Consistent Product Moisture Prediction in Manufacturing using Time Series Analysis
V.P. Gladis Pushparathi1 , D. Robin Reni2 , M. Selva Kumar3 , V. Prasanna Kumar4 , M. Suriyakiran5
Section:Research Paper, Product Type: Journal Paper
Volume-7 ,
Issue-4 , Page no. 507-510, Apr-2019
CrossRef-DOI: https://doi.org/10.26438/ijcse/v7i4.507510
Online published on Apr 30, 2019
Copyright © V.P. Gladis Pushparathi, D. Robin Reni, M. Selva Kumar, V. Prasanna Kumar, M. Suriyakiran . This is an open access article distributed under the Creative Commons Attribution License, which permits unrestricted use, distribution, and reproduction in any medium, provided the original work is properly cited.
View this paper at Google Scholar | DPI Digital Library
How to Cite this Paper
- IEEE Citation
- MLA Citation
- APA Citation
- BibTex Citation
- RIS Citation
IEEE Style Citation: V.P. Gladis Pushparathi, D. Robin Reni, M. Selva Kumar, V. Prasanna Kumar, M. Suriyakiran, “Consistent Product Moisture Prediction in Manufacturing using Time Series Analysis,” International Journal of Computer Sciences and Engineering, Vol.7, Issue.4, pp.507-510, 2019.
MLA Style Citation: V.P. Gladis Pushparathi, D. Robin Reni, M. Selva Kumar, V. Prasanna Kumar, M. Suriyakiran "Consistent Product Moisture Prediction in Manufacturing using Time Series Analysis." International Journal of Computer Sciences and Engineering 7.4 (2019): 507-510.
APA Style Citation: V.P. Gladis Pushparathi, D. Robin Reni, M. Selva Kumar, V. Prasanna Kumar, M. Suriyakiran, (2019). Consistent Product Moisture Prediction in Manufacturing using Time Series Analysis. International Journal of Computer Sciences and Engineering, 7(4), 507-510.
BibTex Style Citation:
@article{Pushparathi_2019,
author = {V.P. Gladis Pushparathi, D. Robin Reni, M. Selva Kumar, V. Prasanna Kumar, M. Suriyakiran},
title = {Consistent Product Moisture Prediction in Manufacturing using Time Series Analysis},
journal = {International Journal of Computer Sciences and Engineering},
issue_date = {4 2019},
volume = {7},
Issue = {4},
month = {4},
year = {2019},
issn = {2347-2693},
pages = {507-510},
url = {https://www.ijcseonline.org/full_paper_view.php?paper_id=4066},
doi = {https://doi.org/10.26438/ijcse/v7i4.507510}
publisher = {IJCSE, Indore, INDIA},
}
RIS Style Citation:
TY - JOUR
DO = {https://doi.org/10.26438/ijcse/v7i4.507510}
UR - https://www.ijcseonline.org/full_paper_view.php?paper_id=4066
TI - Consistent Product Moisture Prediction in Manufacturing using Time Series Analysis
T2 - International Journal of Computer Sciences and Engineering
AU - V.P. Gladis Pushparathi, D. Robin Reni, M. Selva Kumar, V. Prasanna Kumar, M. Suriyakiran
PY - 2019
DA - 2019/04/30
PB - IJCSE, Indore, INDIA
SP - 507-510
IS - 4
VL - 7
SN - 2347-2693
ER -
![]() |
![]() |
![]() |
499 | 342 downloads | 135 downloads |
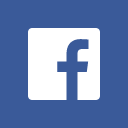
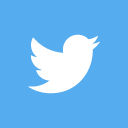
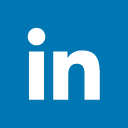
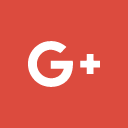
Abstract
Maintaining a consistent moisture environment for a product is one of the key objectives in a manufacturing process. Mostly the product moisture is maintained by a temperature sensor which is manually controlled by a human who knows knowledge about the system and also supervising the temperature by human does not assure complete moisture control of the system. To address this problem, a time series model trained with a custom product moisture dataset which can predict the temperature to be maintained with 97% accuracy in the storage system has been implemented in the temperature system. In this time series model, Longest Short Memory Network is used as neural network architecture with some defined hyper parameters to achieve target accuracy.
Key-Words / Index Term
Time Series Model, LSTM, Moisture Control
References
[1] Poonnoy P “.Artificial Neural Network Modeling for Temperature and Moisture Content Prediction in Tomato Slices Undergoing Microwave” - J Food Sci,2007
[2] Martinez - Martine .V baladron. C,Gomez , Ruiz Navas-Gracia LM, Aguiar JM, Carro B, “Temperature and relative humidity estimation and prediction in the tobacco drying process using Artificial Neural Networks” Department of Signal Theory, Communications and Telematics Engineering, University of Valladolid, 47011 Valladolid, Spain.
[3] Herve Debar, Monique Becker, Didier Siboni, "A Neural Network Component for an Intrusion Detection System" in , Les Ulis Cedex France, 1992.
[4] ason Brownlee, “On the Suitability of Long Short-Term Memory Networks for Time Series Forecasting J”, May 26, 2017
[5] S. Hochreiter and J. Schmidhuber, “Long short-term memory,” Neural Computation, vol. 9, no. 8, pp. 1735–1780, 1997.
[6] L. Wei, E. Keogh, "Semi-supervised time series classification", Proc. 12th ACM SIGKDD Int. Conf. Knowl. Discovery Data Mining, pp. 748-753, 2006.
[7] Huarng ,Tiffany Hui kuang Yu ,Yu Wei Hsu, “A Multivariate Heuristic Model for Fuzzy Time-Series Forecasting” 2007
[8] A.B. Mahagaonkar, A.R. Buchade, “Application Layer Denial of Service Attack Detection using Deep Learning Approach”, vol 7, pp 44-48, 2019
[9] Dipankar Das, “Application of Multilayer Perceptron for Forecasting of Selected IIPs of India – An Empirical Analysis” vol 6, pp 400-406, 2018.
[10] D.K. Shetty, B. Ismail, “Forecasting Financial Time Series using a Hybrid Non-stationary Model with ANN”, vol 7, pp 323-326 2019
[11] Felix A Gers, Nicol N Schraudolph, Jürgen Schmid-huber, "Learning precise timing with LSTM recurrent networks", The Journal of Machine Learning Research, vol. 3, pp. 115-143, 2003.
[12] Xavier Glorot, Antoine Bordes, Yoshua Bengio, "Deep Sparse Rectifier Neural Networks", Aistats, vol. 15, no. 106, pp. 275, 2011.
[13] Sep Hochreiter,Jürgen Schmidhuber,"Long Short-term memory", Neural computation, vol. 9, no. 8, pp. 1735-1780, 1997.
[14] IS Isa et al., "Suitable MLP network activation functions for breast cancer and thyroid disease detection", 2010 Second International Conference on Computational Intelligence Modelling and Simulation, pp. 39-44, 2010.
[15] P. Anitha, D. Rajesh, K. Venkata Ratnam, “Machine Learning in Intrusion Detection – A Survey” vol 7, pp 112-119, 2019