Social Media Data Analytics Framework for Disaster Management
Sabih Ahmad Ansari1 , Ahmad Talha Siddiqui2
Section:Research Paper, Product Type: Journal Paper
Volume-7 ,
Issue-3 , Page no. 410-416, Mar-2019
CrossRef-DOI: https://doi.org/10.26438/ijcse/v7i3.410416
Online published on Mar 31, 2019
Copyright © Sabih Ahmad Ansari, Ahmad Talha Siddiqui . This is an open access article distributed under the Creative Commons Attribution License, which permits unrestricted use, distribution, and reproduction in any medium, provided the original work is properly cited.
View this paper at Google Scholar | DPI Digital Library
How to Cite this Paper
- IEEE Citation
- MLA Citation
- APA Citation
- BibTex Citation
- RIS Citation
IEEE Style Citation: Sabih Ahmad Ansari, Ahmad Talha Siddiqui, “Social Media Data Analytics Framework for Disaster Management,” International Journal of Computer Sciences and Engineering, Vol.7, Issue.3, pp.410-416, 2019.
MLA Style Citation: Sabih Ahmad Ansari, Ahmad Talha Siddiqui "Social Media Data Analytics Framework for Disaster Management." International Journal of Computer Sciences and Engineering 7.3 (2019): 410-416.
APA Style Citation: Sabih Ahmad Ansari, Ahmad Talha Siddiqui, (2019). Social Media Data Analytics Framework for Disaster Management. International Journal of Computer Sciences and Engineering, 7(3), 410-416.
BibTex Style Citation:
@article{Ansari_2019,
author = {Sabih Ahmad Ansari, Ahmad Talha Siddiqui},
title = {Social Media Data Analytics Framework for Disaster Management},
journal = {International Journal of Computer Sciences and Engineering},
issue_date = {3 2019},
volume = {7},
Issue = {3},
month = {3},
year = {2019},
issn = {2347-2693},
pages = {410-416},
url = {https://www.ijcseonline.org/full_paper_view.php?paper_id=3854},
doi = {https://doi.org/10.26438/ijcse/v7i3.410416}
publisher = {IJCSE, Indore, INDIA},
}
RIS Style Citation:
TY - JOUR
DO = {https://doi.org/10.26438/ijcse/v7i3.410416}
UR - https://www.ijcseonline.org/full_paper_view.php?paper_id=3854
TI - Social Media Data Analytics Framework for Disaster Management
T2 - International Journal of Computer Sciences and Engineering
AU - Sabih Ahmad Ansari, Ahmad Talha Siddiqui
PY - 2019
DA - 2019/03/31
PB - IJCSE, Indore, INDIA
SP - 410-416
IS - 3
VL - 7
SN - 2347-2693
ER -
![]() |
![]() |
![]() |
352 | 172 downloads | 99 downloads |
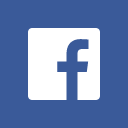
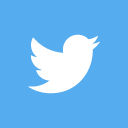
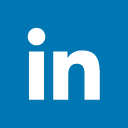
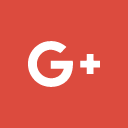
Abstract
Social media plays a significant role within the propagation of information throughout disasters. This paper essentially contains an investigation identifying with anyway people of Chennai utilized Social media especially Twitter, in light of the nation`s most exceedingly awful flood that had happened recently. The tweets are collected & analysed by various machine learning algorithms like Random Forests, Naive Bayes and call Tree. By comparison the performances of all the three, it had been found that Random Forests is that the best algorithmic rule that may be relied on, throughout a disaster. This paper conjointly targeted the sources of the Twitter messages to explore the foremost influential users of Chennai flood.
Key-Words / Index Term
Random Forests, Naive Bayes, call Tree
References
[1] B. Abedin, et al. Characterization of the use of social media in natural disasters: a systematic review. In: Big Data and Cloud Computing (BdCloud), 2014 IEEE Fourth International Conference on 2014; 449–454.
[2] L. Palen, et al. Twitter-based information distribution during the 2009 red river valley flood threat. Bulletin of the American Society for Information Science and Technology 2010; 36(5): 13–17.
[3] N.A. lateef Saeed, et al. The use of social media in knowledge integration for improving disaster emergency management task performance: Review of flood disasters. Indian Journal of Science and Technology 2016; 9(34).
[4] Y. Huang, et al. A scalable system for community discovery in twitter during hurricane sandy. In: Cluster, Cloud and Grid Computing (CCGrid), 2014 14th IEEE/ACM International Symposium on 2014; 893–899.
[5] K. Starbird, et al. Chatter on the red: what hazards
threat reveals about the social life of microblogged
information. In: Proceedings of the 2010 ACM
conference on Computer supported cooperative work
2010; 241–250
[6] K. Kandasamy, P. Koroth. An integrated approach to spam classification on twitter using url analysis, natural language processing and machine learning techniques. In: Electrical, Electronics and Computer Science (SCEECS), 2014 IEEE Students Conference on 2014; 1–5.
[7] H. Dong, et al. Social media data analytics applied to hurricane sandy. In: Social computing (SocialCom), 2013 international conference on IEEE. 2013; 963–966.
[8] S.R. Kalmegh. Comparative analysis of weka data mining algorithm randomforest, randomtree and ladtree for classification of indigenous news data. International Journal of Emerging Technology and Advanced Engineering 2015;5(1): 507–517.
[9] T.S. Aisha, et al. Exploring the use of social media during the 2014 flood in malaysia. Procedia-Social and Behavioral Sciences 2015; 211: 931–937.
[10] Nair MR, Ramya GR, Sivakumar PB (2017) Usage and analysis of Twitter during 2015 Chennai flood towards disaster management. Procedia Comput Sci 115:350–358
[11] A. Kongthon, et al. The role of twitter during a natural disaster: Case study of 2011 thai flood. In: Technology Management for Emerging Technologies (PICMET), Proceedings of PICMET12: IEEE 2012; 2227–2232.
[12] S. Vieweg, et al. Microblogging during two natural hazards events: what twitter may contribute to situational awareness. In: Proceedings of the SIGCHI conference on human factors in computing systems. ACM 2010; 1079–1088.
[13] S.J. Baek, et al. Disaster anxiety measurement and corpus-based content analysis of crisis communication. In: Systems, Man, and Cybernetics (SMC), 2013 IEEE International Conference on 2013; 1789–1794.
[14] B. Takahashi, et al. Communicating on twitter during a disaster: An analysis of tweets during typhoon haiyan in the philippines. Computers in Human Behavior 2015; 50 392–398.
[15] A. Bruns, Y.E. Liang. Tools and methods for capturing twitter data during natural disasters. First Monday 2012; 17(4).
[16] S. Srivastava. Weka: a tool for data preprocessing, classification, ensemble, clustering and association rule mining. International Journal of Computer Applications 2014; 88(10).
[17] G. Biau. Analysis of a random forests model. Journal of Machine Learning Research 2012; 1063–1095.
[18] N. Bhargava, et al. Decision tree analysis on j48 algorithm for data mining. Proceedings of International Journal of Advanced Research in Computer Science and Software Engineering 2013; 3(6).
[19] G. Ramya, P.B. Sivakumar. Advocacy monitoring of women and children health through social data. Indian Journal of Science and Technology 2016; 9(6).
[20] K.L. Devi, et al. Tweet sentiment classification using an ensemble of machine learning supervised classifiers employing statistical feature selection methods. In: Proceedings of the Fifth International Conference on Fuzzy and Neuro Computing (FANCCO-2015). Springer 2015; 1–13.
[21] T.R. Patil, S. Sherekar. Performance analysis of naive bayes and j48 classification algorithm for data classification. International Journal of Computer Science and Applications 2013; 6(2): 256–261
[22] S. Sathyamoorthy, Data Mining and Information Security in Big Data, International Journal of Scientific Research in Computer Science and Engineering Vol.5, Issue.3, pp.86-91, June (2017)