Face Recognition: Modern Assessment of Features Extraction
Payal P. Parekh1 , M. M. Goyani2
Section:Survey Paper, Product Type: Journal Paper
Volume-7 ,
Issue-4 , Page no. 330-335, Apr-2019
CrossRef-DOI: https://doi.org/10.26438/ijcse/v7i4.330335
Online published on Apr 30, 2019
Copyright © Payal P. Parekh, M. M. Goyani . This is an open access article distributed under the Creative Commons Attribution License, which permits unrestricted use, distribution, and reproduction in any medium, provided the original work is properly cited.
View this paper at Google Scholar | DPI Digital Library
How to Cite this Paper
- IEEE Citation
- MLA Citation
- APA Citation
- BibTex Citation
- RIS Citation
IEEE Style Citation: Payal P. Parekh, M. M. Goyani, “Face Recognition: Modern Assessment of Features Extraction,” International Journal of Computer Sciences and Engineering, Vol.7, Issue.4, pp.330-335, 2019.
MLA Style Citation: Payal P. Parekh, M. M. Goyani "Face Recognition: Modern Assessment of Features Extraction." International Journal of Computer Sciences and Engineering 7.4 (2019): 330-335.
APA Style Citation: Payal P. Parekh, M. M. Goyani, (2019). Face Recognition: Modern Assessment of Features Extraction. International Journal of Computer Sciences and Engineering, 7(4), 330-335.
BibTex Style Citation:
@article{Parekh_2019,
author = {Payal P. Parekh, M. M. Goyani},
title = {Face Recognition: Modern Assessment of Features Extraction},
journal = {International Journal of Computer Sciences and Engineering},
issue_date = {4 2019},
volume = {7},
Issue = {4},
month = {4},
year = {2019},
issn = {2347-2693},
pages = {330-335},
url = {https://www.ijcseonline.org/full_paper_view.php?paper_id=4037},
doi = {https://doi.org/10.26438/ijcse/v7i4.330335}
publisher = {IJCSE, Indore, INDIA},
}
RIS Style Citation:
TY - JOUR
DO = {https://doi.org/10.26438/ijcse/v7i4.330335}
UR - https://www.ijcseonline.org/full_paper_view.php?paper_id=4037
TI - Face Recognition: Modern Assessment of Features Extraction
T2 - International Journal of Computer Sciences and Engineering
AU - Payal P. Parekh, M. M. Goyani
PY - 2019
DA - 2019/04/30
PB - IJCSE, Indore, INDIA
SP - 330-335
IS - 4
VL - 7
SN - 2347-2693
ER -
![]() |
![]() |
![]() |
328 | 246 downloads | 160 downloads |
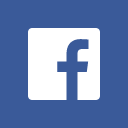
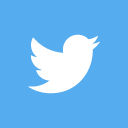
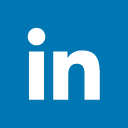
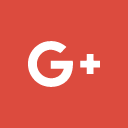
Abstract
Face recognition is the capability of identifying and authenticating the dominating and leading features of the face from the dataset images. It`s important in Access and Security, Healthcare, Banking, Criminal Identification, Payment, Advertising, and in many other fields. In this paper, we have assessment important basic phases of face recognition like Pre-processing, Face Detection, Feature Extraction, Optimal Feature Selection, and Classification. Feature Extraction, Feature Selection, and Classification play a major role in face recognition. The research area of statistical texture classification is widely investigated in several computer vision and pattern recognition problems. A general framework for face recognition with statistical and geometrical approaches and classification presented in this survey paper.
Key-Words / Index Term
Face Recognition, Feature Extraction Approaches, Optimal Feature Reduction, Classification
References
[1] Brunelli, R., & Poggio, T. (1993). Face Recognition: Features versus Templates. IEEE Transactions on Pattern Analysis and Machine Intelligence, 15(10).
[2] Zhao, W., Krishnaswamy, A., Chellappa, R., Swets, D. L., & Weng, J. (1998). Discriminant analysis of principal components for face recognition. In Face Recognition (pp. 73-85). Springer, Berlin, Heidelberg
[3] Ahonen, T., Hadid, A., & Pietikäinen, M. (2004). Face recognition with local binary patterns. In European conference on computer vision (pp. 469-481). Springer, Berlin, Heidelberg.
[4] Tan, X., & Triggs, B. (2010). Recognition Under Difficult Lighting Conditions, In IEEE Transactions on Image Processing, 19(6), 1635–1650.
[5] Juefei-Xu, F., Luu, K., Savvides, M., Bui, T. D., & Suen, C. Y. (2011). Investigating age invariant face recognition based on periocular biometrics. In 2011 International Joint Conference on Biometrics (IJCB) (pp. 1-7). IEEE.
[6] Xie, Z., Jiang, P., & Zhang, S. (2017). Fusion of LBP and HOG using multiple kernel learning for infrared face recognition. In 2017 IEEE/ACIS 16th International Conference on Computer and Information Science (ICIS) (pp. 81-84). IEEE. https://doi.org/10.1109/ICIS.2017.7959973
[7] Yin, X., & Member, X. L. (2017). Multi-Task Convolutional Neural Network for Pose-Invariant Face Recognition. IEEE Transactions on Image Processing, 7149(c), 1–12. https://doi.org/10.1109/TIP.2017.2765830
[8] Ayyavoo, T., & John Suseela, J. (2018). Illumination pre-processing method for face recognition using 2D DWT and CLAHE. IET Biometrics, 7(4), 380–390. https://doi.org/10.1049/iet-bmt.2016.0092
[9] Osuna, E., & Freund, R. (1997). Training Support Vector Machines: an Application to Face Detection, In Computer Vision and Pattern Recognition130–136.
[10] Sung, K., & Poggio, T. (1998). Example-Based Learning for View-Based Human Face Detection. IEEE TRANSACTIONS ON PATTERN ANALYSIS AND MACHINE INTELLIGENCE, 20(1), 39–51.
[11] Yang, M. H., Roth, D., & Ahuja, N. (2000). A SNoW-based face detector. In Advances in neural information processing systems (pp. 862-868).
[12] Viola, P., & Jones, M. (2004). Robust Real-Time Face Detection. International Journal of Computer Vision, 57(2), 137–154.
[13] Weng, R., Lu, J., & Tan, Y. P. (2016). Robust Point Set Matching for Partial Face Recognition. IEEE Transactions on Image Processing, 25(3), 1163–1176. https://doi.org/10.1109/TIP.2016.2515987
[14] Yang, F., Yang, W., Gao, R., & Liao, Q. (2018). Discriminative Multidimensional Scaling for Low-Resolution Face Recognition. IEEE Signal Processing Letters, 25(3), 388–392. https://doi.org/10.1109/LSP.2017.2746658
[15] Ojala, T. (1996). A COMPARATIVE STUDY OF TEXTURE MEASURES WITH CLASSIFICATION BASED ON. Pattern Recognition, 29(1), 51–59.
[16] Guo, Y., Zhao, G., & Pietik, M. (2012). Discriminative features for texture description. Pattern Recognition, 45, 3834–3843. https://doi.org/10.1016/j.patcog.2012.04.003
[17] Rao, L. K., Rohini, P., & Reddy, L. P. (2018). Local color oppugnant quantized extrema patterns for image retrieval. Multidimensional Systems and Signal Processing, 1-23.
[18] Li, L., Feng, L., Wu, J., Sun, M. X., & Liu, S. L. (2018). Exploiting global and local features for image retrieval. Journal of Central South University, 25(2), 259-276.
[19] Wang, J., Fan, Y., Li, Z., & Lei, T. (2018). Texture classification using multi-resolution global and local Gabor features in pyramid space. Signal, Image and Video Processing. https://doi.org/10.1007/s11760-018-1341-6
[20] Fanaee, F., Yazdi, M., & Faghihi, M. (2019). Face image super-resolution via sparse representation and wavelet transform. Signal, Image and Video Processing, 13(1), 79-86. https://doi.org/10.1007/s11760-018-1330-9.
[21] Ayyavoo, T., & Suseela, J. J. (2017). Illumination pre-processing method for face recognition using 2D DWT and CLAHE. IET Biometrics, 7(4), 380-390.
[22] Zhou, D., Yang, D., & Zhang, X. (2017). Exploring joint encoding of multi-direction local binary patterns for image classification. Multimedia Tools and Applications, 1-25.
[23] Cui, X., Zhou, P., & Yang, W. (2017). Local dominant orientation feature histograms (LDOFH) for face recognition. In Applied Informatics Vol. 4, No. 1, p. 14.
[24] Weng, R., Lu, J., & Tan, Y. P. (2016). Robust point set matching for partial face recognition. IEEE transactions on image processing, 25(3), 1163-1176.