Classification of Customer to Upgrade Profits in Retail Market with Deep Learning Methodology
I.Gayathri Devi1 , G. Surya Kala Eswari2 , G. Kumari3
Section:Research Paper, Product Type: Journal Paper
Volume-7 ,
Issue-5 , Page no. 188-191, May-2019
CrossRef-DOI: https://doi.org/10.26438/ijcse/v7i5.188191
Online published on May 31, 2019
Copyright © I.Gayathri Devi, G. Surya Kala Eswari, G. Kumari . This is an open access article distributed under the Creative Commons Attribution License, which permits unrestricted use, distribution, and reproduction in any medium, provided the original work is properly cited.
View this paper at Google Scholar | DPI Digital Library
How to Cite this Paper
- IEEE Citation
- MLA Citation
- APA Citation
- BibTex Citation
- RIS Citation
IEEE Style Citation: I.Gayathri Devi, G. Surya Kala Eswari, G. Kumari, “Classification of Customer to Upgrade Profits in Retail Market with Deep Learning Methodology,” International Journal of Computer Sciences and Engineering, Vol.7, Issue.5, pp.188-191, 2019.
MLA Style Citation: I.Gayathri Devi, G. Surya Kala Eswari, G. Kumari "Classification of Customer to Upgrade Profits in Retail Market with Deep Learning Methodology." International Journal of Computer Sciences and Engineering 7.5 (2019): 188-191.
APA Style Citation: I.Gayathri Devi, G. Surya Kala Eswari, G. Kumari, (2019). Classification of Customer to Upgrade Profits in Retail Market with Deep Learning Methodology. International Journal of Computer Sciences and Engineering, 7(5), 188-191.
BibTex Style Citation:
@article{Devi_2019,
author = {I.Gayathri Devi, G. Surya Kala Eswari, G. Kumari},
title = {Classification of Customer to Upgrade Profits in Retail Market with Deep Learning Methodology},
journal = {International Journal of Computer Sciences and Engineering},
issue_date = {5 2019},
volume = {7},
Issue = {5},
month = {5},
year = {2019},
issn = {2347-2693},
pages = {188-191},
url = {https://www.ijcseonline.org/full_paper_view.php?paper_id=4220},
doi = {https://doi.org/10.26438/ijcse/v7i5.188191}
publisher = {IJCSE, Indore, INDIA},
}
RIS Style Citation:
TY - JOUR
DO = {https://doi.org/10.26438/ijcse/v7i5.188191}
UR - https://www.ijcseonline.org/full_paper_view.php?paper_id=4220
TI - Classification of Customer to Upgrade Profits in Retail Market with Deep Learning Methodology
T2 - International Journal of Computer Sciences and Engineering
AU - I.Gayathri Devi, G. Surya Kala Eswari, G. Kumari
PY - 2019
DA - 2019/05/31
PB - IJCSE, Indore, INDIA
SP - 188-191
IS - 5
VL - 7
SN - 2347-2693
ER -
![]() |
![]() |
![]() |
399 | 329 downloads | 181 downloads |
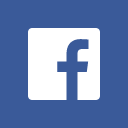
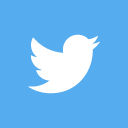
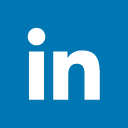
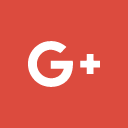
Abstract
Capital investment in retail sector and competition in the market has changed the style of marketing. At the same time the enhancements in the field of information technology provided an upper hand to the marketer to know the exact need, preference and perches trend of the customer. By knowing the actual need, preference and purchase trend of customers the marketer can make a future business plan to increase the sale and earn more profit. This paper provides a framework to the retail marketer to find the potential customer by analyzing the previous purchase history of the customer. This task can be accomplished by the use of data mining technique. In this paper we have used k-mean clustering algorithm and Naive Bayes’ classifier for in identifying potential customer for a particular section of products of the retailer.
Key-Words / Index Term
Naive Bayes,Cluster, Centroid,Foreign Direct Investment(FDI)
References
[1] Revenue Management Through Dynamic Cross Selling in E-Commerce Retailing Operations Research 54(5 – Serguei Netessine,See Profile, Available from Serguei Netessine, Serguei Netessine -1997
[2] Insurance Databases for Effective Prediction of Customer Preferences over Life Insurance Products – S. Balaji, S. K. Srivatsa, PhD Senior Professor - A LITERATURE REVIEW – Olof Wahlberg, Christer Strandberg, Karl W. Sandberg
[3] Unsupervised Learning in Large Datasets for Intelligent Decision
Making – S. Balaji,Dr. S. K. Srivatsa.
[4] important influences on classification accuracy – Mohammad Saad Al-Ahmadi, Peter A. Rosen, Rick L. Wilson
[5] Decision Support System for A Customer Relationship Management Case Study – Ozge Kart, Alp Kut, Vladimir Radevski
[6] Proceedings of the 40th Hawaii International Conference on System Sciences- 2007 Synergies of Data Mining and Operations Research –Stephan Meisel, Dirk Chr Mattfeld
[7] Stakeholder Perceptions Regarding eCRM: A Franchise Case Study –unknown authors
[8] Xin Zhao, Yi Wang, and Hongwang Cha. “A New Prediction Model of Customer Churn Based on PCA Analysis”. In: Information Science and Engineering (ICISE), 2009 1st International Conference on. IEEE. 2009, pp. 4657–4661.
[9] Qing-an Cui et al. “Using PCA and ANN to identify significant factors and modeling customer satisfaction for the complex service processes”.In: Industrial Engineering and Engineering Management (IE&EM),2011 IEEE 18Th International Conference on. IEEE. 2011, pp. 1800–1804.
[10] Volodymyr Kuleshov. “Fast algorithms for sparse principal component analysis based on Rayleigh quotient iteration”. In: International Conference on Machine Learning. 2013, pp. 1418–1425.
[11] Zhexue Huang. “Extensions to the k-means algorithm for clustering large data sets with categorical values”. In: Data mining and knowledge discovery 2.3 (1998), pp. 283–304.
[12] Charu C. Aggarwal, Alexander Hinneburg, and Daniel A. Keim. “On the Surprising Behavior of Distance Metrics in High Dimensional Space”. In: Lecture Notes in Computer Science. Springer, 2001, pp. 420–434.
[13] James Bennett, Stan Lanning, et al. “The netflix prize”. In: Proceedings of KDD cup and workshop. Vol. 2007. New York, NY, USA. 2007, p. 35.
[14] James Bennett. “The Cinematch system: Operation, scale coverage, accuracy impact”. In: Summer School on the Present and Future of Recommender Systems (2006).
[15] Yehuda Koren. “The bellkor solution to the netflix grand prize”. In:Netflix prize documentation 81 (2009), pp. 1–10.
[16] Andreas T ̈oscher, Michael Jahrer, and Robert M Bell. “The bigchaos solution to the netflix grand prize”. In: Netflix prize documentation (2009), pp. 1–52.
[17] Martin Piotte and Martin Chabbert. “The pragmatic theory solution to the netflix grand prize”. In: Netflix prize documentation (2009).
[18] A Literature Review on Text Mining Techniques and Methods,IJCSE,Vol.06,Issue.02,pp.96-99,Mar-2018.
[19] Meteric for evaluating availability of an information system: A Quantitative approach based on component dependency,IJNSA,Vol-9,number-2,Mar-2017.