A Survey on Feature Extraction Methods in Retinal Fundus Images for Diabetic Retinopathy
Noushira K I1 , Anil Kumar K.R2 , Meenakshy K3
Section:Survey Paper, Product Type: Journal Paper
Volume-7 ,
Issue-4 , Page no. 77-87, Apr-2019
CrossRef-DOI: https://doi.org/10.26438/ijcse/v7i4.7787
Online published on Apr 30, 2019
Copyright © Noushira K I, Anil Kumar K.R, Meenakshy K . This is an open access article distributed under the Creative Commons Attribution License, which permits unrestricted use, distribution, and reproduction in any medium, provided the original work is properly cited.
View this paper at Google Scholar | DPI Digital Library
How to Cite this Paper
- IEEE Citation
- MLA Citation
- APA Citation
- BibTex Citation
- RIS Citation
IEEE Citation
IEEE Style Citation: Noushira K I, Anil Kumar K.R, Meenakshy K, “A Survey on Feature Extraction Methods in Retinal Fundus Images for Diabetic Retinopathy,” International Journal of Computer Sciences and Engineering, Vol.7, Issue.4, pp.77-87, 2019.
MLA Citation
MLA Style Citation: Noushira K I, Anil Kumar K.R, Meenakshy K "A Survey on Feature Extraction Methods in Retinal Fundus Images for Diabetic Retinopathy." International Journal of Computer Sciences and Engineering 7.4 (2019): 77-87.
APA Citation
APA Style Citation: Noushira K I, Anil Kumar K.R, Meenakshy K, (2019). A Survey on Feature Extraction Methods in Retinal Fundus Images for Diabetic Retinopathy. International Journal of Computer Sciences and Engineering, 7(4), 77-87.
BibTex Citation
BibTex Style Citation:
@article{I_2019,
author = {Noushira K I, Anil Kumar K.R, Meenakshy K},
title = {A Survey on Feature Extraction Methods in Retinal Fundus Images for Diabetic Retinopathy},
journal = {International Journal of Computer Sciences and Engineering},
issue_date = {4 2019},
volume = {7},
Issue = {4},
month = {4},
year = {2019},
issn = {2347-2693},
pages = {77-87},
url = {https://www.ijcseonline.org/full_paper_view.php?paper_id=3998},
doi = {https://doi.org/10.26438/ijcse/v7i4.7787}
publisher = {IJCSE, Indore, INDIA},
}
RIS Citation
RIS Style Citation:
TY - JOUR
DO = {https://doi.org/10.26438/ijcse/v7i4.7787}
UR - https://www.ijcseonline.org/full_paper_view.php?paper_id=3998
TI - A Survey on Feature Extraction Methods in Retinal Fundus Images for Diabetic Retinopathy
T2 - International Journal of Computer Sciences and Engineering
AU - Noushira K I, Anil Kumar K.R, Meenakshy K
PY - 2019
DA - 2019/04/30
PB - IJCSE, Indore, INDIA
SP - 77-87
IS - 4
VL - 7
SN - 2347-2693
ER -
![]() |
![]() |
![]() |
963 | 550 downloads | 291 downloads |
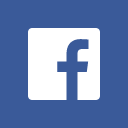
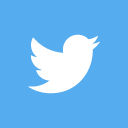
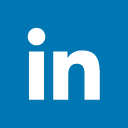
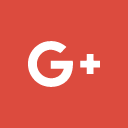
Abstract
The uniqueness of retinal microvasculature is that it is the only part of human circulation that can be directly visualised non-invasively in vivo and readily photographed. Developments in fundus image processing over the past 20 years includes advancement being made towards developing automated detection for conditions, such as diabetic retinopathy, age-related macular degeneration and retinopathy of prematurity. Features of retinal blood vessels, microaneurysms, exudates and the hemorrhages are extracted to detect the Diabetic Retinopathy (DR) in the early stages. Diabetic Retinopathy results fluid leaks from retinal blood vessels leading to vision loss. Microaneurysms appear as small circular dark spots on the surface of the retina. The appearance of red and yellow lesions on retina is exudates and hemorrhages. Image processing algorithms can be used to reduce the workload of ophthalmologist and play a vital role in quality assurance tasks. Feature extraction is the first step in developing these automated algorithms for detecting retinal pathologies. Here we review numerous early studies that used for automatic detection of these features. Most of the literature has differences in the method used to evaluate their algorithms or the dataset used, which makes it difficult to compare any two algorithms together. Our study reveals that even though a large number of feature extraction technique are available there is still scope for more accurate algorithms which will work with High Resolution Fundus (HRF) images also.
Key-Words / Index Term
Diabetic retinopathy, Exudates, Hemorrhages, Micro aneurysms
References
[1] O. Faust, R. Acharya, E. Y.-K. Ng, K.-H. Ng, and J. S. Suri, “Algorithms for the automated detection of diabetic retinopathy using digital fundus images: a review”, Journal of medical systems, vol. 36, no. 1, pp. 145–157, 2012.
[2] T. Spencer, J . A .Olson, K. C. McHardy, P. F. Sharp, and J. V. Forrester, “An image processing strategy for the segmentation and quantification of microaneurysms in fluorescein angiograms of the ocular fundus,” Computers and biomedical research, vol. 29, no. 4, pp. 284–302, 1996.
[3] M. J. Cree, J. A. Olson, K. C. McHardy, P. F.Sharp, and J. V. Forrester, “A fully automated comparative microaneurysm digital detection system,” Eye, vol. 11, no. 5, p. 622, 1997.
[4] A. Sopharak, B. Uyyanonvara, and S. Barman, “Simple hybrid method for fine microaneurysm detection from non-dilated diabetic retinopathy retinal images,” Computerized Medical Imaging and Graphics, vol. 37, no. 5-6, pp. 394–402, 2013.
[5] M. U. Akram, S. Khalid, and S. A. Khan, “Identification and classification of microaneurysms for early detection of diabetic retinopathy,” Pattern Recognition, vol. 46, no.1, pp. 107–116,2013.
[6] M.Niemeijer, B. Van Ginneken, J. Staal, M. S. A. Suttorp-Schulten, and M. D. Abramoff, “Automatic detection of red lesions in digital color fundus photographs,” IEEE Transactions on Medical Imaging, vol. 24, no. 5, pp. 584–592, May 2005.
[7] A. Mizutani, C. Muramatsu, Y. Hatanaka, S. Suemori, T. Hara, and H. Fujita, “Automated microaneurysm detection method based on double ring filter in retinal fundus images,” in Medical Imaging 2009: Computer-Aided Diagnosis, vol. 7260. International Society for Optics and Photonics, 2009, p. 72601N.
[8] G. Quellec, M. Lamard, P. M. Josselin, G. Cazuguel, B. Cochener, and C. Roux, “Optimal wavelet transform for the detectionof microaneurysms in retina photographs.” IEEE Transactions on Medical Imaging, vol. 27, no. 9, pp. 1230–41, 2008.
[9] B. Zhang, X. Wu, J. You, Q. Li, and F. Karray, “Detection of microaneurysms using multiscale correlation coefficients,” Pattern Recognition, vol. 43, no. 6, pp. 2237–2248, 2010.
[10] C. Sinthanayothin, J. F. Boyce, T. H. Williamson, H. L. Cook, E. Mensah, S. Lal, and D. Usher, “Automated detection of diabetic retinopathy on digital fundus images,” Diabetic medicine, vol. 19, no. 2, pp. 105–112, 2002.
[11] A. D. Fleming, S. Philip, K. A. Goatman, J. A.Olson, and P. F. Sharp, “Automated microaneurysm detection using local contrast normalization and local vessel detection,” IEEE transactions on medical imaging, vol. 25, no. 9, pp.1223–1232, 2006.
[12] M. Habib, R. Welikala, A. Hoppe, C. Owen, A.Rudnicka, and S. Barman, “Detection of microaneurysms in retinal images using an ensemble classifier,” Informatics in Medicine Unlocked, vol. 9, pp. 44–57, 2017.
[13] M. P. Bala and S. Vijayachitra, “Early detection and classification of microaneurysms in retinal fundus images using sequential learning methods,” International Journal of Biomedical Engineering and Technology, vol. 15, no. 2, pp. 128– 143, 2014.
[14] I. Lazar and A. Hajdu, “Microaneurysm detection in retinal images using a rotating cross section based model,” in Biomedical Imaging: From Nano to Macro, 2011 IEEE International Symposium on. IEEE, 2011, pp. 1405–1409.
[15] P. Subbuthai and S. Muruganand, “Roc curve analysis for automatic detection of microaneurysms in the retinal fundus image,” International Journal of ChemTech Research, vol. 10, no. 13, pp. 190–194, 2017.
[16] U. R. Acharya, C. M. Lim, E. Y. K. Ng, C. Chee, and T. Tamura, “Computer-based detection of diabetes retinopathy stages using digital fundus images,” Proceedings of the institution of mechanical engineers, part H: journal of engineering in medicine, vol. 223, no. 5, pp. 545–553, 2009.
[17] H. Wang, W. Hsu, K. G. Goh, and M. L. Lee,“An effective approach to detect lesions in colour retinal images,” in Computer Vision and Pattern Recognition, 2000. Proceedings. IEEE Conference on, vol. 2. IEEE, 2000, pp. 181–186.
[18] D. Kavitha and S. S. Devi, “Automatic detection of optic disc and exudates in retinal images,” in Intelligent Sensing and Information Processing, 2005. Proceedings of 2005 International Conference on. IEEE, 2005, pp. 501–506.
[19] M. Garcia, C. I. S´anchez, J. Poza, M. I. L´opez, and R. Hornero, “Detection of hard exudates in retinal images using a radial basis function classifier,” Annals of biomedical engineering, vol. 37, no. 7, pp. 1448–1463, 2009.
[20] S. Sreng, J.-i Takada, N. Maneerat, D. Isarakorn, R. Varakulsiripunth, B. Pasaya, and M R. Panjaphongse, “Feature extraction from retinal fundus image for early detection of diabetic retinopathy,” in Humanitarian Technology Conference (R10-HTC), 2013 IEEE Region 10.IEEE, 2013, pp. 63–66.
[21] K. Y. Win and S. Choomchuay, “Automated detection of exudates using histogram analysis for digital retinal images,” in Intelligent Signal Processing and Communication Systems (ISPACS),2016 International Symposium on. IEEE, 2016, pp. 1–6.
[22] D. T. Susetianingtias and S. Madenda, “An approach to exudates detection using color reference segmentation in retinal fundus image,” International Journal of Computer Applications, vol. 146, no. 2, 2016.
[23] C. Sinthanayothin, V. Kongbunkiat, S. Phoo jaruenchanachai, and A. Singalavanija, “Automated screening system for diabetic retinopathy,” in Image and Signal Processing and Analysis, 2003. ISPA 2003. Proceedings of the 3rd International Symposium on, vol. 2.IEEE, 2003,pp. 915–920.
[24] H. Li and O. Chutatape, “Automated feature extraction in colour retinal images by a model based approach,” IEEE Transactions on biomedical engineering, vol. 51, no.2,pp.246–254,2004.
[25] S. Joshi and P. Karule, “A review on exudates detection methods for diabetic retinopathy,” Biomedicine & Pharmacotherapy, vol. 97, pp. 1454–1460, 2018.
[26] J. C. Dunn, “A fuzzy relative of the isodata process and its use in detecting compact well separated clusters,” 1973.
[27] J. C. Bezdek, J. Keller, R. Krisnapuram, and N. Pal, Fuzzy models and algorithms for pattern recognition and image processing. Springer Science & Business Media, 1999, vol. 4.
[28] A.Osareh, M. Mirmehdi, B. Thomas, and R. Markham, “Automated identification of diabetic retinal exudates in digital colour images,” British Journal of Ophthalmology, vol. 87,no. 10, pp. 1220–1223, 2003.
[29] A. Osareh, B. Shadgar, and R. Markham, “A computational-intelligence-based approach for detection of exudates in diabetic retinopathy images,” IEEE Transactions on Information Technology in Biomedicine, vol. 13, no. 4, pp. 535–545, 2009.
[30] P. H. Princye and V. Vijayakumari, “Detection of exudates and feature extraction of retinal images using fuzzy clustering method,” Computational Intelligence and Information Technology, 2013.
[31] T. Walter, J.-C. Klein, P. Massin, and A. Erginay, “A contribution of image processing to the diagnosis of diabetic retinopathy detection of exudates in color fundus images of the human retina,” IEEE transactions on medical imaging, vol. 21, no. 10, pp. 1236–1243, 2002.
[32] A. Sopharak, B. Uyyanonvara, S. Barman, and T. H. Williamson, “Automatic detection of diabetic retinopathy exudates from non dilated retinal images using mathematical morphology methods,” Computerized medical imaging and graphics, vol. 32, no. 8, pp. 720–727, 2008.
[33] D. Welfer, J. Scharcanski, and D. R. Marinho, “A morphologic two-stage approach for automated optic disk detection in color eye fundus images,” Pattern Recognition Letters, vol. 34, no. 5, pp. 476–485, 2013.
[34] G. Gardner, D. Keating, T. H. Williamson, and A. T. Elliott, “Automatic detection of diabetic retinopathy using an artificial neural network: a screening tool.” British journal of Ophthalmology, vol. 80, no. 11, pp. 940–944, 1996.
[35] S. K. Mitra, T.-W. Lee, and M. Goldbaum, “A Bayesian network based sequential inference for diagnosis of diseases from retinal images,” Pattern recognition letters, vol. 26, no. 4, pp. 459–470, 2005.
[36] A. Osareh, M. Mirmehdi, B. Thomas, and R. Markham, “Comparative exudate classification using support vector machines and neural networks,” in International Conference on Medical Image Computing and Computer-Assisted Intervention. Springer, 2002, pp. 413–420.
[37] A. Hunter, J. Lowell, J. Owens, L. Kennedy, and D. Steele, “Quantification of diabetic retinopathy using neural networks and sensitivity analysis,” in Artificial Neural Networks in Medicine and Biology. Springer, 2000, pp. 81–86.
[38] L. Xu and S. Luo, “Support vector machine based method for identifying hard exudates in retinal images,” in Information, Computing and Telecommunication, 2009. YC-ICT’09.IEEE Youth Conference on. IEEE, 2009, pp. 138–141.
[39] V. V. Kumari and N. SuriyaNarayanan, “Diabetic retinopathy-early detection using image processing techniques,” International Journal on Computer Science and Engineering, vol. 2, no. 02, pp. 357–361, 2010.
[40] S. Roychowdhury, D. D. Koozekanani, and K. K.Parhi, “Dream: diabetic retinopathy analysis using machine learning,” IEEE journal of biomedical and health informatics, vol. 18, no. 5, pp. 1717–1728, 2014.
[41] M. Niemeijer, B. van Ginneken, S. R. Russell, M. S. Suttorp-Schulten, and M. D. Abramoff, “Automated detection and differentiation of drusen, exudates, and cotton-wool spots in digital colour fundus photographs for diabetic retinopathy diagnosis,” Investigative ophthalmology & visual science, vol. 48, no. 5, pp. 2260–2267, 2007.
[42] K. Adem, “Exudate detection for diabetic retinopathy with circular hough transformation and convolutional neural networks,” Expert Systems with Applications, vol. 114, pp.289–295, 2018.
[43] R. Phillips, J. Forrester, and P. Sharp, “Automated detection and quantification of retinal exudates,” Graefe’s archive for clinical and experimental ophthalmology, vol. 231, no. 2, pp. 90–94,1993.
[44] E. B, H. O, L. O, M. K, J. B, K. D, and C. DA, “Screening for diabetic retinopathy using computer based image analysis and statistical classification.”Comput.Meth. Programs Biomed. vol. 62, p. 165, 2000.
[45] X. Zhang and O. Chutatape, “Top-down and bottom-up strategies in lesion detection of background diabetic retinopathy,” in Computer Vision and Pattern Recognition, 2005. CVPR 2005. IEEE Computer Society Conference on, vol. 2. IEEE, 2005, pp. 422–428.
[46] M. Larsen, J. Godt, N. Larsen, H Lund Andersen, A. K. Sjolie, E. Agardh, H. Kalm, M. Grunkin, and D. R. Owens, “Automated detection of fundus photographic red lesions in diabetic retinopathy,” Investigative ophthalmology & visual science, vol. 44, no. 2, pp. 761–766, 2003.
[47] M. Niemeijer, B. Van Ginneken, J. Staal, M. S. Suttorp-Schulten, and M. D. Abramoff, “Automatic detection of red lesions in digital colour fundus photographs,” IEEE Transactions on medical imaging, vol. 24, no. 5, pp. 584–592, 2005.