“Review Paper on Crops Disease Diagnosing Using Image-Based Deep Learning Mechanism”
Pragya Lariya1 , Mukul Shrivastava2
Section:Review Paper, Product Type: Journal Paper
Volume-7 ,
Issue-4 , Page no. 306-312, Apr-2019
CrossRef-DOI: https://doi.org/10.26438/ijcse/v7i4.306312
Online published on Apr 30, 2019
Copyright © Pragya Lariya, Mukul Shrivastava . This is an open access article distributed under the Creative Commons Attribution License, which permits unrestricted use, distribution, and reproduction in any medium, provided the original work is properly cited.
View this paper at Google Scholar | DPI Digital Library
How to Cite this Paper
- IEEE Citation
- MLA Citation
- APA Citation
- BibTex Citation
- RIS Citation
IEEE Style Citation: Pragya Lariya, Mukul Shrivastava, ““Review Paper on Crops Disease Diagnosing Using Image-Based Deep Learning Mechanism”,” International Journal of Computer Sciences and Engineering, Vol.7, Issue.4, pp.306-312, 2019.
MLA Style Citation: Pragya Lariya, Mukul Shrivastava "“Review Paper on Crops Disease Diagnosing Using Image-Based Deep Learning Mechanism”." International Journal of Computer Sciences and Engineering 7.4 (2019): 306-312.
APA Style Citation: Pragya Lariya, Mukul Shrivastava, (2019). “Review Paper on Crops Disease Diagnosing Using Image-Based Deep Learning Mechanism”. International Journal of Computer Sciences and Engineering, 7(4), 306-312.
BibTex Style Citation:
@article{Lariya_2019,
author = {Pragya Lariya, Mukul Shrivastava},
title = {“Review Paper on Crops Disease Diagnosing Using Image-Based Deep Learning Mechanism”},
journal = {International Journal of Computer Sciences and Engineering},
issue_date = {4 2019},
volume = {7},
Issue = {4},
month = {4},
year = {2019},
issn = {2347-2693},
pages = {306-312},
url = {https://www.ijcseonline.org/full_paper_view.php?paper_id=4033},
doi = {https://doi.org/10.26438/ijcse/v7i4.306312}
publisher = {IJCSE, Indore, INDIA},
}
RIS Style Citation:
TY - JOUR
DO = {https://doi.org/10.26438/ijcse/v7i4.306312}
UR - https://www.ijcseonline.org/full_paper_view.php?paper_id=4033
TI - “Review Paper on Crops Disease Diagnosing Using Image-Based Deep Learning Mechanism”
T2 - International Journal of Computer Sciences and Engineering
AU - Pragya Lariya, Mukul Shrivastava
PY - 2019
DA - 2019/04/30
PB - IJCSE, Indore, INDIA
SP - 306-312
IS - 4
VL - 7
SN - 2347-2693
ER -
![]() |
![]() |
![]() |
472 | 323 downloads | 163 downloads |
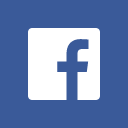
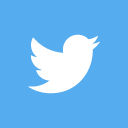
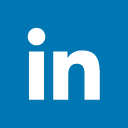
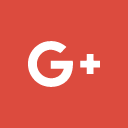
Abstract
Agricultural productivity are some things on that economy extremely depends. This can be the one among the explanations that disease detection in plants plays a crucial role in agriculture field, as having diseases in plants are quite natural. If correct care isn`t taken during this space then it causes serious effects on plants and because of that several product quality, amount or productivity is affected. Crop diseases function a significant threat to food provide. As a results of the growing of smartphone technology throughout the globe, it`s currently become technical possible to leverage image process techniques to identity variety of disease from a straightforward photograph. Employing a public dataset of 54,306 pictures of pathological and healthy plant leaves collected beneath controlled conditions, we have a tendency to train a deep convolutional neural network to spot fourteen crop species and twenty six diseases.This paper discuss about the crop diseases diagnosing using Deep Learning which becomes the foremost correct and precise paradigms for the detection of disease. Leaves of Infected crops are collected and labelled in line with the illness, process of image is performed together with pixel-wise operations to boost the image info. It`s followed with feature extraction, segmentation and therefore the classification of patterns of captured leaves so as to spot plant leaf diseases. Four classifier labels are used as microorganism Spot, Yellow Leaf Curl Virus, blight and Healthy Leaf. The options extracted are match into the neural network with twenty epochs. Many artificial neural network architectures are enforced with the most effective performance of ninety 98.59% accuracy in determinative the disease. This was a good success, demonstrating the feasibleness of this approach within the field of disease diagnosis and high crop yielding.
Key-Words / Index Term
Deep Learning, Image-based Crops, CNN diagnosing
References
[1]. Machine-Learning: What it is and why it matters, 09 2016, [online] Available: www.sas.com.
[2]. R. E. SCHAPIRE, "The boosting approach to machine learning: An overview" in Nonlinear estimation and classification, New York: Springer, pp. 149-171, 2003.
[3]. A. Ben-HUR, D. Horn, H. T. Siegel MANN, V. VAPNIK, "Support vector clustering", Journal of machine learning research, vol. 2, pp. 125-137, Dec 2001.
[4]. J. R. OTUKEI, T. BLASCHKE, "Land cover change assessment using decision trees support vector machines and maximum likelihood classification algorithms", International Journal of Applied Earth Observation and Geo information, vol. 12, pp. S27-S31, 2010.
[5]. PANIGRAHI, S. & Ting K.C. (1998) Artificial Intelligence for Biology and Agriculture, Kluwer Academic Press).
[6]. MUKHOPADHYAY S.C. (2012) Smart Sensing Technology for Agriculture and Environmental Monitoring. Vol. 146, Springer Berlin Heidelberg.
[7]. German, L., RAMISCH, J.J. & VERMA R. (2010) Beyond the Biophysical, Knowledge, Culture, and Power in Agriculture and Natural Resource Management, Springer Publ.
[8]. Won Don Lee (2009). The Development and Application of Decision Tree for Agriculture Data. IITSI :16-20.
[9]. LEEMANS, V., DESTAIN, M.F.,2004.A real-time grading method of apples based on features extracted from defects. J. Food Eng. 61, 83-89.
[10]. Quinlan, J.R.(1985b). Decision trees and multi-valued attributes. In J.E. Hayes & D. MICHIE (Eds.), Machine intelligence 11. Oxford University Press (in press).
[11]. ZELU Zia (2009). An Expert System Based on Spatial Data Mining used Decision Tree for Agriculture Land Grading. Second International Conference on Intelligent Computation.
[12]. K. Muthukannan1, P. Latha2, R. Pon Selvi1 and P. Nisha1 “CLASSIFICATION OF DISEASED PLANT LEAVES USING NEURAL NETWORK ALGORITHMS”, VOL. 10, NO. 4,
[13] Hughes D, Marcel Salathe (2015) An open access repository of images on plant health to enable the development of mobile disease diagnostics pp 1–13, 1511.08060.
[14] Blancard D (2012) 2 - Diagnosis of Parasitic and Nonparasitic Diseases. Academic Press.
[15] Chen XW, Lin X (2014) Big data deep learning: Challenges and perspectives. IEEE Access 2:514–525, DOI 10.1109/ACCESS.2014.2325029.
[16] Dandawate Y, Kokare R (2015) An automated approach for classification of plant diseases towards development of futuristic Decision Support System in Indian perspective. In: 2015 International Conference on Advances in Computing, Communications and Informatics, ICACCI 2015, IEEE, Kochi,India, pp 794–799.
[17] Fujita E, Kawasaki Y, Uga H, Kagiwada S, Iyatomi H (2016) Basic investigation on a robust and practical plant diagnostic system. In: Proceedings - 2016 15th IEEE International Conference on Machine Learning and Applications, ICMLA 2016, pp 989–992.
[18] Szegedy C et al. (2015) Going deeper with convolutions in Proceedings of the IEEE Conference on Computer Vision and Pattern Recognition. pp. 1–9.
[19] Bay H, Ess A, Tuytelaars T, Van Gool L (2008) Speeded-up robust features (surf). Computer vision and image understanding 110(3):346–359.
[20] LeCun Y et al. (1989) Backpropagation applied to handwritten zip code recognition. Neural computation 1(4):541–551.