Identification and Classification of Historical Kannada Handwritten Scripts based on their Age-Type using Line Segmentation with GLCM features
Parashuram Bannigidad1 , Chandrashekar Gudada2
Section:Research Paper, Product Type: Journal Paper
Volume-7 ,
Issue-3 , Page no. 754-763, Mar-2019
CrossRef-DOI: https://doi.org/10.26438/ijcse/v7i3.754763
Online published on Mar 31, 2019
Copyright © Parashuram Bannigidad, Chandrashekar Gudada . This is an open access article distributed under the Creative Commons Attribution License, which permits unrestricted use, distribution, and reproduction in any medium, provided the original work is properly cited.
View this paper at Google Scholar | DPI Digital Library
How to Cite this Paper
- IEEE Citation
- MLA Citation
- APA Citation
- BibTex Citation
- RIS Citation
IEEE Style Citation: Parashuram Bannigidad, Chandrashekar Gudada, “Identification and Classification of Historical Kannada Handwritten Scripts based on their Age-Type using Line Segmentation with GLCM features,” International Journal of Computer Sciences and Engineering, Vol.7, Issue.3, pp.754-763, 2019.
MLA Style Citation: Parashuram Bannigidad, Chandrashekar Gudada "Identification and Classification of Historical Kannada Handwritten Scripts based on their Age-Type using Line Segmentation with GLCM features." International Journal of Computer Sciences and Engineering 7.3 (2019): 754-763.
APA Style Citation: Parashuram Bannigidad, Chandrashekar Gudada, (2019). Identification and Classification of Historical Kannada Handwritten Scripts based on their Age-Type using Line Segmentation with GLCM features. International Journal of Computer Sciences and Engineering, 7(3), 754-763.
BibTex Style Citation:
@article{Bannigidad_2019,
author = {Parashuram Bannigidad, Chandrashekar Gudada},
title = {Identification and Classification of Historical Kannada Handwritten Scripts based on their Age-Type using Line Segmentation with GLCM features},
journal = {International Journal of Computer Sciences and Engineering},
issue_date = {3 2019},
volume = {7},
Issue = {3},
month = {3},
year = {2019},
issn = {2347-2693},
pages = {754-763},
url = {https://www.ijcseonline.org/full_paper_view.php?paper_id=3912},
doi = {https://doi.org/10.26438/ijcse/v7i3.754763}
publisher = {IJCSE, Indore, INDIA},
}
RIS Style Citation:
TY - JOUR
DO = {https://doi.org/10.26438/ijcse/v7i3.754763}
UR - https://www.ijcseonline.org/full_paper_view.php?paper_id=3912
TI - Identification and Classification of Historical Kannada Handwritten Scripts based on their Age-Type using Line Segmentation with GLCM features
T2 - International Journal of Computer Sciences and Engineering
AU - Parashuram Bannigidad, Chandrashekar Gudada
PY - 2019
DA - 2019/03/31
PB - IJCSE, Indore, INDIA
SP - 754-763
IS - 3
VL - 7
SN - 2347-2693
ER -
![]() |
![]() |
![]() |
509 | 186 downloads | 112 downloads |
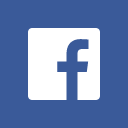
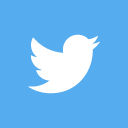
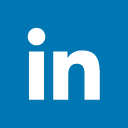
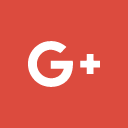
Abstract
The glorious history of the dynasties is recorded within the variety of inscriptions/epigraphic records. The department of ancient history and Archaeology is exhuming new inscriptions and need for the automatic explanation of such inscriptions is increasing, that minimizes the work or eliminates the necessity of partner epigrapher in translating antiquated epigraphs. The ancient inscription on the rock, metal plates, cloth and other writing materials are the main sources to recreate the culture and history of Karnataka in India. The offline handwritten text recognition is one of the most challenging tasks in document image analysis; our aim is to recreate the cultural importance of the Kannada Language writing tradition through the historical degraded manuscripts. In the present digital era, we need to protect and digitize the resources of our Indian culture and heritage by digitizing those manuscripts which are losing its status; the degraded manuscripts are influenced by weather condition. In this paper, we have attempted to identify and recognise the historical Kannada handwritten scripts of various dynasties; namely, Vijayanagara dynasty (1460 AD), Mysore Wodeyar dynasty (1936 AD), Vijayanagara dynasty (1400 AD) and Hoysala dynasty (1340 AD) by using the improved seam carving text line segmentation method with GLCM features. The average classification accuracy for different dynasties is computed. The LDA classifier has yielded 86.5%, K-NN classifier has yielded 85.3% and SVM classifier has 85.6%. Based on the experimentation, the LDA classifier has recorded good classification performance comparatively K-NN and SVM classifiers for historical Kannada handwritten scripts.
Key-Words / Index Term
Restoration, Seam carving, Line segmentation, Kannada, LDA, K-NN, SVM, Recognition, GLCM, handwritten script, historical documents, document image analysis
References
[1] Manjunath, M.G., Devarajaswamy G.K., “Kannada Lipiya Vikasa”, Published by Jagadhguru Sri Madhvacharya Trust, Sri Raghavendra Swami Matta, Mantralaya
[2] Narasimha Murthy, A.V., “Kannada Lipiya Ugama Mattu Vikasa”, Kannada Adhyayana Samsthe, Mysore University, Mysore (1968)
[3] Reddy D., “Lipiya HuttuMattu Belavanige—Origin and Evolution of Script”, Kannada Pustaka Pradhikara (Kannada Book Authority), Bangalore
[4] Nikolaos Arvanitopoulos and Sabine Susstrunk, “Seam Carving for Text Line Extraction on Color and Grayscale Historical Manuscripts” 14th IEEE International Conference on Frontiers in Handwriting Recognition, DOI: 10.1109/ICFHR.2014.127, pp.726-731, 2014.
[5] S. Avidan, A. Shamir, “Seam Carving for Content-Aware Image Resizing”, ACM Transactions on Graphics, vol. 26, no. 3, p. 10, 2007.
[6] Veronica Romero, Joan Andreu Sanchez, Vicente Bosch, Katrien Depuydt and Jesse de Does, “Influence of Text Line Segmentation in Handwritten Text Recognition” 13th IEEE International Conference on Document Analysis and Recognition (ICDAR), 978-1-4799-1805-8/15, pp.536-540, 2015.
[7] Maroua Mehri, Petra Gomez-Kramer, Pierre Héroux, Alain Boucher and Rémy Mullot,“Texture feature evaluation for segmentation of historical document images”, 2nd International Workshop on Historical Document Imaging and Processing, 2013, Washington, United States,pp.102-109, 2013.
[8] Mehri M., Héroux P., Gomez-Krämer and Remy Mullot, “Texture feature benchmarking and evaluation for historical document image analysis” IJDAR 20: 1. DOI: 10.1007/s10032-016-0278-y, pp. 1-35, 2017.
[9] Darko Brodić, “Script Recognition by Statistical Analysis of the Image Texture”, X International Symposium on Industrial Electronics INDEL 2014, Banja Luka, November 06 to 08, 2014, pp.168-174.
[10] Berat Kurar Barakat and Jihad El-Sana, “Binarization Free Layout Analysis for Arabic Historical Documents Using Fully Convolutional Networks”, IEEE 2nd International Workshop on Arabic and Derived Script Analysis and Recognition, {ASAR}, London, UK, March 12-14, 2018,pp.151-155, 2018.
[11] Laurence Likforman-Sulem, Abderrazak Zahour and Bruno Taconet, “Text line segmentation of historical documents: a survey” IJDAR (2007) pp:123–138, DOI 10.1007/s10032-006-0023-z
[12] Abedelkadir Asi, Raid Saabni and Jihad El-Sana, “Text Line Segmentation for Gray Scale Historical Document Images”, HIP `11 Proceedings of the 2011 Workshop on Historical Document Imaging and Processing, DOI: 10.1145/2037342.2037362, pp. 120-126, 2011.
[13] Parashuram Bannigidad and Chandrashekar Gudada, “Restoration of Degraded Historical Kannada Handwritten Document Images using Image Enhancement Techniques”, International Conference on Soft Computing and Pattern Recognition (SoCPaR 2016), 2016. pp. 498-508.
[14] Parashuram Bannigidad and Chandrashekar Gudada, “Restoration of Degraded Kannada Handwritten Paper Inscriptions (Hastaprati) using Image Enhancement Techniques”, IEEE International Conference on Computer Communication and Informatics (ICCCI -2017), 2017, pp.1-6.
[15] Parashuram Bannigidad and Chandrashekar Gudada, “Identification and Recognition of Historical Kannada Handwritten Document Images Using GLCM Features”, International Journal of Advanced Research in Computer Science, Vol.9, No.1, 2018, pp.686-690.
[16] Robert M Haralick, K Shanmugam and Its`hak Dinstein, “Textural Features for Image Classification”, IEEE Transactions on Systems, Man, and Cybernetics. SMC-3 (6), 1973, pp. 610–621.