Performance Evaluation of MOA v/s KNN Classification Schemes: Case Study of Major Cities in the World
Sanjay Srivas1 , P. G. Khot2
Section:Research Paper, Product Type: Journal Paper
Volume-7 ,
Issue-4 , Page no. 489-495, Apr-2019
CrossRef-DOI: https://doi.org/10.26438/ijcse/v7i4.489495
Online published on Apr 30, 2019
Copyright © Sanjay Srivas, P. G. Khot . This is an open access article distributed under the Creative Commons Attribution License, which permits unrestricted use, distribution, and reproduction in any medium, provided the original work is properly cited.
View this paper at Google Scholar | DPI Digital Library
How to Cite this Paper
- IEEE Citation
- MLA Citation
- APA Citation
- BibTex Citation
- RIS Citation
IEEE Style Citation: Sanjay Srivas, P. G. Khot, “Performance Evaluation of MOA v/s KNN Classification Schemes: Case Study of Major Cities in the World,” International Journal of Computer Sciences and Engineering, Vol.7, Issue.4, pp.489-495, 2019.
MLA Style Citation: Sanjay Srivas, P. G. Khot "Performance Evaluation of MOA v/s KNN Classification Schemes: Case Study of Major Cities in the World." International Journal of Computer Sciences and Engineering 7.4 (2019): 489-495.
APA Style Citation: Sanjay Srivas, P. G. Khot, (2019). Performance Evaluation of MOA v/s KNN Classification Schemes: Case Study of Major Cities in the World. International Journal of Computer Sciences and Engineering, 7(4), 489-495.
BibTex Style Citation:
@article{Srivas_2019,
author = {Sanjay Srivas, P. G. Khot},
title = {Performance Evaluation of MOA v/s KNN Classification Schemes: Case Study of Major Cities in the World},
journal = {International Journal of Computer Sciences and Engineering},
issue_date = {4 2019},
volume = {7},
Issue = {4},
month = {4},
year = {2019},
issn = {2347-2693},
pages = {489-495},
url = {https://www.ijcseonline.org/full_paper_view.php?paper_id=4063},
doi = {https://doi.org/10.26438/ijcse/v7i4.489495}
publisher = {IJCSE, Indore, INDIA},
}
RIS Style Citation:
TY - JOUR
DO = {https://doi.org/10.26438/ijcse/v7i4.489495}
UR - https://www.ijcseonline.org/full_paper_view.php?paper_id=4063
TI - Performance Evaluation of MOA v/s KNN Classification Schemes: Case Study of Major Cities in the World
T2 - International Journal of Computer Sciences and Engineering
AU - Sanjay Srivas, P. G. Khot
PY - 2019
DA - 2019/04/30
PB - IJCSE, Indore, INDIA
SP - 489-495
IS - 4
VL - 7
SN - 2347-2693
ER -
![]() |
![]() |
![]() |
267 | 191 downloads | 185 downloads |
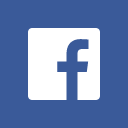
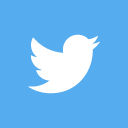
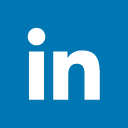
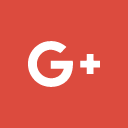
Abstract
Satellite imageries are widely available from various sources which can be used for Land use/Land cover analysis. Land use/Land cover analysis is necessary for environmental monitoring, urban planning and natural resource analysis. In this paper, we have used newly created algorithm- Multi Objective Algorithm (MOA) which is the combination of two metaheuristic algorithms for classification of satellite imageries. Classification result was compared with the KNN (K-Nearest Neighbour) algorithm. In this view, satellite imageries of Delhi and Shenyang were used for the experiment purpose. Also accuracy of classification was measured using the error matrix/kappa coefficient and was compared with the KNN classification technique. The classification results of the two major cities indicate a substantial difference in the percentage of overall accuracy and kappa coefficient value.
Key-Words / Index Term
Classification, Land use/Land Cover, K-Nearest Neighbour, MOA, Accuracy Assessment
References
[1] Congalton, R. G. (1991). A review of assessing the accuracy of classifications of remotely sensed data. Remote Sensing of Environment, 37(1), 35–46.
[2] Sanjay Srivas, P. G. Khot, (2018). Analysis & Visualization of Multidimensional GIS Images Using Multi Objective Algorithm (MOA). International Journal of Computer Sciences and Engineering, 6(8), 460-464.
[3] Darius Phiri, & Justin Morgenroth. (2017). Developments in Landsat Land Cover Classification Methods: A Review. Remote Sensing, 9(9), 967.
[4] Liu, X. Supervised Classification and Unsupervised Classification. 12.
[5] Kalra, K., Goswami, A. K., & Gupta, R. (2013). A Comparative Study of Supervised Image Classification Algorithms for Satellite Images. International Journal of Electrical, Electronics and Data Communication, 1(10), 7.
[6] Canty, M. J., & Nielsen, A. A. (2006). Visualization and unsupervised classification of changes in multispectral satellite imagery. International Journal of Remote Sensing, 27(18), 3961–3975.
[7] Cai, Y., Ji, D., & Cai, D. (2010). A KNN Research Paper Classification Method Based on Shared Nearest Neighbor. NTCIR, 5.
[8] Ahmad, A., & Quegan, S. (2012). Analysis of Maximum Likelihood Classification on Multispectral Data. Applied Mathematical Sciences, 6(129), 6425 - 6436.
[9] Yadav, J., & Sharma, M. (2013). A Review of K-mean Algorithm. International Journal of Engineering Trends and Technology, 4(7), 5.
[10] Scofield, G. B., Pantaleao, E., & Negri, R. G. (2015). A Comparison of Accuracy Measures for Remote Sensing Image Classification: Case Study In An Amazonian Region Using Support Vector Machine, International Journal Image Processing, 9(1), 11–21.
[11] Rwanga, S. S., & Ndambuki, J. M. (2017). Accuracy Assessment of Land Use/Land Cover Classification Using Remote Sensing and GIS. International Journal of Geosciences, 08(04), 611–622.
[12] Viera, A. J., & Garrett, J. M. (2005). Understanding Interobserver Agreement: The Kappa Statistic. Family Medicine, 37(5), 360-363.
[13] Olmsted, A. Calculating kappa measures of agreement and standard errors using SAS software: some tricks and traps. 6
[14] Bharatkar, P. S., & Patel, R. (2013). Approach to Accuracy Assessment tor RS Image Classification Techniques. International Journal of Scientific & Engineering Research, 4(12), 8.