Movie Recommendation System
Kunal Raj1 , Atulya Abhinav Das2 , Antariksh Guha3 , Parth Sharma4 , Mohana Kumar S5
Section:Survey Paper, Product Type: Journal Paper
Volume-7 ,
Issue-4 , Page no. 1024-1028, Apr-2019
CrossRef-DOI: https://doi.org/10.26438/ijcse/v7i4.10241028
Online published on Apr 30, 2019
Copyright © Kunal Raj, Atulya Abhinav Das, Antariksh Guha, Parth Sharma, Mohana Kumar S . This is an open access article distributed under the Creative Commons Attribution License, which permits unrestricted use, distribution, and reproduction in any medium, provided the original work is properly cited.
View this paper at Google Scholar | DPI Digital Library
How to Cite this Paper
- IEEE Citation
- MLA Citation
- APA Citation
- BibTex Citation
- RIS Citation
IEEE Style Citation: Kunal Raj, Atulya Abhinav Das, Antariksh Guha, Parth Sharma, Mohana Kumar S, “Movie Recommendation System,” International Journal of Computer Sciences and Engineering, Vol.7, Issue.4, pp.1024-1028, 2019.
MLA Style Citation: Kunal Raj, Atulya Abhinav Das, Antariksh Guha, Parth Sharma, Mohana Kumar S "Movie Recommendation System." International Journal of Computer Sciences and Engineering 7.4 (2019): 1024-1028.
APA Style Citation: Kunal Raj, Atulya Abhinav Das, Antariksh Guha, Parth Sharma, Mohana Kumar S, (2019). Movie Recommendation System. International Journal of Computer Sciences and Engineering, 7(4), 1024-1028.
BibTex Style Citation:
@article{Raj_2019,
author = {Kunal Raj, Atulya Abhinav Das, Antariksh Guha, Parth Sharma, Mohana Kumar S},
title = {Movie Recommendation System},
journal = {International Journal of Computer Sciences and Engineering},
issue_date = {4 2019},
volume = {7},
Issue = {4},
month = {4},
year = {2019},
issn = {2347-2693},
pages = {1024-1028},
url = {https://www.ijcseonline.org/full_paper_view.php?paper_id=4160},
doi = {https://doi.org/10.26438/ijcse/v7i4.10241028}
publisher = {IJCSE, Indore, INDIA},
}
RIS Style Citation:
TY - JOUR
DO = {https://doi.org/10.26438/ijcse/v7i4.10241028}
UR - https://www.ijcseonline.org/full_paper_view.php?paper_id=4160
TI - Movie Recommendation System
T2 - International Journal of Computer Sciences and Engineering
AU - Kunal Raj, Atulya Abhinav Das, Antariksh Guha, Parth Sharma, Mohana Kumar S
PY - 2019
DA - 2019/04/30
PB - IJCSE, Indore, INDIA
SP - 1024-1028
IS - 4
VL - 7
SN - 2347-2693
ER -
![]() |
![]() |
![]() |
663 | 479 downloads | 153 downloads |
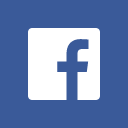
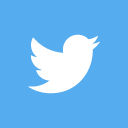
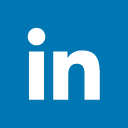
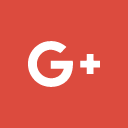
Abstract
In this hustling world, enjoyment is a need for every one of us to refresh our temper and energy. Entertainment regains our self-assurance for work and we can work extra enthusiastically. For revitalizing ourselves, we are able to pay attention to our preferred music or can watch films of our preference. For looking favorable movies online, we will make use of movie recommendation systems, that are extra dependable, when you consider that searching of preferred films would require more and more time which one can ‘t have the funds to waste. In this paper, to improve the quality of a movie recommendation system, a deep learning-based approach is presented to find out what exactly was being talked about in the user`s review and the sentiments that people are expressing.
Key-Words / Index Term
Deep Learning , Recommendation System, Review Sentiment Analysis
References
[1] S. M. Taheri and I. Irajian, "DeepMovRS : A unified framework for deep learning-based movie recommender Systems," 2018 6th Iranian Joint Congress on Fuzzy and Intelligent Systems (CFIS), Kerman, 2018, pp. 200-204. DOI: 10.1109/CFIS.2018.8336633
[2] H.-T. Cheng, L. Koc, J. Harmsen, T. Shaked, T. Chandra, H.Aradhye, G.Anderson, G. Corrado, W. Chai, M. Ispir, R Anil, Z. Haque, L. Hong,V. Jain, X. Liu and H. Shah, Wide & Deep Learning for Recommender Systems, In Proceedings of the 1st Workshop on Deep Learning for Recommender Systems (DLRS 2016), (2016). ACM, New York, NY, USA, 7-10.
[3] P. Covington, J. Adams and E. Sargin, Deep Neural Networks for YouTube Recommendations, In Proceedings of the 10th ACM Conference on Recommender Systems, (2016). ACM, New York, NY, USA, 191-198.
[4] X. He, L. Liao, H. Zhang, L. Nie, X. Hu and T.-S. Chua, Neural Collaborative Filtering, In Proceedings of the 26th International Conference on World Wide Web (WWW ’17), (2017). International World Wide Web Conferences Steering Committee, Republic and Canton of Geneva, Switzerland, 173-182.
[5] Y. Koren, R. Bell and C. Volinsky, Matrix Factorization Techniques for Recommender Systems, Computer, 42, 8 (August 2009), 30-37.
[6]Tomas Mikolov, Ilya Sutskever, Kai Chen, Greg Corrado, Jeffrey Dean and G. Hinton, Distributed Representations of Words and Phrases and their Compositionality,NIPS`13 Proceedings of the 26th International Conference on Neural Information Processing Systems - Volume 2.
[7] H. Wang, N. Wang and D.-Y. Yeung, Collaborative Deep Learning for Recommender Systems, In Proceedings of the 21th ACM SIGKDD International Conference on Knowledge Discovery and Data Mining (KDD ’15), (2015). ACM, New York, NY, USA, 1235-1244.
[8] F. Zhang, N. J. Yuan, D. Lian, X. Xie and W.-Y. Ma, Collaborative Knowledge Base Embedding for Recommender Systems, In Proceedings of the 22nd ACM SIGKDD International Conference on Knowledge Discovery and Data Mining (KDD ’16), (2016). ACM, New York, NY, USA, 353-362.