-Based Emotion Recognition Using Frequency Domain Features and Support Vector Machines”, Neural Information Processing, Lecture Notes in Computer Science. Springer vol. 7062, pp. 734-743, 2011.
[20] Panagiotis C, Petrantonakis, and LeontiosHadjileontiadis,"Emotion Recognition from brain signals using Hybrid Adaptive Filtering and Higher order Crossings Analysis, “IEEE Ttransactions on affective computing, vol. 1, no. 2, pp.81 – 97,December 2010