Image Segmentation for detecting Polycystic Ovarian Disease using Deep Neural Networks
Palvi Soni1 , Sheveta Vashisht2
Section:Research Paper, Product Type: Journal Paper
Volume-7 ,
Issue-3 , Page no. 534-537, Mar-2019
CrossRef-DOI: https://doi.org/10.26438/ijcse/v7i3.534537
Online published on Mar 31, 2019
Copyright © Palvi Soni, Sheveta Vashisht . This is an open access article distributed under the Creative Commons Attribution License, which permits unrestricted use, distribution, and reproduction in any medium, provided the original work is properly cited.
View this paper at Google Scholar | DPI Digital Library
How to Cite this Paper
- IEEE Citation
- MLA Citation
- APA Citation
- BibTex Citation
- RIS Citation
IEEE Style Citation: Palvi Soni, Sheveta Vashisht, “Image Segmentation for detecting Polycystic Ovarian Disease using Deep Neural Networks,” International Journal of Computer Sciences and Engineering, Vol.7, Issue.3, pp.534-537, 2019.
MLA Style Citation: Palvi Soni, Sheveta Vashisht "Image Segmentation for detecting Polycystic Ovarian Disease using Deep Neural Networks." International Journal of Computer Sciences and Engineering 7.3 (2019): 534-537.
APA Style Citation: Palvi Soni, Sheveta Vashisht, (2019). Image Segmentation for detecting Polycystic Ovarian Disease using Deep Neural Networks. International Journal of Computer Sciences and Engineering, 7(3), 534-537.
BibTex Style Citation:
@article{Soni_2019,
author = {Palvi Soni, Sheveta Vashisht},
title = {Image Segmentation for detecting Polycystic Ovarian Disease using Deep Neural Networks},
journal = {International Journal of Computer Sciences and Engineering},
issue_date = {3 2019},
volume = {7},
Issue = {3},
month = {3},
year = {2019},
issn = {2347-2693},
pages = {534-537},
url = {https://www.ijcseonline.org/full_paper_view.php?paper_id=3875},
doi = {https://doi.org/10.26438/ijcse/v7i3.534537}
publisher = {IJCSE, Indore, INDIA},
}
RIS Style Citation:
TY - JOUR
DO = {https://doi.org/10.26438/ijcse/v7i3.534537}
UR - https://www.ijcseonline.org/full_paper_view.php?paper_id=3875
TI - Image Segmentation for detecting Polycystic Ovarian Disease using Deep Neural Networks
T2 - International Journal of Computer Sciences and Engineering
AU - Palvi Soni, Sheveta Vashisht
PY - 2019
DA - 2019/03/31
PB - IJCSE, Indore, INDIA
SP - 534-537
IS - 3
VL - 7
SN - 2347-2693
ER -
![]() |
![]() |
![]() |
722 | 297 downloads | 148 downloads |
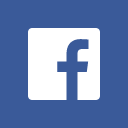
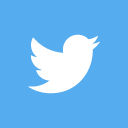
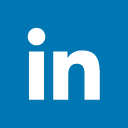
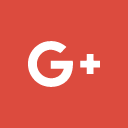
Abstract
Polycystic Ovaries in females in today’s age is a matter of concern.It can hinder the fertile nature of female and cause many more issues.Polycystic Ovaries can be detected by ultrasound.It can create a lot of problems if not taken seriously.For leading a good life females should be aware of this disease also.In approximate 70 per cent of this kind of cases remain undiagnosed. In past studies feature extraction using Convolutional Neural Network has proposed manually,here we try to propose a methodology in which we will add segmentation prior CNN so as to delete or eliminate redundant data and to achieve better accuarcy .Segmentation allows to divide the data or images so as deeply extract the exact information what is needed.
Key-Words / Index Term
Deep neural network,region growing,CNN
References
[1] Y. Deng, Y. Wang, and P. Chen, “Automated detection of Polycystic Ovary Syndrome from ultrasound images,” Conf. Proc. IEEE Eng. Med. Biol. Soc., vol. 2008, pp. 4772–4775, 2008.
[2] P. Mehrotra, B. Ghoshdastidar, and S. Ghoshdastidar, “Automated Screening of Polycystic Ovary Syndrome using Machine Learning Techniques Palak,” 2011 Annu. IEEE India Conf., 2011.
[3] R. Sitheswaran and S. Malarkhodi, “An effective automated system in follicle identification for Polycystic Ovary Syndrome using ultrasound images,” 2014 Int. Conf. Electron. Commun. Syst. ICECS 2014, 2014.
[4] C. Panchasara, “Application of Image Segmentation Techniques on Medical Reports,” vol. 6, no. 7, pp. 2931–2933, 2015.
[5] O. S. Polytechnic, “Overview of Medical Image Segmentation,” vol. 8, no. 9, pp. 13–17, 2013.
[6] P. S. Anushalin and S. I. J, “Ultrasound Image Analysis of Kidney Stone using Wavelet Transform,” vol. 1, no. August, pp. 39–49, 2014.
[7] K. Viswanath, “Design and analysis performance of Kidney Stone Detection from Ultrasound Image by Level Set Segmentation and ANN Classification,” pp. 407–414, 2014.
[8] B. Cahyono, Adiwijaya, M. S. Mubarok, and U. N. Wisesty, “An implementation of convolutional neural network on PCO classification based on ultrasound image,” 2017 5th Int. Conf. Inf. Commun. Technol. ICoIC7 2017, vol. 0, no. c, pp. 3–6, 2017.
[9] H. P. Kumar and S. Srinivasan, “Segmentation of polycystic ovary in ultrasound images,” 2nd Int. Conf. Curr. Trends Eng. Technol. ICCTET 2014, pp. 237–240, 2014.
[10] C. Science and S. Hospital, “Exploring Female Infertility Using Predictive Analytic,” 2017.
[11] E. Setiawati, Adiwijaya, and A. B. W. Tjokorda, “Particle Swarm Optimization on follicles segmentation to support PCOS detection,” 2015 3rd Int. Conf. Inf. Commun. Technol. ICoICT 2015, pp. 369–374, 2015.
[12] B. Purnama, U. N. Wisesti, Adiwijaya, F. Nhita, A. Gayatri, and T. Mutiah, “A classification of polycystic Ovary Syndrome based on follicle detection of ultrasound images,” 2015 3rd Int. Conf. Inf. Commun. Technol. ICoICT 2015, pp. 396–401, 2015.
[13]https://towardsdatascience.com/applied-deep-learning-part-4-convolutional-neural-networks-584bc134c1e2
[14]https://www.abc.net.au/news/2018-04-28/polycystic-ovary- syndrome-women-on-life-with-pcos/9607494
[15]https://www.researchgate.net/figure/Deep-CNN-architecture-to-classify-between-healthy-and-exudate-patches_fig1_318910427
[16]http://mathalytics.blogspot.com/2015/04/k-nearest-neighbor-algorithm-machine.html