Network Intrusion Detection Using Genitic Algorithm: A Comparision
Sayi Sruthi.k1 , Liston Deva Glinds2 , Saran Raj3
Section:Research Paper, Product Type: Journal Paper
Volume-7 ,
Issue-4 , Page no. 134-136, Apr-2019
CrossRef-DOI: https://doi.org/10.26438/ijcse/v7i4.134136
Online published on Apr 30, 2019
Copyright © Sayi Sruthi.k, Liston Deva Glinds, Saran Raj . This is an open access article distributed under the Creative Commons Attribution License, which permits unrestricted use, distribution, and reproduction in any medium, provided the original work is properly cited.
View this paper at Google Scholar | DPI Digital Library
How to Cite this Paper
- IEEE Citation
- MLA Citation
- APA Citation
- BibTex Citation
- RIS Citation
IEEE Style Citation: Sayi Sruthi.k, Liston Deva Glinds, Saran Raj, “Network Intrusion Detection Using Genitic Algorithm: A Comparision,” International Journal of Computer Sciences and Engineering, Vol.7, Issue.4, pp.134-136, 2019.
MLA Style Citation: Sayi Sruthi.k, Liston Deva Glinds, Saran Raj "Network Intrusion Detection Using Genitic Algorithm: A Comparision." International Journal of Computer Sciences and Engineering 7.4 (2019): 134-136.
APA Style Citation: Sayi Sruthi.k, Liston Deva Glinds, Saran Raj, (2019). Network Intrusion Detection Using Genitic Algorithm: A Comparision. International Journal of Computer Sciences and Engineering, 7(4), 134-136.
BibTex Style Citation:
@article{Sruthi.k_2019,
author = {Sayi Sruthi.k, Liston Deva Glinds, Saran Raj},
title = {Network Intrusion Detection Using Genitic Algorithm: A Comparision},
journal = {International Journal of Computer Sciences and Engineering},
issue_date = {4 2019},
volume = {7},
Issue = {4},
month = {4},
year = {2019},
issn = {2347-2693},
pages = {134-136},
url = {https://www.ijcseonline.org/full_paper_view.php?paper_id=4007},
doi = {https://doi.org/10.26438/ijcse/v7i4.134136}
publisher = {IJCSE, Indore, INDIA},
}
RIS Style Citation:
TY - JOUR
DO = {https://doi.org/10.26438/ijcse/v7i4.134136}
UR - https://www.ijcseonline.org/full_paper_view.php?paper_id=4007
TI - Network Intrusion Detection Using Genitic Algorithm: A Comparision
T2 - International Journal of Computer Sciences and Engineering
AU - Sayi Sruthi.k, Liston Deva Glinds, Saran Raj
PY - 2019
DA - 2019/04/30
PB - IJCSE, Indore, INDIA
SP - 134-136
IS - 4
VL - 7
SN - 2347-2693
ER -
![]() |
![]() |
![]() |
336 | 274 downloads | 175 downloads |
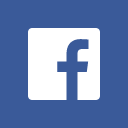
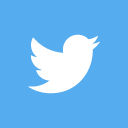
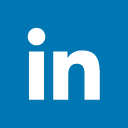
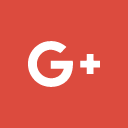
Abstract
The network intrusion detection system is used to detect and analyze the network traffic and all possible network threats that may affect the system. When the threats are identified the network intrusion detection system immediately takes action such as alerting the administrator or blocking the source of ip address from accessing the network. Various research activities are already conducted to find a efficient and effective solution to prevent intrusions in the network in order to ensure the network security and privacy .machine learning is the one of the efficient and effective techniques to detect network intrusion. Due to high traffic flow, the traditional signature based intrusion detection system is inefficient one to detect anomalies the machine learning techniques is the solution for this. In this paper a combination of two machine learning algorithm is proposed to classify any anomalous behavior in the network traffic. The overall efficiency of the proposed method is dignified recall. However using area under the Receiver operating curve (ROC) metric, we find that genetic algorithm is the best among the two algorithm proposed in this work.
Key-Words / Index Term
Intrusion detection,Genetic Algorithm, Rbf algorithm, Roc metrics calculation
References
[1] Syarif I, Prugel Bennett A, Wills G., “Unsupervised clustering approach for network anomaly detection”, Networked Digital Technologies Communications in Computer and Information Science, vol. 293. Berlin Heidelberg: Springer, 2012, pp.135–45.
[2] S. Novakov, C.-H. Lung, I. Lambadaris, Ioannis N. Seddigh, “Studies in applying PCA and wavelet algorithms for network traffic anomaly detection”, Proc. of IEEE 14th International Conference on High Performance Switching and Routing, 2013, pp. 185-190.
[3]Intrusion Detection using an Ensemble of Classification Methods, M.Govindarajan and RM.Chandrasekaran, Proceedings of the World Congress on Engineering and Computer Science 2012 Vol I WCECS 2012, October 24-26, 2012, San Francisco, USA
[4] S. Novakov, C.-H. Lung, I. Lambadaris, Ioannis N. Seddigh, “Combining statistical and spectral analysis techniques in network traffic anomaly detection”, Proc. of IEEE Conf. on Next Generation Networks and Services, 2012, pp. 94-101.
[5] S.A. Mulay, P. R. Devale, G.V. Garje, “Intrusion Detection System using Support Vector Machine and Decision Tree”, International Journal of Computer Applications, vol. 3, no. 3, 2010.
[6] J. Cannady, “Artificial neural networks for misuse detection,” in Proceedings of the 1998 National Information Systems Secu- rity Conference, pp. 443–456, Arlington, VA, USA, 1998.
[7] S. Pan, T. Morris, and U. Adhikari, “Developing a hybrid intrusion detection system using data mining for power sys- tems,” IEEE Transactions on Smart Grid, vol. 6, no. 6, pp. 3104–3113, 2015.